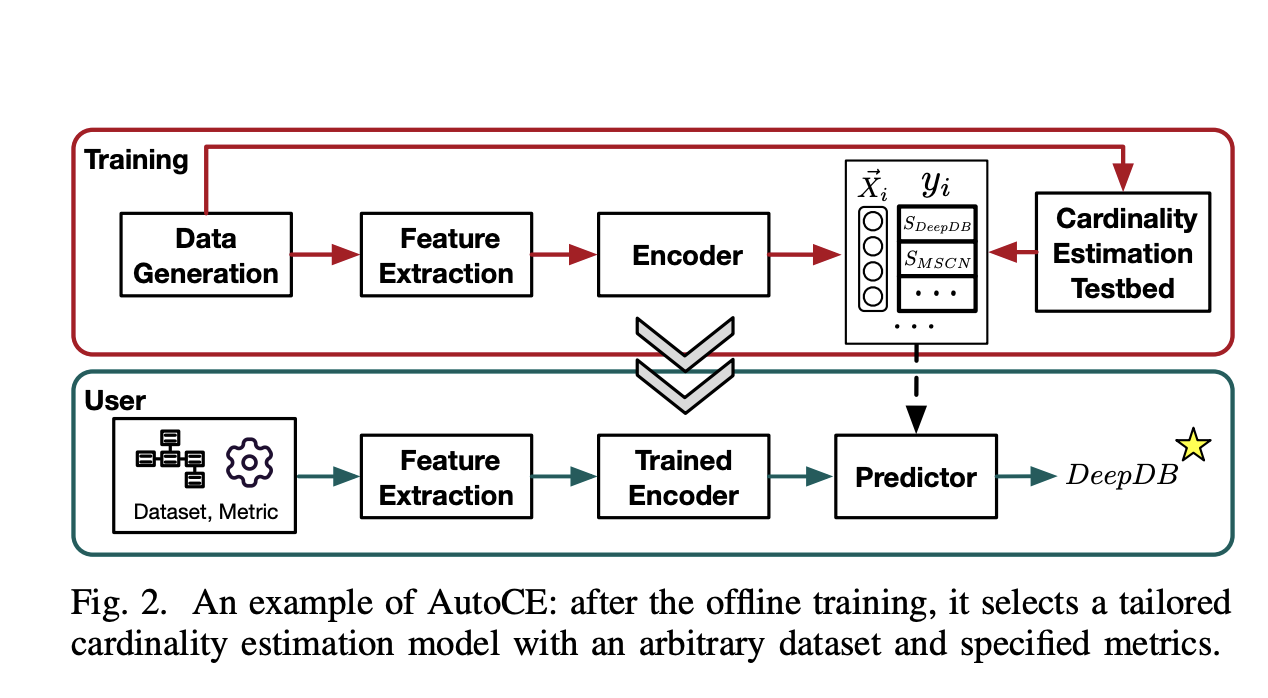
Practical Solutions and Value of Cardinality Estimation in Databases
Importance of Cardinality Estimation (CE) in Database Tasks
CE is crucial for tasks like query planning, cost estimation, and optimization in databases. Accurate CE ensures efficient query execution.
Benefits of Machine Learning in CE
Using Machine Learning enhances CE accuracy and reduces processing time, leading to better database management systems.
Challenges in CE and Existing Methods
Diverse datasets pose challenges in CE. Two main methods, query-driven and data-driven models, exist but struggle to generalize performance.
Introducing AutoCE for Intelligent Model Selection
AutoCE by researchers selects the best CE model automatically based on dataset features. It improves performance significantly without exhaustive training.
AutoCE’s Core Technology and Performance
AutoCE extracts dataset features, trains a graph encoder, and uses an incremental learning strategy for improved predictions. It outperforms traditional models in accuracy and efficiency metrics.
Key Takeaways from AutoCE Research
AutoCE enhances efficiency, accuracy, and reduces latency in database systems. It adapts to varying dataset characteristics and integrates well into PostgreSQL v13.1.
Conclusion
AutoCE offers a solution to CE model selection using advanced deep-learning techniques, transforming database query optimization and improving accuracy and efficiency in data-intensive applications.
AI Solutions for Your Company
Utilize AutoCE to stay competitive and redefine your work processes with AI. Identify automation opportunities, define KPIs, select suitable AI tools, and implement gradually for successful integration. Connect with us for AI KPI management advice and insights on leveraging AI for sales processes and customer engagement.