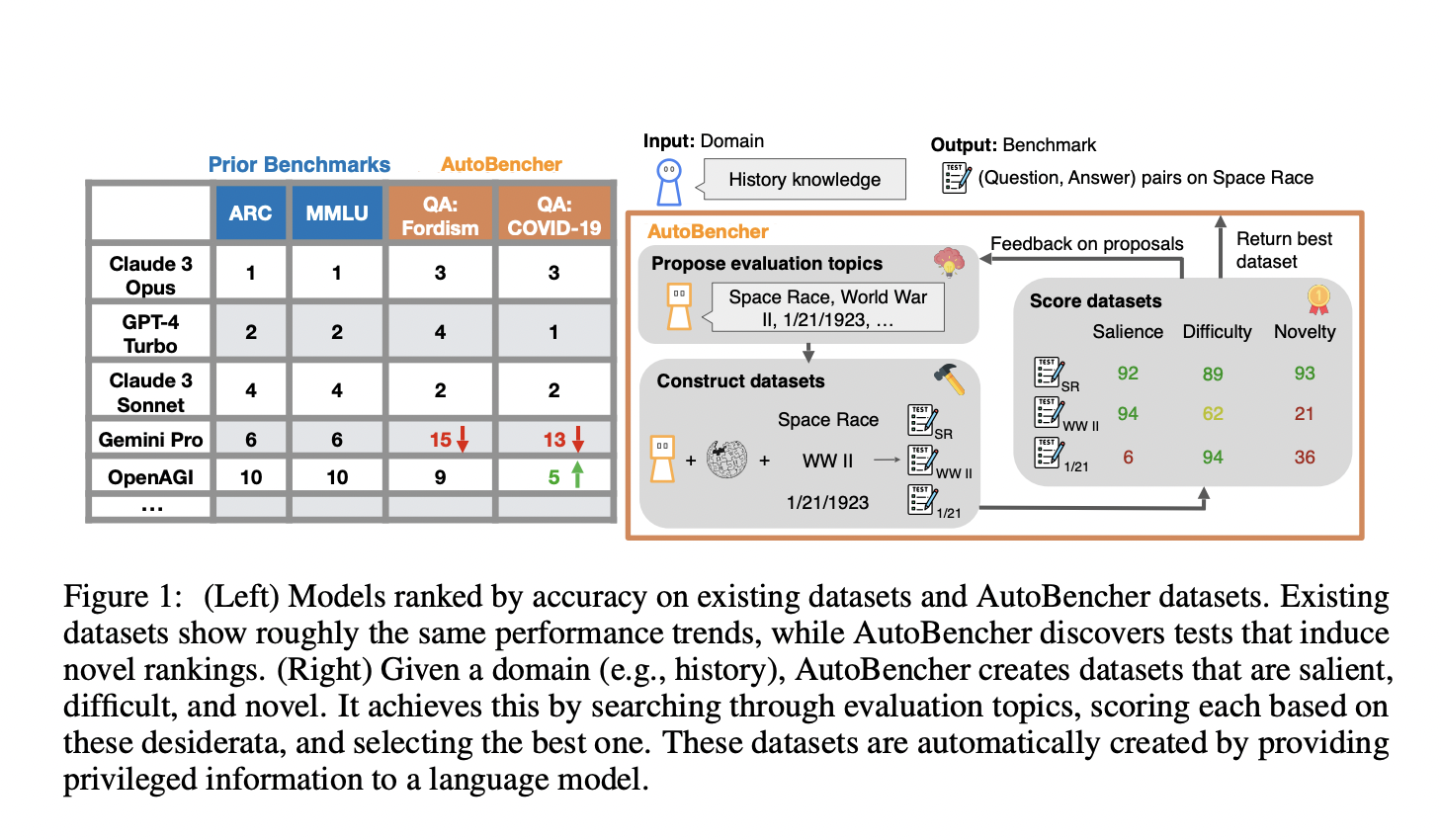
The Challenge of Evaluating Language Models
This paper addresses the challenge of effectively evaluating language models (LMs). Evaluation is crucial for assessing model capabilities, tracking scientific progress, and informing model selection. Traditional benchmarks often fail to highlight novel performance trends and are sometimes too easy for advanced models, providing little room for growth. The research identifies three key desiderata that existing benchmarks often lack: salience (testing practically important capabilities), novelty (revealing previously unknown performance trends), and difficulty (posing challenges for existing models).
Introducing AutoBencher: A New Solution
The researchers of this paper propose a new tool, AutoBencher, which automatically generates datasets that fulfill the three desiderata: salience, novelty, and difficulty. AutoBencher uses a language model to search for and construct datasets from privileged information sources. This approach allows creation of more challenging and insightful benchmarks compared to existing ones.
How AutoBencher Works
AutoBencher operates by leveraging a language model to propose evaluation topics within a broad domain (e.g., history) and constructing small datasets for each topic using reliable sources like Wikipedia. The tool evaluates each dataset based on its salience, novelty, and difficulty, selecting the best ones for inclusion in the benchmark. This iterative and adaptive process allows the tool to refine its dataset generation to maximize the desired properties continuously.
The Impact of AutoBencher
The results show that AutoBencher-created benchmarks are, on average, 27% more novel and 22% more difficult than existing human-constructed benchmarks. The tool has been used to create datasets across various domains, including math, history, science, economics, and multilingualism, revealing new trends and gaps in model performance.
AutoBencher: A Metrics-Driven AI Approach Towards Constructing New Datasets for Language Models
The problem of effectively evaluating language models is critical for guiding their development and assessing their capabilities. AutoBencher offers a promising solution by automating the creation of salient, novel, and difficult benchmarks, thereby providing a more comprehensive and challenging evaluation framework for language models.
Get in Touch
If you want to evolve your company with AI, stay competitive, and use AutoBencher, connect with us at hello@itinai.com. For continuous insights into leveraging AI, stay tuned on our Telegram t.me/itinainews or Twitter @itinaicom.