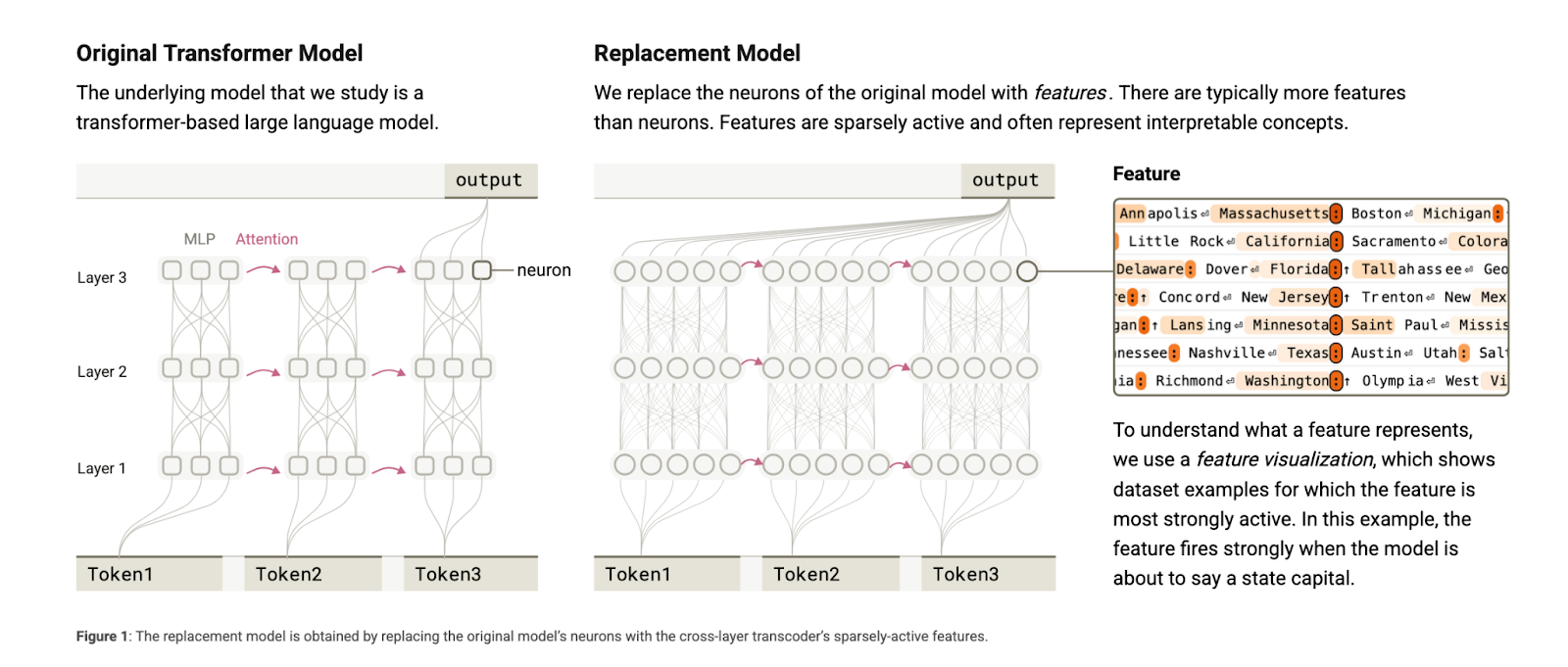
Understanding Attribution Graphs: A New Approach to AI Interpretability
Introduction
In recent developments in artificial intelligence, researchers from Anthropic have introduced a novel technique known as attribution graphs. This method aims to enhance our understanding of how large language models (LLMs), such as Claude 3.5 Haiku, derive their outputs. As AI systems are increasingly utilized in critical applications, it is essential to comprehend their internal reasoning processes.
The Challenge of AI Interpretability
One of the primary challenges in AI is deciphering the internal decision-making processes of models, which operate using complex layers and vast numbers of parameters. Without insight into these mechanisms, it becomes difficult to trust or troubleshoot AI performance, especially in tasks requiring logical reasoning or factual accuracy. Traditional interpretability methods, such as attention maps and feature attribution, provide limited visibility into model behavior, often overlooking the intricate steps involved in generating outputs.
Limitations of Existing Methods
- Partial Insights: Current tools often highlight which input elements contribute to an output but fail to trace the complete reasoning chain.
- Surface-Level Analysis: Many existing methods focus on immediate behaviors rather than deeper computational processes.
- Need for Structure: There is a demand for more organized techniques to analyze the internal logic of models over multiple steps.
Introducing Attribution Graphs
To address these challenges, Anthropic has developed attribution graphs, which allow researchers to track the flow of information within a model during a single processing cycle. This technique helps uncover intermediate reasoning steps that are not evident from the final outputs alone.
Methodology and Application
Attribution graphs were applied to Claude 3.5 Haiku, a lightweight language model released in October 2024. The methodology involves identifying key features activated by specific inputs and tracing their influence on the final results. For instance, when tasked with solving a riddle, the model strategically selects rhyming words before generating the text, demonstrating planning capabilities.
Case Studies and Findings
The application of attribution graphs has revealed several advanced behaviors in Claude 3.5 Haiku:
- Poetry Composition: The model exhibits anticipatory reasoning by pre-selecting rhyming words, enhancing its poetic outputs.
- Multi-Hop Reasoning: It forms internal representations, such as linking Dallas to Texas, before arriving at the correct answer, Austin.
- Medical Diagnosis: The model generates internal diagnoses in medical queries, which inform subsequent questions.
These insights indicate that the model can perform logical deductions and set internal goals independently of explicit instructions.
Business Implications
The introduction of attribution graphs represents a significant advancement in AI interpretability, providing businesses with the tools to better understand and trust AI systems. Here are practical steps companies can take to leverage this technology:
- Identify Automation Opportunities: Look for processes that can be automated with AI to enhance efficiency.
- Monitor Key Performance Indicators (KPIs): Establish metrics to evaluate the effectiveness of AI implementations.
- Select Customizable Tools: Choose AI solutions that can be tailored to meet your specific business needs.
- Start Small: Begin with a pilot project, assess its impact, and gradually expand AI usage based on data-driven insights.
Conclusion
Attribution graphs offer a groundbreaking approach to understanding the internal workings of AI models like Claude 3.5 Haiku. By revealing the layered reasoning processes involved in generating outputs, this method enhances the transparency and reliability of AI systems. As businesses explore AI integration, utilizing tools like attribution graphs will be vital for fostering trust and ensuring responsible deployment of advanced technologies.