The text discusses the proposal of the asymmetric certified robustness problem for deep learning classifiers, which addresses the vulnerability of these classifiers to adversarial examples. It introduces feature-convex classifiers as a solution to this problem, providing closed-form and deterministic certified radii for inputs. The text also highlights the theoretical promise of input-convex classifiers achieving perfect training accuracy. Overall, the aim is to inspire novel architectures that can be certifiable in real-world adversarial scenarios.
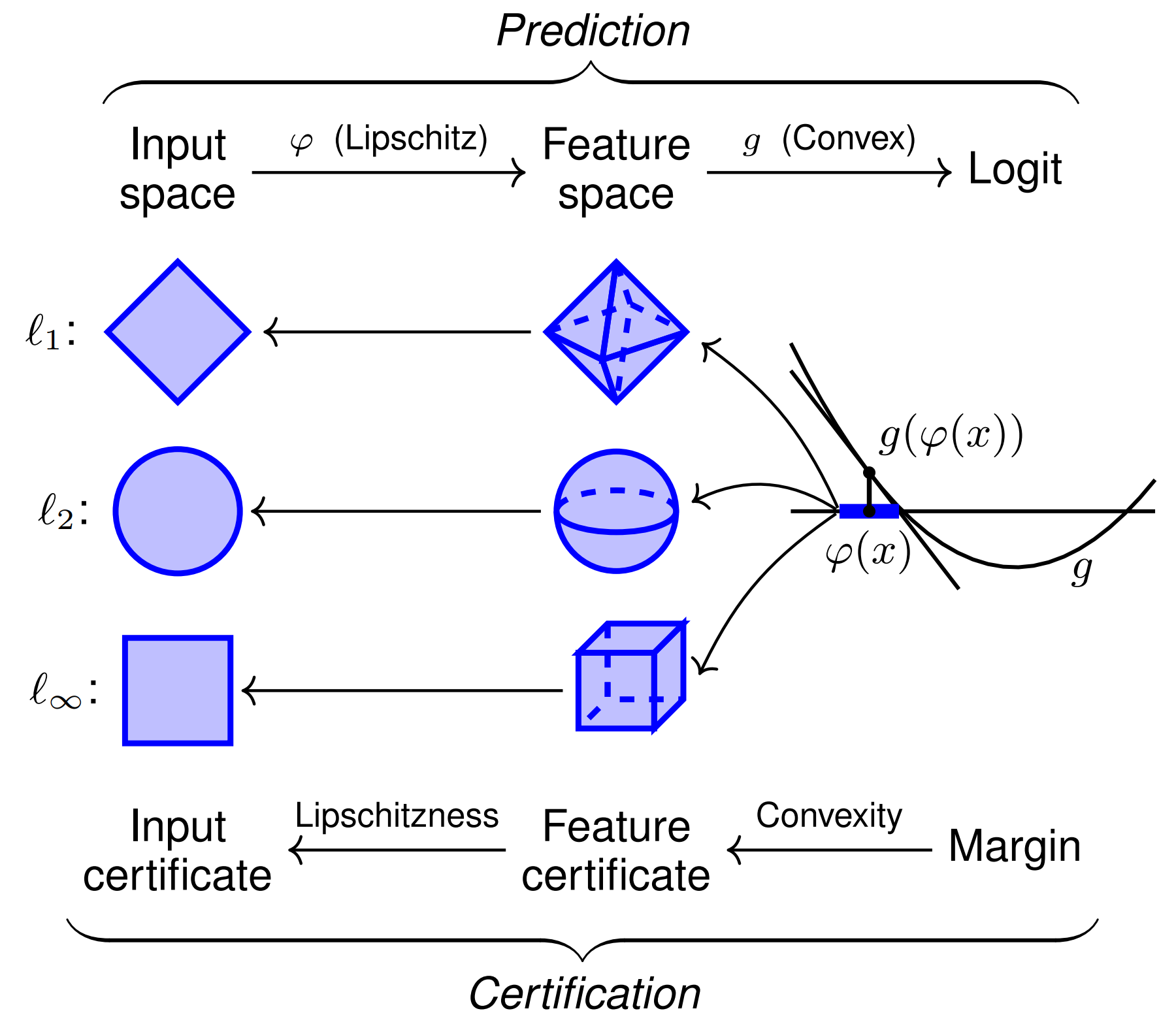
Asymmetric Certified Robustness via Feature-Convex Neural Networks
TLDR:
We propose a solution to the problem of adversarial attacks on deep learning classifiers. Our approach, called asymmetric certified robustness, focuses on providing certified robustness for one specific class, reflecting real-world adversarial scenarios. We introduce feature-convex classifiers, which produce fast and deterministic certified radii on the order of milliseconds.
Introduction:
Deep learning classifiers are vulnerable to adversarial examples, which are small modifications to inputs that fool the classifiers into misclassifying them. This poses a significant problem for safety-critical processes that rely on machine learning. We focus on certifiably robust classifiers, which provide a mathematical guarantee that their predictions will remain constant within a certain range of input perturbations.
The Asymmetric Certified Robustness Problem:
Current certified robustness methods provide certificates for inputs belonging to any class, which is unnecessarily broad for many real-world adversarial applications. We propose the problem of asymmetric certified robustness, which aims to provide certifiably robust predictions for inputs in a specific sensitive class while maintaining high accuracy for all other inputs. This is relevant in various applications such as email filtering, malware detection, and financial fraud detection.
Feature-Convex Classifiers:
We propose feature-convex neural networks to address the asymmetric robustness problem. This architecture combines a Lipschitz-continuous feature map with a learned Input-Convex Neural Network (ICNN). The ICNN enforces convexity from the input to the output, allowing for nonconvex decision regions. Feature-convex classifiers enable fast computation of certified radii for different norm types.
Practical Value:
Our feature-convex classifiers provide fast and deterministic certified radii for any norm type. These certificates are computable in milliseconds and scale well with network size. Compared to other methods, our approach achieves competitive certificates with significantly less runtime. Our theoretical work also suggests untapped potential in ICNNs, even without a feature map.
Conclusion:
Asymmetric certified robustness and feature-convex classifiers offer practical solutions to the problem of adversarial attacks on deep learning classifiers. Our approach provides fast and deterministic certified radii for any norm type, ensuring the reliability of safety-critical processes. For more details, please refer to our NeurIPS paper and codebase.
If you’re looking to evolve your company with AI and stay competitive, consider leveraging Asymmetric Certified Robustness via Feature-Convex Neural Networks. Discover how AI can redefine your way of work and automate key customer interactions. Connect with us at hello@itinai.com for AI KPI management advice and stay updated on our Telegram channel t.me/itinainews or Twitter @itinaicom for continuous insights into leveraging AI.
Spotlight on a Practical AI Solution: AI Sales Bot
Consider our AI Sales Bot from itinai.com/aisalesbot, designed to automate customer engagement and manage interactions across all customer journey stages. Discover how AI can redefine your sales processes and customer engagement. Explore solutions at itinai.com.