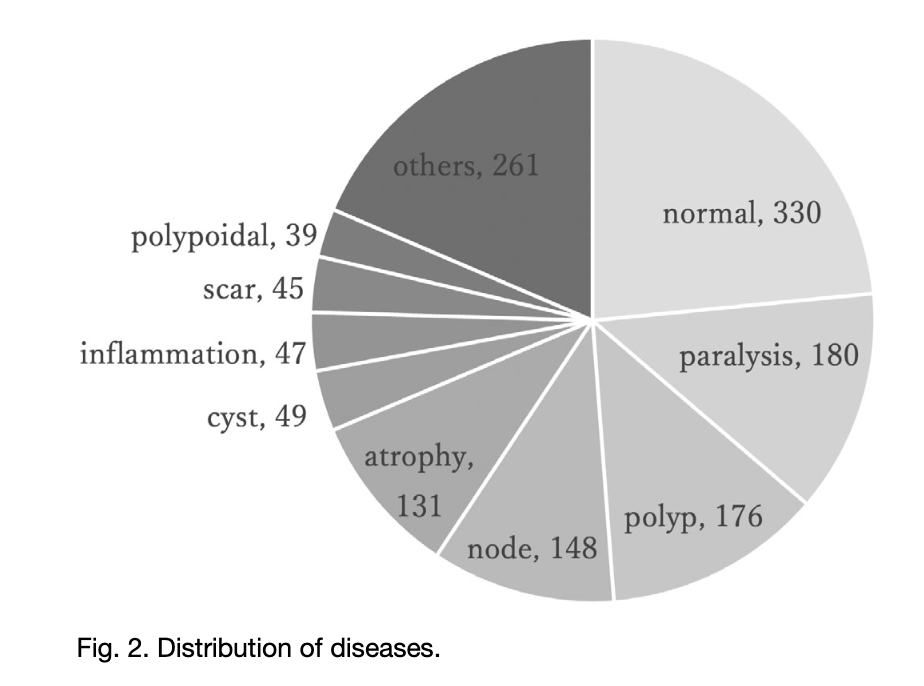
Practical Solutions for Assessing Noise Impact on Machine Learning Models for Voice Disorder Evaluation
Challenges in Pathological Voice Classification
Traditional methods for classifying pathological voices are time-consuming and inconsistent. Deep learning techniques offer advantages by automatically learning relevant features from raw audio data, capturing complex patterns and nuances indicative of specific pathological conditions.
Impact of Noise on Model Accuracy
Noise significantly impacts the accuracy of machine learning models used for evaluating voice disorders. Preprocessing techniques like noise reduction and signal enhancement are often employed, but may not always be sufficient to eliminate the effects of noise on classification performance.
Study on Noise Impact
A recent study in The Laryngoscope assessed the impact of background noise on machine learning models used for evaluating the GRBAS scale in voice disorder assessments. The study highlighted the significance of noise as a challenge in applying machine learning models to real-world scenarios like examination rooms.
Findings and Recommendations
The study found that background noise severely affects the model’s accuracy and performance metrics. It suggests incorporating noise-resilient techniques such as data augmentation and noise reduction to improve model robustness. Future research should focus on developing noise-tolerant methods to enhance the model’s resilience in real-world conditions.
Value of AI in Business
AI can redefine work processes, automate tasks, and improve customer engagement. It can help identify automation opportunities, define measurable KPIs, select suitable AI solutions, and implement AI gradually for business impact.
Connect with Us
For AI KPI management advice and continuous insights into leveraging AI, connect with us at hello@itinai.com. Follow us on Telegram t.me/itinainews or Twitter @itinaicom for more insights.