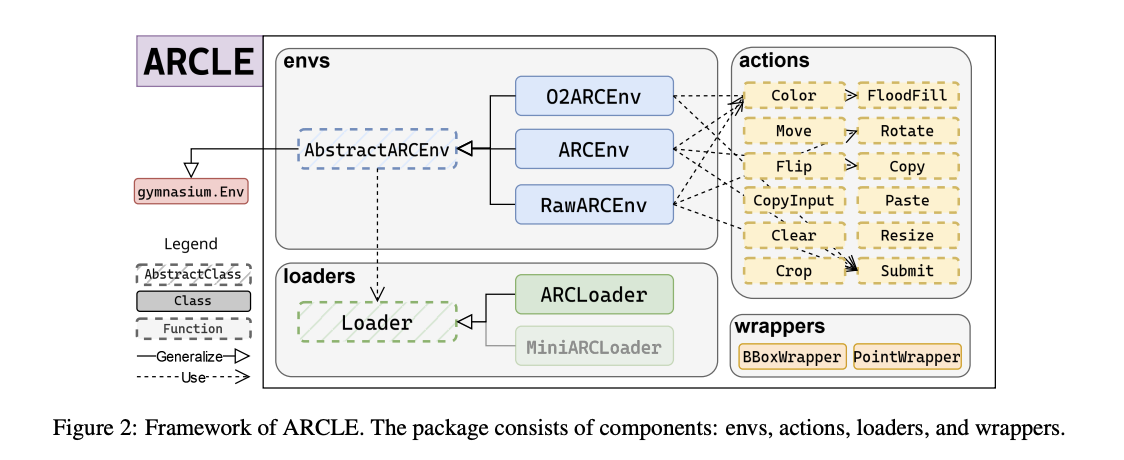
Reinforcement Learning for Abstract Reasoning Challenges
Practical Solutions and Value
Reinforcement learning (RL) trains agents to make sequential decisions by rewarding desirable actions, applicable in robotics, gaming, and autonomous systems. RL allows machines to learn from interactions, adjusting actions to maximize rewards over time.
One significant challenge in RL is addressing tasks requiring high levels of abstraction and reasoning, such as those presented by the Abstraction and Reasoning Corpus (ARC). Traditional approaches have struggled with the complexity of ARC tasks, leading to the exploration of alternative methods like reinforcement learning.
ARCLE (ARC Learning Environment) is a specialized RL environment designed to facilitate research on ARC. It enables researchers to train agents using reinforcement learning techniques tailored for the complex tasks presented by ARC.
Agents trained within ARCLE using proximal policy optimization (PPO) showed marked improvements in task performance, achieving a high success rate in solving ARC tasks. The introduction of auxiliary losses led to a significant increase in cumulative rewards and success rates, outperforming agents trained without these methods.
The research underscores the potential of ARCLE in advancing RL strategies for abstract reasoning tasks, paving the way for exploring advanced RL techniques and promising to enhance AI’s reasoning and abstraction capabilities further.
If you want to evolve your company with AI, stay competitive, and use ARCLE to redefine your way of work. Connect with us at hello@itinai.com for AI KPI management advice and continuous insights into leveraging AI.
Discover how AI can redefine your sales processes and customer engagement. Explore solutions at itinai.com.