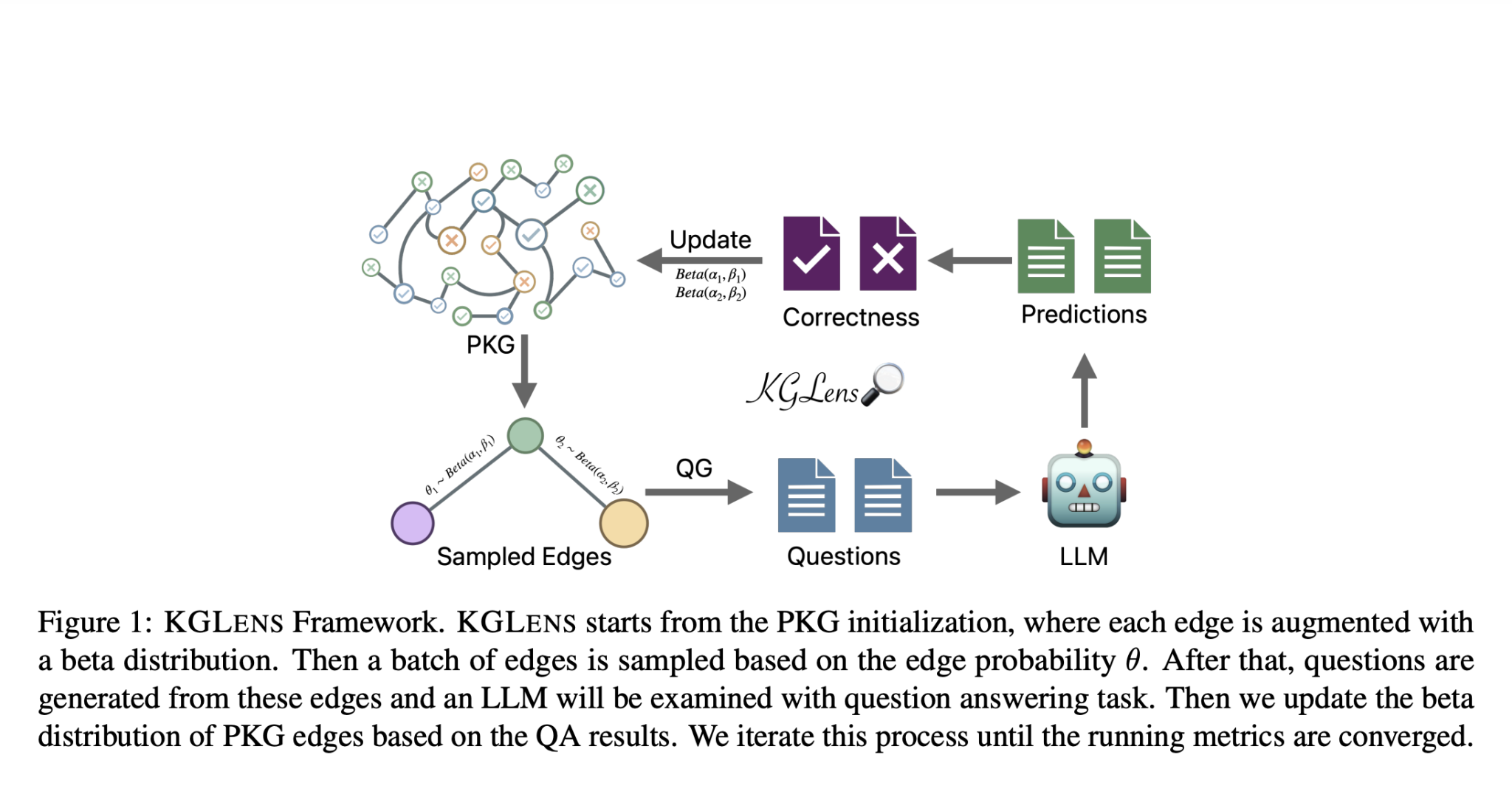
Challenges in Evaluating Large Language Models (LLMs)
Concerns with Factualness and Evaluation Methods
Large Language Models (LLMs) are versatile but can produce nonfactual, outdated information, posing reliability concerns. Current evaluation methods, such as fact-checking and fact-QA, face challenges in assessing factualness and scaling up evaluation data.
Limitations of Existing Evaluation Approaches
Existing attempts to evaluate LLMs’ knowledge face challenges like data leakage, static content, and limited metrics. Current approaches focus on accuracy over reliability, failing to address LLMs’ inconsistent responses to the same fact.
Introduction of KGLENS Framework
Researchers from Apple introduced KGLENS, an innovative knowledge probing framework that efficiently measures knowledge alignment between knowledge graphs (KGs) and LLMs. KGLENS identifies LLMs’ knowledge blind spots and features a graph-guided question generator to reduce answer ambiguity.
KGLENS: A Breakthrough in Evaluating LLMs
Efficient Knowledge Probing
KGLENS employs a Thompson sampling-inspired method with a parameterized knowledge graph (PKG) to probe LLMs efficiently. It features a graph-guided question generator that converts KG edges into natural language questions using GPT-4.
Answer Verification and Evaluation
KGLENS instructs LLMs to generate specific response formats and employs GPT-4 to check the correctness of responses for Wh-questions. The framework’s efficiency is evaluated through various sampling methods, demonstrating its effectiveness in identifying LLMs’ knowledge blind spots across diverse topics and relationships.
Performance Comparison
KGLENS evaluation across various LLMs reveals that the GPT-4 family consistently outperforms other models. It provides insights into different error types and model behaviors, demonstrating the varying capabilities of LLMs in handling diverse knowledge domains and difficulty levels.
Impact and Future Availability
Advantages and Availability
KGLENS introduces an efficient method for evaluating factual knowledge in LLMs and outperforms existing methods in revealing knowledge blind spots. Human evaluation confirms its effectiveness, achieving 95.7% accuracy. KGLENS and its assessment of KGs will be made available to the research community, fostering collaboration.
Business Implications
For businesses, KGLENS facilitates the development of more reliable AI systems, enhancing user experiences and improving model knowledge. It represents a significant advancement in creating more accurate and dependable AI applications.
AI Solutions for Business Transformation
AI Implementation Guidance
Discover how AI can redefine your way of work by identifying automation opportunities, defining KPIs, selecting AI solutions, and implementing gradually. Connect with us at hello@itinai.com for AI KPI management advice and continuous insights into leveraging AI.
Enhancing Sales Processes and Customer Engagement
Explore AI solutions at itinai.com to redefine your sales processes and customer engagement, leveraging the power of AI to stay competitive and evolve your company.