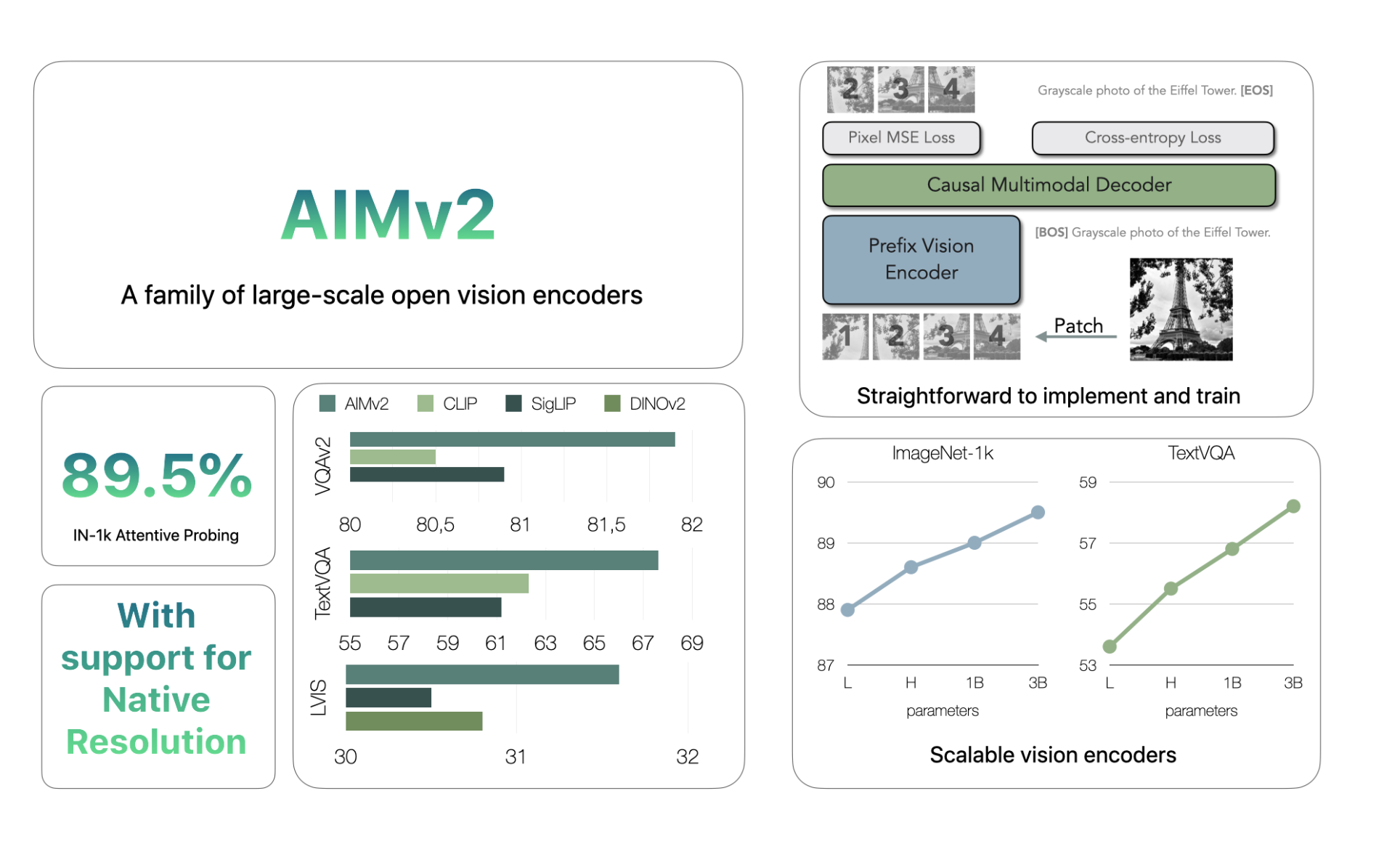
Vision Models and Their Evolution
Vision models have greatly improved over time, responding to the challenges of previous versions. Researchers in computer vision often struggle with making models that are both complex and adaptable. Many current models find it hard to manage various visual tasks or adapt to new datasets effectively. Previous large-scale vision encoders relied on contrastive learning, which, while successful, has issues with scalability and efficiency. There is still a need for a strong model that can work with different types of data, like images and text, without losing performance or needing excessive data filtering.
AIMv2: A New Approach
Apple has addressed these challenges with AIMv2, a new set of vision encoders designed for better multimodal understanding and object recognition. AIMv2 introduces an autoregressive decoder, enhancing its ability to generate both image patches and text tokens. The AIMv2 family includes 19 models with sizes ranging from 300M to 2.7B parameters, and offers resolutions of 224, 336, and 448 pixels. This variety helps accommodate different applications, from small to large-scale tasks.
Key Features of AIMv2
- Multimodal Autoregressive Pre-training: AIMv2 combines a Vision Transformer (ViT) encoder with a causal multimodal decoder, allowing for more effective training.
- Enhanced Training Efficiency: The setup simplifies training and scales easily, without needing large batch sizes.
- Improved Learning: AIMv2 can learn from both images and text more effectively, increasing its performance.
Performance and Scalability
AIMv2 has outperformed several leading models in multimodal understanding benchmarks. For instance, the AIMv2-3B model achieved an impressive 89.5% accuracy on the ImageNet dataset. Its performance improves with larger datasets and models, making it a flexible choice for various applications. Additionally, AIMv2 integrates smoothly with tools like Hugging Face Transformers, simplifying implementation.
Conclusion
AIMv2 marks significant progress in vision encoder technology, focusing on ease of training and versatility for multimodal tasks. It delivers strong results across various benchmarks, including object recognition. The autoregressive methods used enable enhanced supervision, resulting in robust and adaptable models. AIMv2’s availability on platforms like Hugging Face allows developers to easily explore advanced vision models. This release sets a new benchmark for visual encoders, ready to tackle the challenges of real-world multimodal understanding.
Get Involved
Check out the AIMv2 paper and models on Hugging Face. Follow us on Twitter, join our Telegram Channel, and connect with us on LinkedIn. If you appreciate our work, subscribe to our newsletter and join our community of over 55k on the ML SubReddit.
Upcoming Event
[FREE AI VIRTUAL CONFERENCE] Join SmallCon: A Free Virtual GenAI Conference featuring Meta, Mistral, Salesforce, and more on Dec 11th. Learn how to effectively build with small models from industry leaders.
Transform Your Business with AI
To enhance your company with AI and stay competitive:
- Identify Automation Opportunities: Find key customer interaction points for AI benefits.
- Define KPIs: Ensure measurable impacts from your AI initiatives.
- Select an AI Solution: Choose tools that fit your needs and allow customization.
- Implement Gradually: Start with a pilot project, gather data, and expand use wisely.
For advice on AI KPI management, connect with us at hello@itinai.com. For ongoing insights into leveraging AI, follow us on Telegram at t.me/itinainews or on Twitter at @itinaicom.
Explore how AI can revolutionize your sales processes and customer engagement at itinai.com.