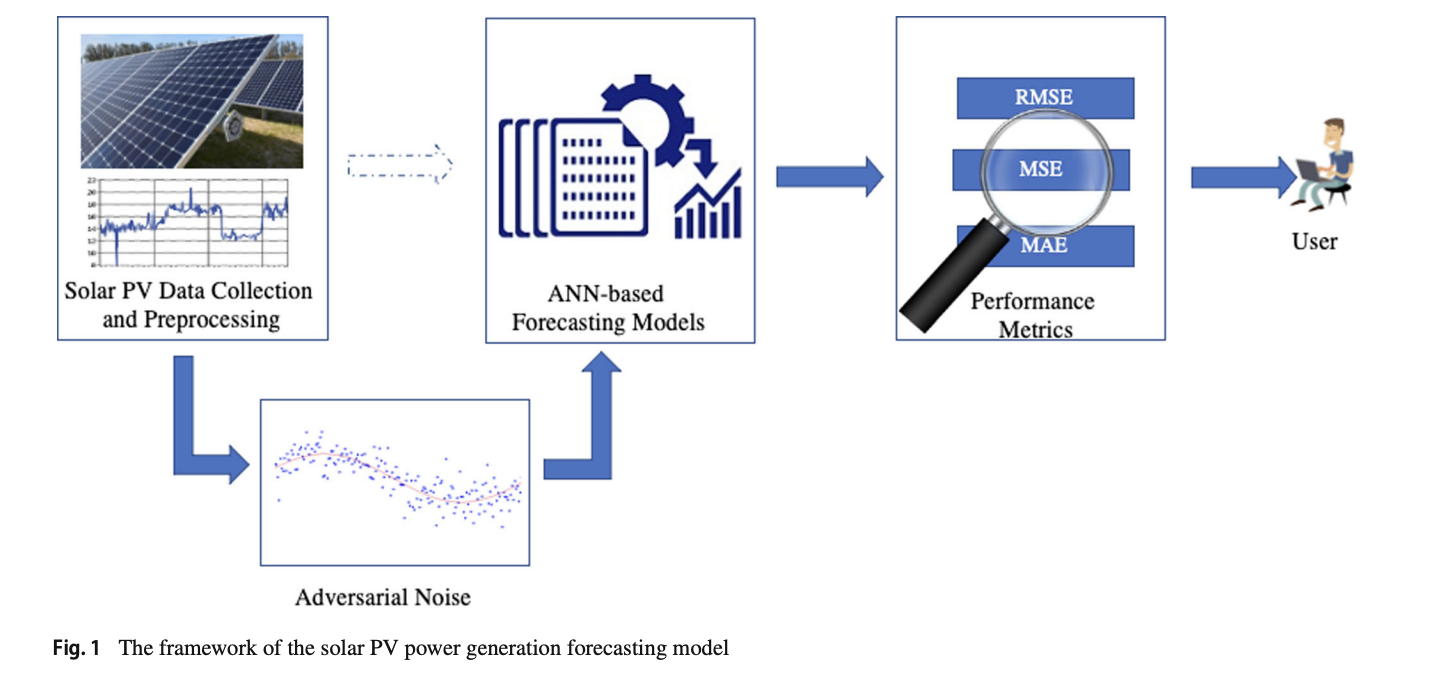
Understanding Photovoltaic Energy and AI Solutions
Photovoltaic energy uses solar panels to convert sunlight into electricity, playing a crucial role in the transition to renewable energy. Deep learning helps optimize energy production, predict weather changes, and enhance solar system efficiency, leading to smarter energy management.
Current Prediction Techniques
There are various ways to forecast photovoltaic power generation. Traditional methods like linear regression and decision trees are fast but often lack accuracy. More advanced techniques, such as artificial neural networks (ANNs), can uncover complex data patterns. Additionally, deep learning methods, including convolutional networks (CNNs) and long short-term memory (LSTM) models, excel in analyzing time and weather data. However, these models are vulnerable to adversarial attacks, which can threaten the reliability of forecasts in smart grids.
Innovative Research Breakthrough
A recent study by a US-Norwegian research team introduces a new approach to photovoltaic energy forecasting. Utilizing ANN and examining adversarial machine learning attacks, this research could greatly enhance the accuracy of solar energy predictions. By adapting the FGSM attack to regression models, the study shows how minor data changes can mislead ANN solar forecasting models, improving efficiency.
Four-Step Forecasting Method
The proposed method consists of four key steps:
- Data Collection and Processing: Gather solar data from public sources, clean it by removing missing values, detecting anomalies, and normalizing it for better accuracy.
- Introducing Adversarial Noise: Use the FGSM method to add subtle noise to the data, aiming to challenge the forecasting model.
- Prediction with ANN: Train and test the ANN model on processed data, both with and without adversarial noise, to assess its performance.
- Performance Measurement: Evaluate the model using metrics like Mean Squared Error (MSE) and Root Mean Squared Error (RMSE) to understand the impact of adversarial attacks.
Experimental Study Insights
The study utilized an open-source dataset from the 2014 Global Energy Forecasting Competition, comprising hourly solar generation data and weather forecasts from April 2012 to July 2014. The ANN model achieved impressive metrics of RMSE at 0.0874 without attacks, but faced challenges under adversarial conditions, with RMSE rising to about 0.793. This highlights the importance of developing more robust forecasting strategies.
Conclusion and Future Directions
This research emphasizes significant progress in photovoltaic energy forecasting through artificial neural networks while revealing their susceptibility to adversarial attacks. It stresses the necessity for stronger forecasting models in smart grids, showcasing the relationship between machine learning and energy prediction. The findings pave the way for future advancements that prioritize both security and efficiency in renewable energy systems.
Check out the Paper. All credit goes to the researchers involved. Follow us on Twitter, join our Telegram Channel, and connect with our LinkedIn Group. If you appreciate our work, subscribe to our newsletter and join our 50k+ ML SubReddit.
Upcoming Event
Live Webinar – Oct 29, 2024: Discover the Best Platform for Serving Fine-Tuned Models: Predibase Inference Engine.
AI Solutions for Your Business
Transform your business with AI to stay competitive. Here’s how:
- Identify Automation Opportunities: Find key customer interaction points to enhance with AI.
- Define KPIs: Ensure your AI initiatives yield measurable business outcomes.
- Select an AI Solution: Choose tools that meet your specific needs and allow for customization.
- Implement Gradually: Start with a pilot program, gather data, and scale AI usage wisely.
For AI KPI management advice, contact us at hello@itinai.com. For ongoing insights, follow us on Telegram or Twitter.
Explore how AI can enhance your sales processes and customer engagement at itinai.com.