An Analytics Engineer is responsible for transforming raw data into a format that can be used by Data Analysts to create reports and dashboards. They bridge the gap between Data Engineers and Analysts, allowing Data Engineers to focus on data ingestion while Analysts focus on the business intelligence layer. The ultimate tool for Analytics Engineering is data build tool (dbt), which helps manage data models efficiently.
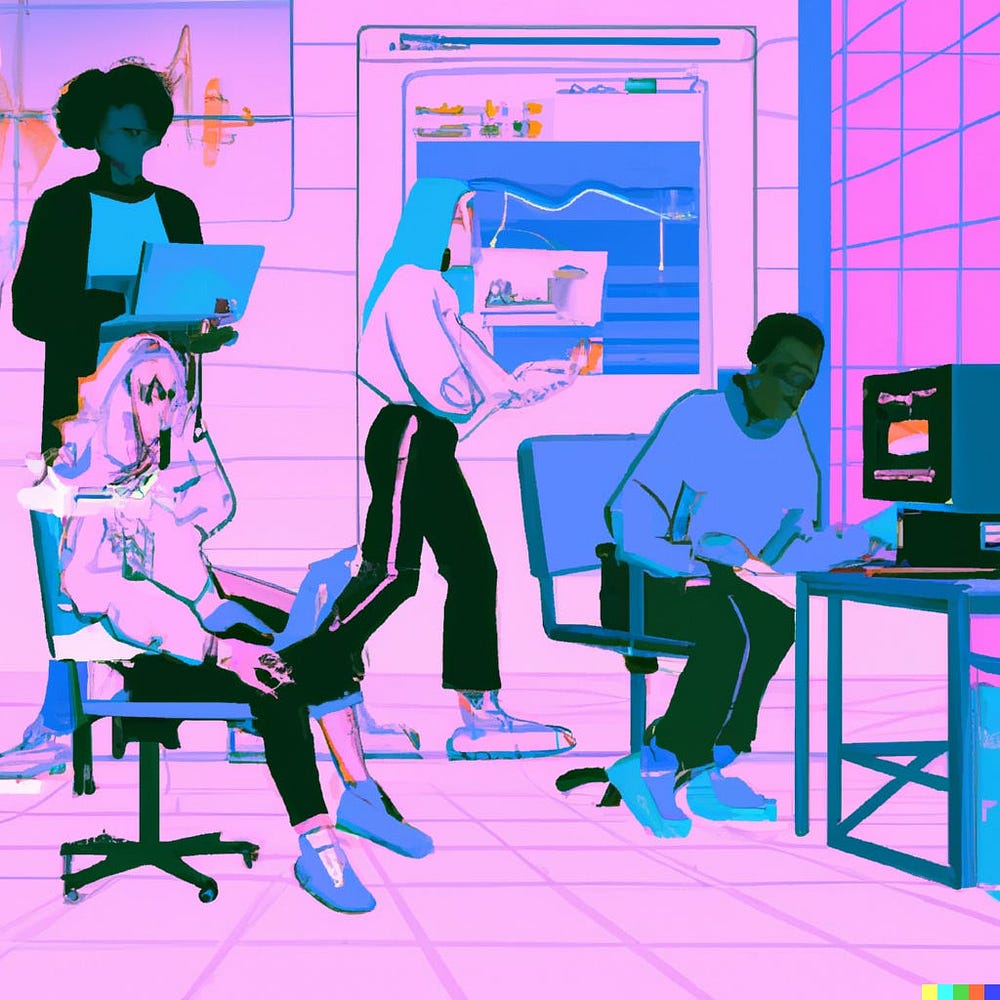
Who is an Analytics Engineer and what do they do?
An Analytics Engineer is a crucial role in data teams. They bridge the gap between Data Engineers and Data Analysts. Their main responsibility is to take raw data and apply transformations so that Data Analysts can use the transformed data to create dashboards and reports for business insights. This allows internal users to make data-informed decisions. Analytics Engineers allow Data Engineers to focus more on data ingestion and the overall data infrastructure.
Transitioning From ETL to ELT
Data teams traditionally used ETL (Extract, Transform, Load) processes to process and gain insights from data. This involved extracting data from a source, transforming it, and then loading it into a destination system. However, the emergence of cloud technologies has shifted the process towards ELT (Extract, Load, Transform). ELT allows data transformation to happen within the destination system, removing the need for a separate staging environment. The raw data also remains available for further transformations at any time.
Analytics Engineering and dbt
Analytics Engineering is an emerging field that focuses on speeding up the development of analytics products, improving data quality, and building trust in data. Analytics Engineers use tools like dbt (data build tool) to build and manage data models in a scalable and cost-effective manner. dbt automates the process of figuring out inter-dependencies between data models and provides functionality for data quality tests, freshness tests, and documentation. It sits within the ELT pipeline, performing transformations within the Data Warehouse where the raw data resides.
Practical AI Solutions for Middle Managers
If you want to evolve your company with AI and stay competitive, consider the practical AI solutions offered by itinai.com. AI can redefine your way of work by automating tasks, improving customer engagement, and providing data-driven insights. Follow these steps to leverage AI effectively:
1. Identify Automation Opportunities: Locate key customer interaction points that can benefit from AI.
2. Define KPIs: Ensure your AI endeavors have measurable impacts on business outcomes.
3. Select an AI Solution: Choose tools that align with your needs and provide customization.
4. Implement Gradually: Start with a pilot, gather data, and expand AI usage judiciously.
For AI KPI management advice, connect with us at hello@itinai.com. Stay tuned on our Telegram channel t.me/itinainews or Twitter @itinaicom for continuous insights into leveraging AI.
Spotlight on a Practical AI Solution: AI Sales Bot
Consider using the AI Sales Bot from itinai.com/aisalesbot to automate customer engagement 24/7 and manage interactions across all customer journey stages. This AI solution can redefine your sales processes and customer engagement. Explore more solutions at itinai.com.