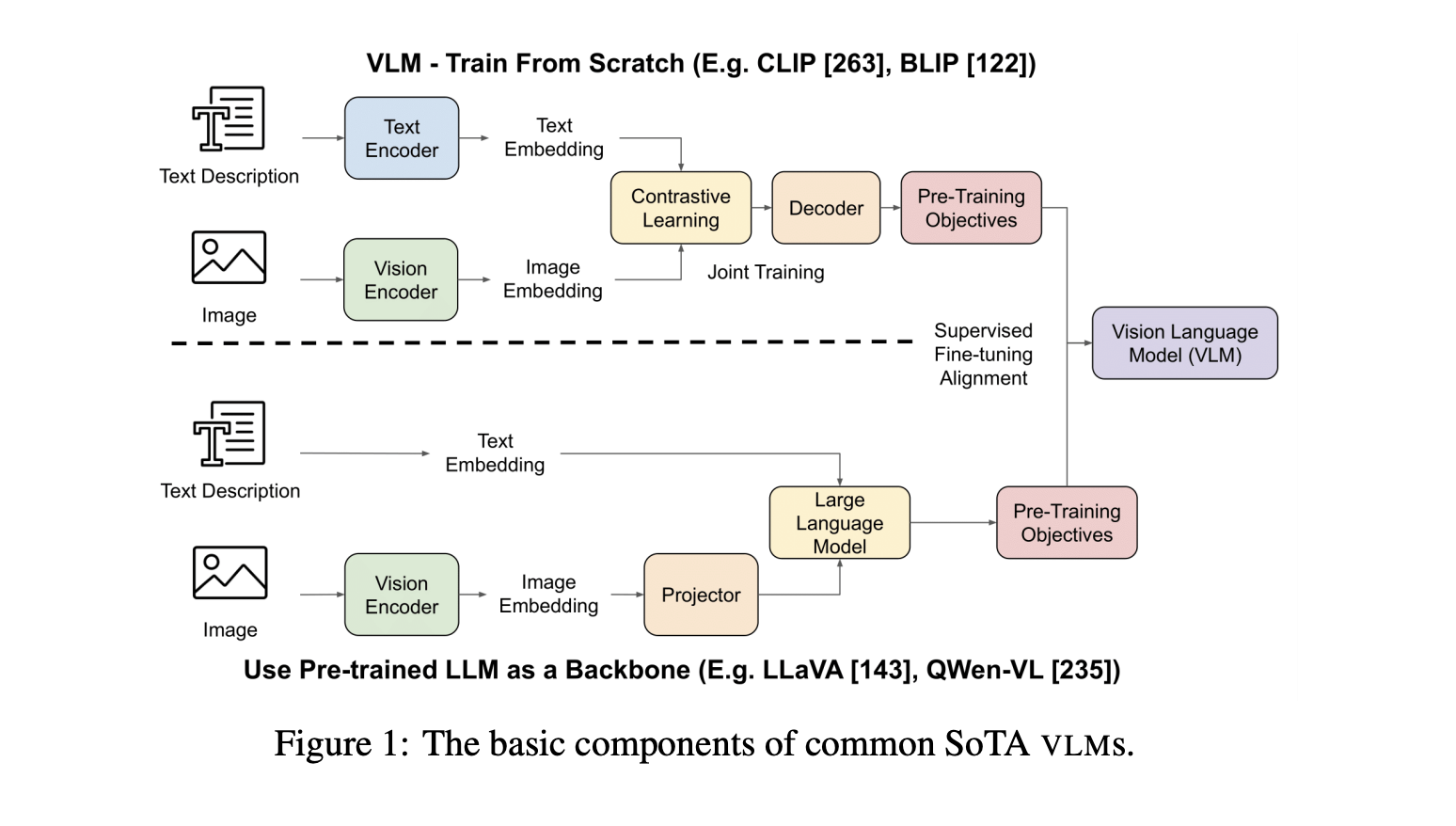
Understanding Vision Language Models (VLMs)
Vision Language Models (VLMs) represent a significant advancement in language model technology. They address the limitations of earlier models like LLama and GPT by integrating text, images, and videos. This integration enhances our understanding of visual and spatial relationships, offering a broader perspective.
Current Developments and Challenges
Researchers worldwide are actively tackling the challenges associated with VLMs. A recent survey from the University of Maryland and the University of Southern California highlights ongoing advancements in this field. This article provides insights into the evolution of VLMs over the past five years, covering their architecture, training methods, benchmarks, applications, and the challenges they face.
Key VLM Models
Some leading VLM models include:
- CLIP by OpenAI
- BLIP by Salesforce
- Flamingo by DeepMind
- Gemini
These models are at the forefront of supporting multimodal user interactions.
Structure of VLMs
VLMs consist of essential components:
- Vision Encoder
- Text Encoder
- Text Decoder
Cross-attention mechanisms help integrate information from different modalities, although they are not universally present. Developers often use pre-trained large language models to enhance VLM architecture, employing self-supervised techniques like masked image modeling and contrastive learning.
Benchmarking VLMs
VLMs are evaluated through various benchmarks that assess their capabilities, including:
- Visual text understanding
- Text-to-image generation
- Multimodal general intelligence
Common evaluation methods include answer matching, multiple-choice questions, and image/text similarity scores.
Applications of VLMs
VLMs have diverse applications, including:
- Virtual agents that interact with their environment
- Robotics for navigation and human-robot interaction
- Autonomous driving
Generative VLM models can also create visual content, enhancing user engagement.
Challenges Ahead
Despite their potential, VLMs face several challenges:
- Balancing flexibility and generalizability
- Addressing visual hallucinations and reliability concerns
- Ensuring fairness and safety due to biases in training data
- Developing efficient training methods with limited high-quality datasets
- Resolving contextual misalignments between modalities
Conclusion
This overview highlights the key aspects of Vision Language Models, including their architecture, innovations, and current challenges. For further insights, check out the Paper and GitHub Page. Follow us on Twitter and join our 75k+ ML SubReddit.
Transform Your Business with AI
To stay competitive, consider the following steps to leverage AI:
- Identify Automation Opportunities: Find customer interaction points that can benefit from AI.
- Define KPIs: Ensure measurable impacts on business outcomes.
- Select an AI Solution: Choose tools that meet your needs and offer customization.
- Implement Gradually: Start with a pilot project, gather data, and expand AI usage wisely.
For AI KPI management advice, connect with us at hello@itinai.com. For ongoing insights, follow us on Telegram or @itinaicom.
Discover how AI can transform your sales processes and customer engagement at itinai.com.