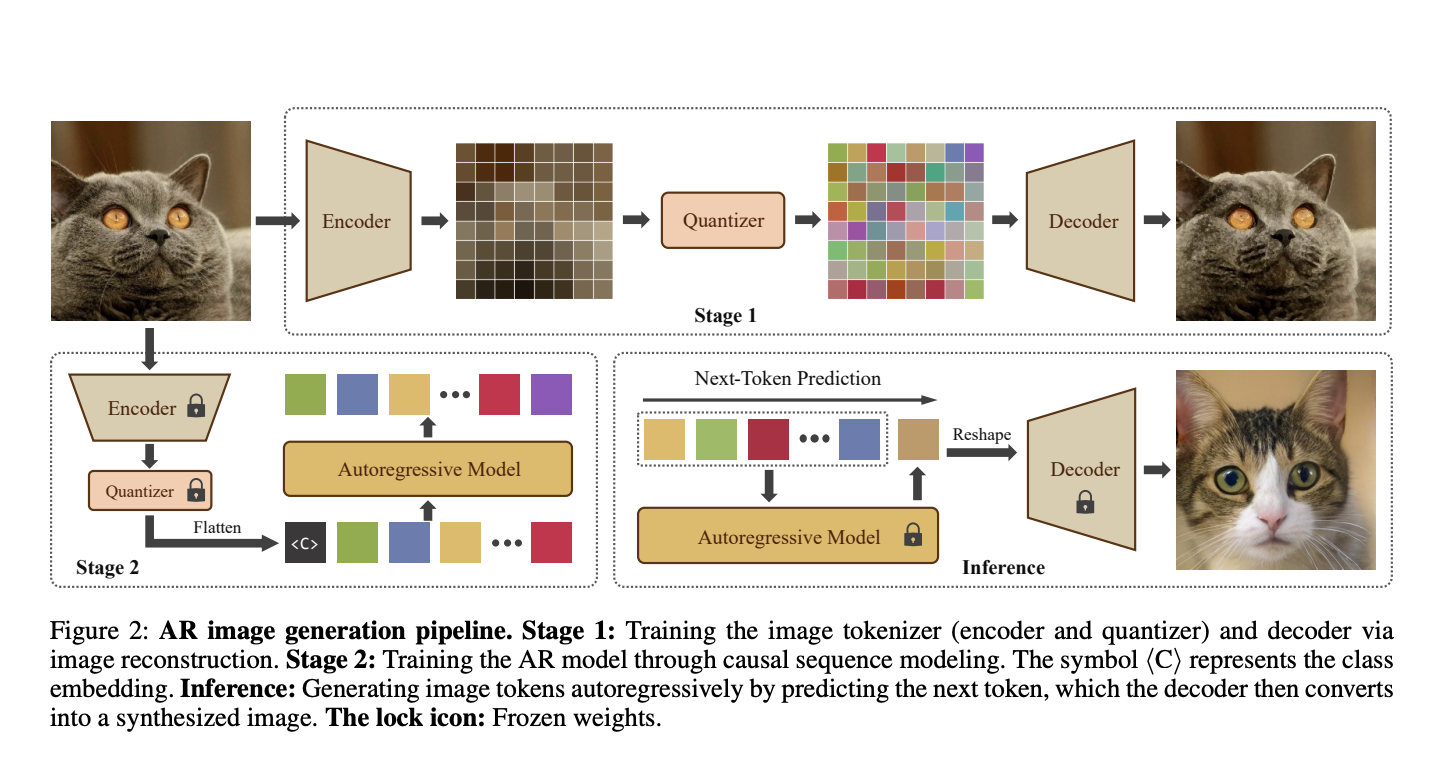
Practical Solutions and Value of AiM: An Autoregressive (AR) Image Generative Model based on Mamba Architecture
Overview
Large language models (LLMs) based on autoregressive Transformer Decoder architectures have advanced natural language processing with outstanding performance and scalability. Recently, diffusion models have gained attention for visual generation tasks, overshadowing autoregressive models (AMs). However, AMs show better scalability for large-scale applications and work more efficiently with language models, making them more suitable for unifying language and vision tasks.
Challenges and Solutions
Existing methods include Vector Quantization (VQ) based models and State Space Models (SSMs) to solve the challenges in autoregressive visual generation (AVG). VQ-based approaches compress images into discrete codes and use AMs to predict these codes. SSMs, especially the Mamba family, have shown potential in managing long sequences with linear computational complexity.
Introduction of AiM
Researchers have proposed AiM, a new Autoregressive image generation model based on the Mamba framework. It is developed for high-quality and efficient class-conditional image generation, making it the first model of its kind. AiM uses positional encoding and a new adaptive layer normalization method called adaLN-Group, optimizing the balance between performance and parameter count. It has shown state-of-the-art performance among AMs on the ImageNet 256×256 benchmark while achieving fast inference speeds.
Evaluation and Performance
AiM was developed in four scales and evaluated on the ImageNet1K benchmark to evaluate its architectural design, performance, scalability, and inference efficiency. It achieved state-of-the-art performance among AMs such as GANs, diffusion models, masked generative models, and Transformer-based AMs. Moreover, AiM has a clear advantage in inference speed compared to other models, with Transformer-based models benefiting from Flash-Attention and KV Cache optimizations.
Conclusion and Future Directions
The effectiveness and efficiency of AiM highlight its scalability and wide applicability in autoregressive visual modeling. However, it focuses only on class-conditional generation, without exploring text-to-image generation, providing directions for future research for further advancements in the visual generation field using state space models like Mamba.
AI Solutions for Business
If you want to evolve your company with AI, stay competitive, and use AiM for your advantage. Identify Automation Opportunities, Define KPIs, Select an AI Solution, and Implement Gradually. For AI KPI management advice, connect with us at hello@itinai.com. And for continuous insights into leveraging AI, stay tuned on our Telegram t.me/itinainews or Twitter @itinaicom.
Discover AI for Sales Processes and Customer Engagement
Discover how AI can redefine your sales processes and customer engagement. Explore solutions at itinai.com.