The text discusses various AI security risks and strategies to mitigate them effectively. These risks include data breaches and privacy concerns, model poisoning, copyright infringement, vulnerabilities in the AI infrastructure, and model inversion attacks. To combat these risks, organizations should implement robust security measures, such as encryption of sensitive data, regular security audits, and employee training on cybersecurity best practices. Privacy regulations should also be followed, and organizations should foster a culture of integrity and respect for intellectual property rights. Additionally, organizations should invest in rigorous data validation processes, continuous monitoring of AI models, and collaboration between AI researchers, cybersecurity experts, and domain specialists to detect and mitigate model poisoning attacks.
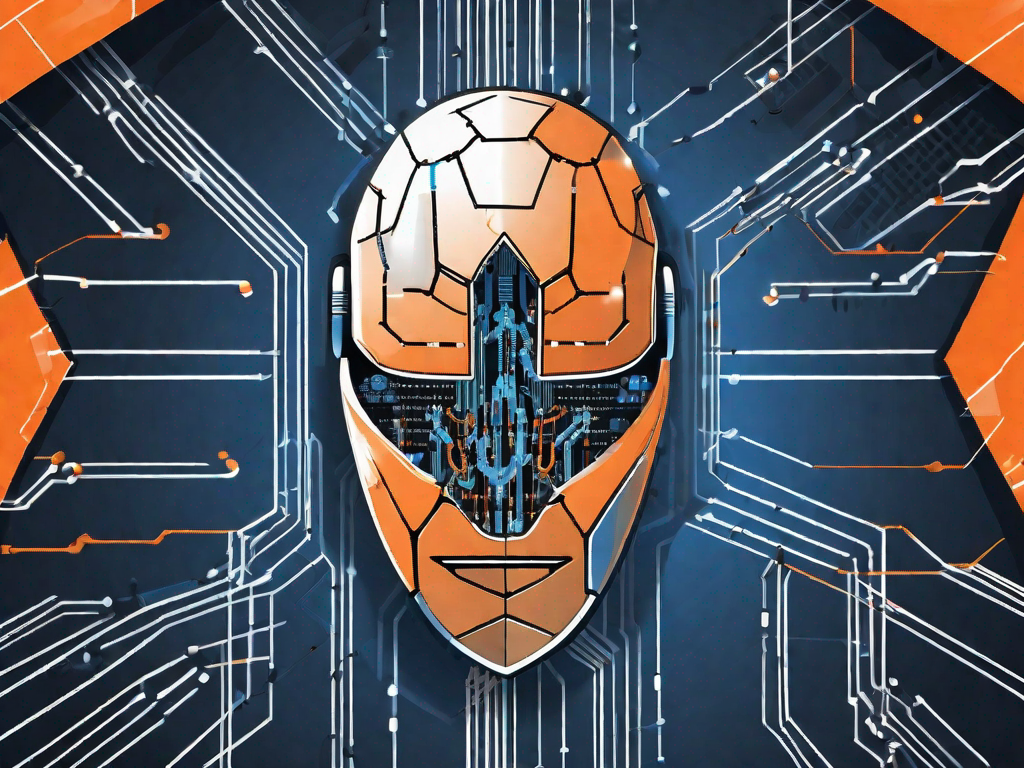
Practical Solutions for Mitigating AI Security Risks
1. Data Breach and Privacy Risks
To protect sensitive information from data breaches, implement robust security measures such as encryption of data, regular security audits, and employee training on cybersecurity best practices. Ensure compliance with privacy regulations like HIPAA and RFPA, which require explicit consent for data collection and the right to access and delete data.
2. Model Poisoning
To defend against model poisoning attacks, implement rigorous data validation processes during the training phase. Carefully examine the training dataset for signs of tampering or malicious injections. Employ anomaly detection algorithms to identify unusual patterns or biases in the AI model’s predictions. Collaborate with AI researchers, cybersecurity experts, and domain specialists to stay ahead of emerging threats.
3. Copyright Infringement and Plagiarism
To combat AI-assisted plagiarism, establish robust systems to verify the authenticity and originality of AI-generated content. Conduct thorough checks to ensure that the content produced does not infringe upon existing copyrights. Establish clear guidelines and policies to educate content creators about the ethical implications of plagiarism. Foster a culture of integrity and respect for intellectual property rights.
4. Vulnerabilities in AI Infrastructure
Protect AI infrastructure by investing in resilience, leveraging AI for rapid threat identification and mitigation, collaborating with government agencies and private sector partners, and prioritizing critical infrastructure security initiatives.
5. Model Inversion
Defend against model inversion attacks by enhancing the privacy of training data, employing differential privacy techniques, limiting output information, applying input perturbation techniques, incorporating adversarial training methods, and aggregating data at a higher level.
Conclusion
As AI technology continues to evolve rapidly, it is crucial to address new and emerging AI security risks. Implement specialized AI security solutions, collaborate within the industry, and adopt a holistic approach to safeguarding data. Mitigate risks through effective implementation, including risk assessments, secure coding practices, robust testing, and an AI security incident response plan. Embrace the transformative potential of AI with LiveHelpNow’s omnichannel customer support suite, integrating seamlessly with AI to enhance security and efficiency.