Predictive policing uses advanced analytics and machine learning to anticipate crimes before they happen. By analyzing historical crime data and other relevant information, algorithms can identify patterns and hotspots of criminal activity. However, recent investigations have revealed failures and ethical concerns, highlighting biases and the potential for inaccurate predictions. The efficacy of predictive policing software, such as Geolitica, has been questioned, with a success rate of less than half a percent. The use of AI in law enforcement also raises questions about human decision-making, accountability, and the perpetuation of biases.
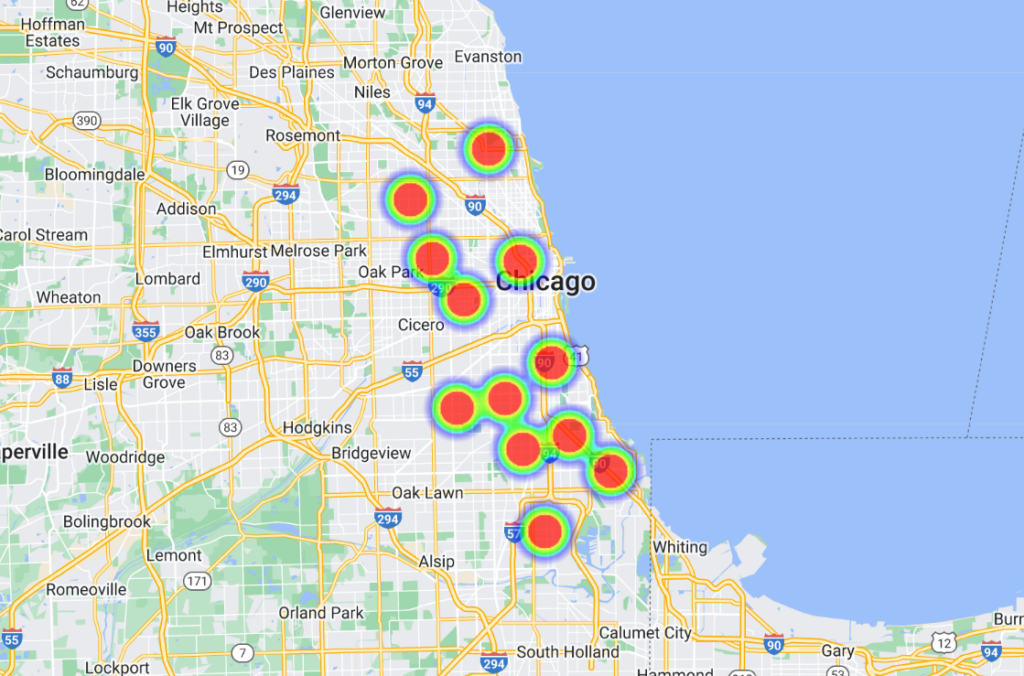
AI Predictive Policing Software Fails in Crime Prediction
Predictive policing, the use of advanced analytics and machine learning to anticipate criminal activities before they happen, has been highly debated and controversial. While the idea of preventing crimes before they occur seems promising, recent investigations have revealed the shortcomings and dangers of relying on AI systems in law enforcement decision-making.
What is Predictive Policing?
Predictive policing involves analyzing historical crime data, surveillance feeds, social media chatter, and other relevant information to identify patterns and forecast future crime occurrences. This information is then transformed into visual representations, such as heat maps, to help law enforcement agencies allocate resources and optimize their patrols and interventions. Some models even provide specific predictions about individuals.
The History of Predictive Policing
Predictive policing technology is still in its early stages, with limited adoption in the industry. In a notable study, the University of Chicago developed an AI model capable of forecasting crime locations and rates within the city with alleged 90% precision. However, the study also revealed biases in police efforts and resource allocation, with affluent neighborhoods receiving more resources than lower-income areas.
Chicago is an important case study in data-driven and AI-aided policing, with initiatives like the gun crime heatmap that identifies crime “hotspots.” However, there have been instances of wrongful targeting based on predictive lists, with a disproportionate number of black men included.
Evidence of Bias and Failures
Recent investigations have uncovered failures and biases in predictive policing software. For example, Geolitica, a predictive policing software used in Plainfield, New Jersey, showed a success rate of less than half a percent in predicting crimes. Analysis of its predictions revealed a lack of accuracy, with less than 100 predictions matching real crime instances.
Beyond the issue of accuracy, there is also growing evidence of biases in AI systems used in law enforcement. Facial recognition technologies, for instance, have shown disproportionate failures for ethnic minority groups and women. AI systems implemented without appropriate oversight and training have resulted in constitutional issues and wrongful arrests.
The Pitfalls of Predictive Policing
Predictive policing raises concerns about unnatural decision-making and the potential for biases to be perpetuated. When algorithms inform police about likely crime scenes, it can prime them to view situations through a lens of expectancy, leading to confirmation bias. Algorithms can also be viewed as authoritative, leading to undue trust and moral disengagement on the part of officers.
Overall, the current evidence suggests that predictive policing often reinforces biases and can have potentially catastrophic impacts when combined with human decision-making.
Using AI to Evolve Your Company
If you want to stay competitive and leverage AI to redefine your way of work, consider the following steps:
- Identify Automation Opportunities: Locate key customer interaction points that can benefit from AI.
- Define KPIs: Ensure your AI endeavors have measurable impacts on business outcomes.
- Select an AI Solution: Choose tools that align with your needs and provide customization.
- Implement Gradually: Start with a pilot, gather data, and expand AI usage judiciously.
To learn more about AI KPI management and continuous insights into leveraging AI, connect with us at hello@itinai.com or stay tuned on our Telegram channel t.me/itinainews or Twitter @itinaicom.
Spotlight on a Practical AI Solution: AI Sales Bot
Discover how AI can redefine your sales processes and customer engagement with the AI Sales Bot from itinai.com/aisalesbot. This solution is designed to automate customer engagement 24/7 and manage interactions across all customer journey stages.