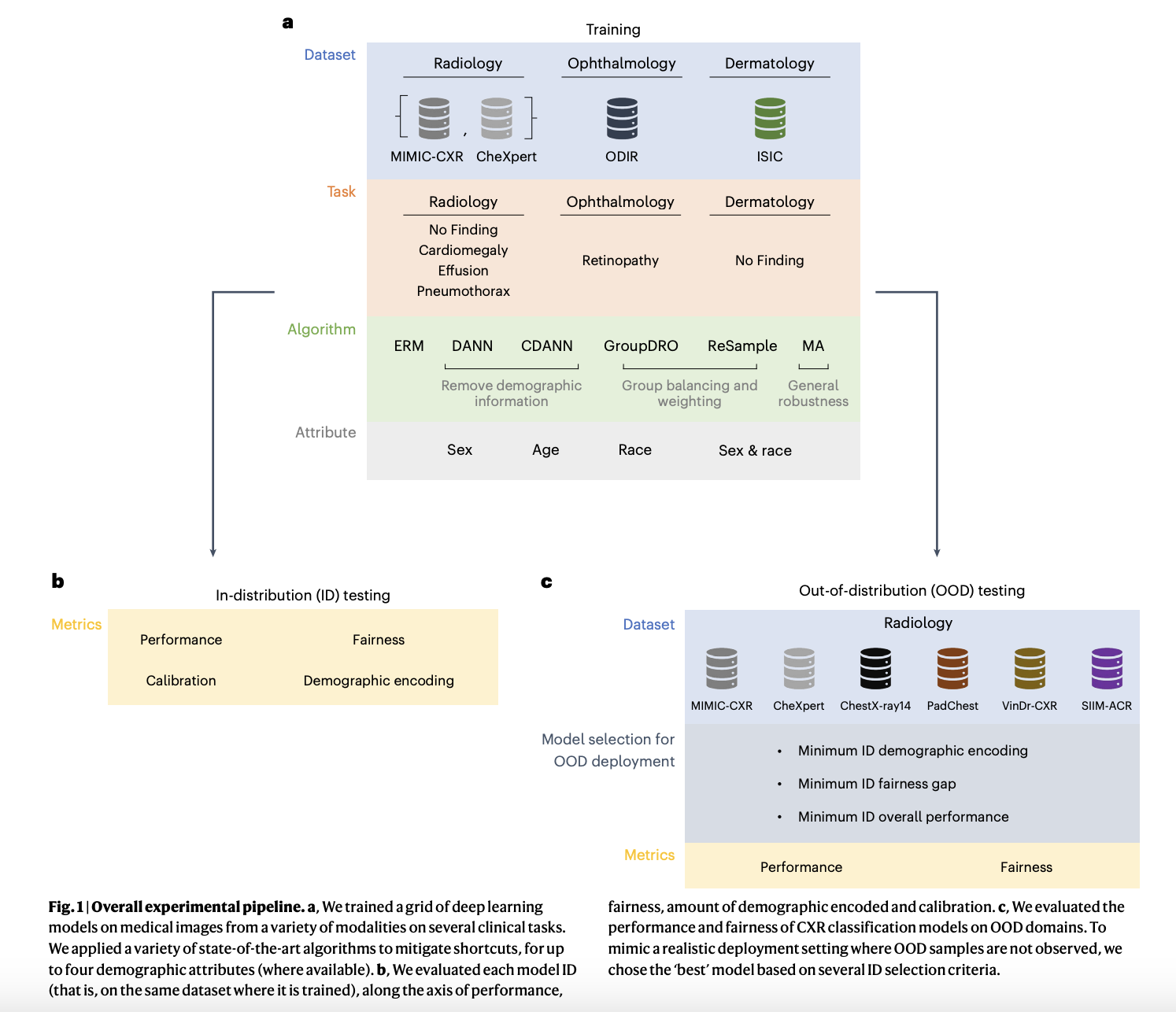
Practical Solutions for AI Bias in Medical Imaging
Identifying and Addressing Biases in AI Models
As AI models are integrated into clinical practice, it’s crucial to assess their performance and biases. Deep learning in medical imaging often inherits biases from the data, affecting performance across different demographic groups. For example, chest X-ray classifiers may underdiagnose conditions in certain patient groups, leading to delayed care.
Debiasing Techniques and Fairness Evaluation
Recent studies show the unexpected capability of deep models to predict demographic information from medical images more accurately than radiologists. This raises concerns about disease prediction models using demographic features as misleading shortcuts. A recent study in Nature Medicine examines how demographic data may bias disease classification models, evaluating the effectiveness of debiasing techniques and fairness assessment.
Evaluating Fairness Across Demographic Groups
The research team conducted experiments to evaluate AI models’ performance and fairness across various demographic groups and modalities in chest X-ray, dermatology, and ophthalmology tasks. Fairness was assessed using metrics like false-positive rates and false-negative rates, emphasizing equalized odds to measure performance disparities across demographic subgroups.
Strategies for Ensuring Fair and Equitable AI Deployment
From the experiments, the authors observed that demographic encoding can significantly impact fairness, particularly under distribution shifts. The study emphasizes the tradeoff between fairness and other clinically meaningful metrics and the need for continuous monitoring of AI models in clinical environments to address fairness degradation. In conclusion, it emphasizes the necessity of employing efficient debiasing strategies, ongoing monitoring, and meticulous model selection to ensure trustworthy and equitable AI deployment.
Value of AI in Medical Imaging: Balancing Performance and Fairness
As AI becomes integrated into clinical practice, it’s essential to comprehend the biases AI models may acquire from training data. The study highlights the challenge of enhancing fairness while retaining performance, especially in real-world settings. To ensure trustworthy and equitable AI systems, efficient debiasing strategies, ongoing monitoring, and meticulous model selection are essential.
Discover the Power of AI for Your Business
Automation Opportunities, KPIs, and AI Solutions
Identify key customer interaction points that can benefit from AI, ensure measurable impacts on business outcomes, choose AI tools aligned with your needs, and gradually implement AI usage starting with a pilot and expanding judiciously.
Connect and Stay Informed
For AI KPI management advice and continuous insights into leveraging AI, connect with us at hello@itinai.com. Stay tuned on our Telegram t.me/itinainews or Twitter @itinaicom for ongoing insights and solutions.