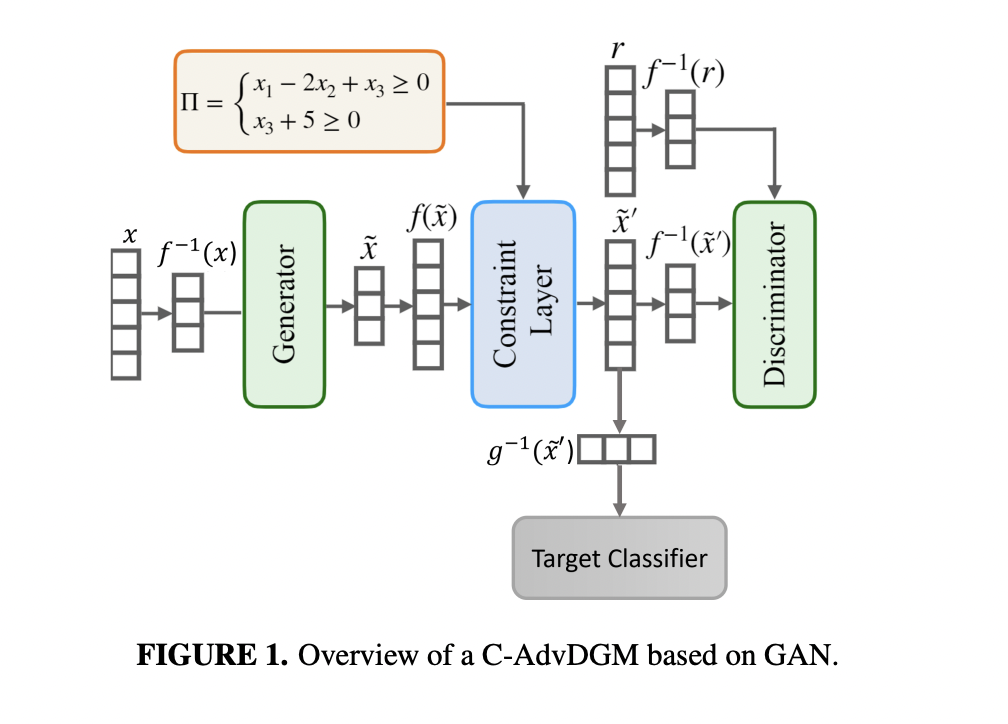
Practical Solutions for Enhancing Adversarial Robustness in Tabular Machine Learning
Value Proposition:
Adversarial machine learning focuses on testing and strengthening ML systems against deceptive data. Deep generative models play a crucial role in creating adversarial examples, but applying them to tabular data presents unique challenges.
Challenges in Tabular Data:
Tabular data complexity arises from intricate relationships between various data types like categorical and numerical variables. Ensuring realistic constraints, such as in finance, is essential to generate meaningful adversarial examples for evaluating ML model security.
Innovative Approach:
Researchers have developed constrained adversarial DGMs (C-AdvDGMs) by enhancing existing DGMs with a constraint repair layer. This innovation allows for the creation of adversarial data that not only alters model predictions but also adheres to domain-specific rules.
Key Advancements:
The constraint repair layer ensures that generated adversarial examples meet predefined constraints, maintaining realism in the data. This approach significantly improves the Attack Success Rate (ASR) of models like AdvWGAN, showcasing the effectiveness of the method in enhancing ML model robustness.
Impact and Future:
This research bridges a critical gap in adversarial machine learning for tabular data, offering a way to generate realistic adversarial examples while upholding real-world relationships. By leveraging AdvDGMs with constraint repair layers, businesses can enhance the security of their ML models in structured domains.