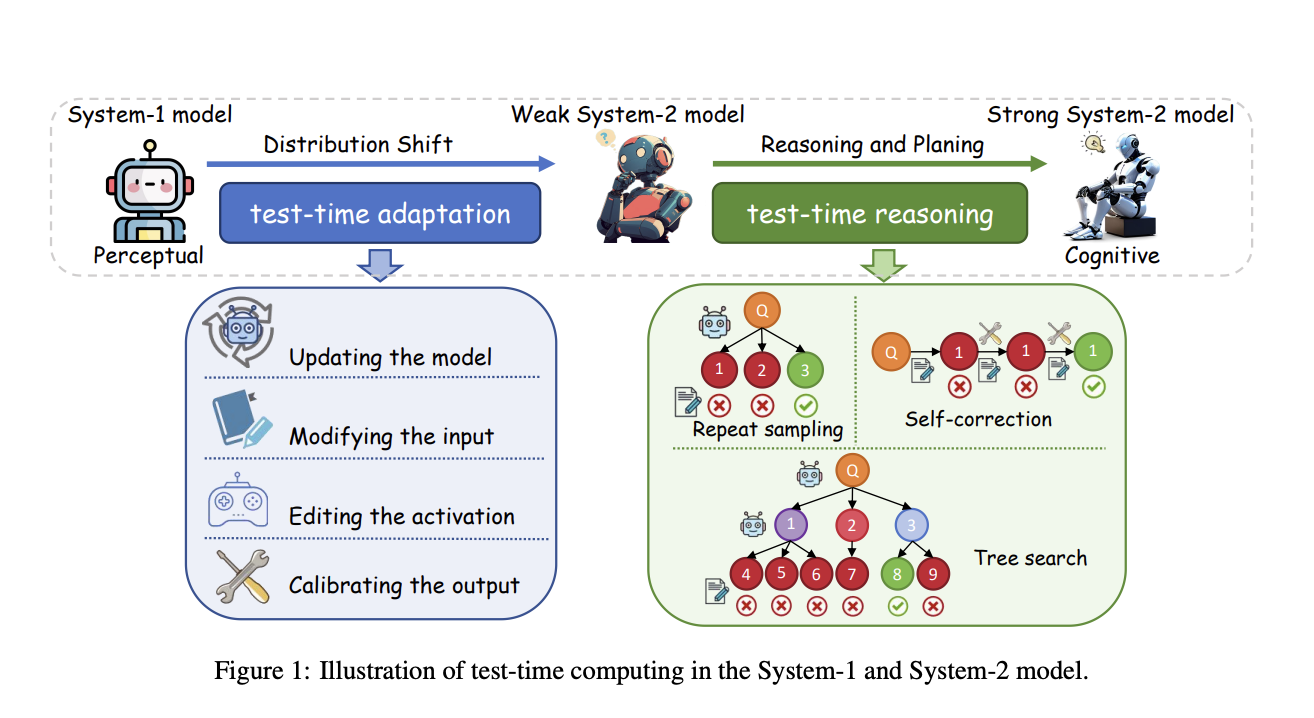
Understanding the o1 Model and Its Impact on AI
The o1 model shows great potential for AI by enhancing complex reasoning through a method called test-time computing scaling. This approach focuses on improving System-2 thinking by using more computational resources during inference, which helps in making more accurate decisions. OpenAI’s o1 model, launched in 2024, demonstrates that increasing computing power can significantly improve the model’s performance in challenging reasoning tasks.
Challenges in Current AI Models
Most AI models today rely on System-1 thinking, which is fast but not very adaptable. They often struggle with complex tasks and can be less reliable. While efforts have been made to improve these models, like test-time adaptation (TTA), their ability to handle complex reasoning remains limited.
Advancements in Test-Time Computing
Researchers from various institutions have explored how test-time computing has evolved from basic models to more advanced systems. Initially, this method enhanced robustness in System-1 models. Now, it’s being used to boost reasoning in System-2 models through techniques such as repeated sampling and self-correction, allowing AI to reflect on its mistakes and improve its reasoning.
Key Techniques in Test-Time Adaptation
TTA enhances model performance during inference by using information from test samples. Key aspects include:
- Learning Signals: Methods like Test-time Training (TTT) help models adapt through auxiliary tasks.
- Feedback Mechanisms: Including human feedback to improve tasks like question answering.
- Efficiency Improvements: Targeted parameter updates to enhance specific layers of the model.
Future Directions in Test-Time Computing
The future of test-time computing involves several important areas:
- Broader Generalization: Extending the capabilities of System-2 models beyond specific tasks to support scientific research.
- Multimodal Integration: Combining different forms of data, such as audio and video, to align more closely with human thinking.
- Efficiency vs. Performance: Balancing resource use while maintaining high performance.
- Universal Scaling Laws: Developing consistent rules for effectively scaling AI models.
Join the Movement
Discover how AI can transform your business. Here are practical steps you can take:
- Identify Opportunities: Find customer interaction points that can benefit from AI.
- Define KPIs: Ensure your AI projects have measurable impacts.
- Select the Right Tools: Choose AI solutions that meet your specific needs.
- Implement Gradually: Start small, learn, and scale up.
For expert AI KPI management advice, contact us at hello@itinai.com. Stay updated by following us on Telegram or on @itinaicom.
Explore solutions and redefine your sales and customer engagement processes at itinai.com.