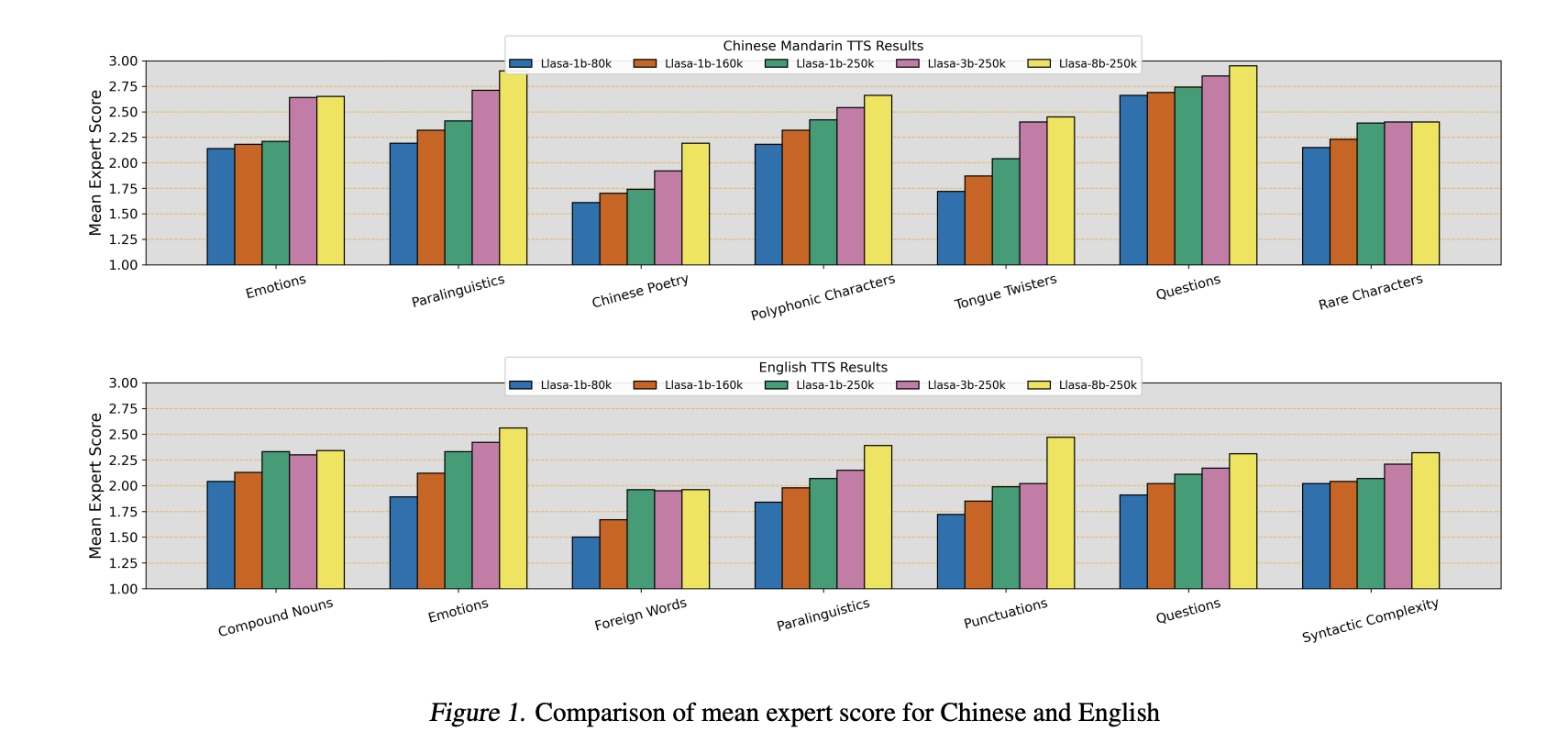
Recent Advances in Text-to-Speech Technology
Understanding the Benefits of Scaling
Recent developments in large language models (LLMs), like the GPT series, show that increasing computing power during both training and testing phases leads to better performance. While expanding model size and data during training is common, using more resources during testing can significantly enhance output quality and handle complex tasks more effectively. This approach has been largely applied to text models but is still underused in speech synthesis.
Streamlining Text-to-Speech Systems
Many existing text-to-speech (TTS) systems use complex multi-stage architectures. These systems combine LLMs with other processing models, making scaling decisions more complicated. In contrast, single-stage TTS architectures simplify the process by directly modeling speech tokens. This method reduces complexity, improves scalability, and allows for large training without heavy memory use. Evaluations show that these architectures outperform traditional models in areas like zero-shot speech synthesis and emotional expression.
Introducing Llasa: A New TTS Model
Researchers from various universities have developed Llasa, a Transformer-based TTS model that aligns with standard LLM structures. By scaling computing during training and testing, Llasa enhances speech quality, emotional expressiveness, and accuracy. The model is publicly available, encouraging further research in TTS technology.
How Llasa Works
Llasa uses a tokenizer and a Transformer-based architecture similar to text LLMs. It features a unique speech tokenizer that converts audio into discrete tokens, then decodes them back into high-quality sound. This model learns to generate speech based on text input, optimizing performance through effective training data and model size scaling.
Performance Evaluation
The speech tokenizer has been tested against various models using metrics like Word Error Rate (WER) and speech quality evaluations. Results indicate that it performs exceptionally well, especially at lower token rates, providing better speech quality compared to other codecs. The models improve their understanding and learning capabilities with larger sizes and datasets.
Conclusion: The Future of TTS with Llasa
Llasa represents a significant step forward in TTS technology, utilizing a single Transformer model that aligns closely with text-based LLMs. By exploring both training and testing scaling, it shows that larger models can improve speech quality and comprehension. The model also enhances emotional expressiveness and accuracy, demonstrating impressive performance in various applications.
For more details, check out the Paper and GitHub Page. Follow us on Twitter, join our Telegram Channel, and connect with our LinkedIn Group. Join our 75k+ ML SubReddit for ongoing discussions.
Transform Your Business with AI
Stay competitive by leveraging advancements in scalable TTS technology like Llasa. Here’s how AI can redefine your operations:
Identify Automation Opportunities
Find key customer interactions that can benefit from AI solutions.
Define KPIs
Ensure your AI initiatives have measurable impacts on your business goals.
Select an AI Solution
Choose tools that meet your specific needs and allow for customization.
Implement Gradually
Start with pilot projects, gather insights, and expand AI usage thoughtfully.
For AI KPI management advice, contact us at hello@itinai.com. For continuous insights into AI, follow us on Telegram or @itinaicom.
Explore how AI can enhance your sales processes and customer engagement at itinai.com.