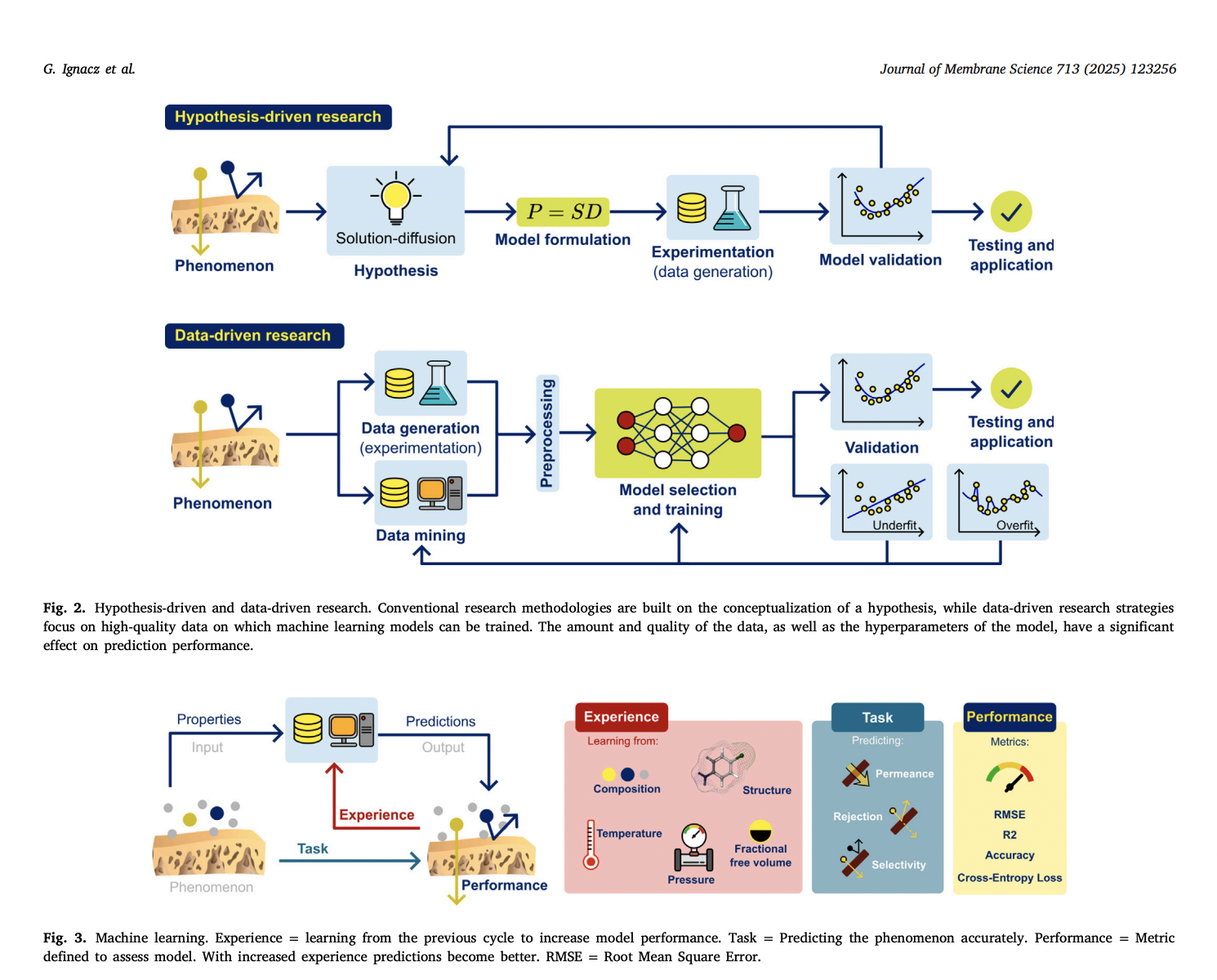
Machine Learning in Membrane Science
Practical Solutions and Value:
ML transforms natural sciences like cheminformatics and materials science, benefiting membrane technology. ML applications analyze data to improve processes like reverse osmosis and gas separation, enhancing membrane design and performance.
Machine Learning Approaches in Membrane Science
Practical Solutions and Value:
ML techniques model physical phenomena without predefined theories, improving predictive accuracy. Effective featurization techniques optimize models by transforming molecular structures for better predictions, addressing challenges in membrane science.
Advancements in Membrane Technology through Machine Learning Innovations
Practical Solutions and Value:
ML innovations optimize membrane performance, reduce costs, and enhance efficiency. Predictive models identify structure-property relationships, tackle fouling issues, and improve operational parameters in applications like wastewater treatment and gas separation.
Guidelines for Machine Learning in Membrane Science
Practical Solutions and Value:
Adopting best ML practices enhances reproducibility in membrane applications. Reliable data sources, proper validation, and model explainability are crucial. Ethical considerations and transparent reporting foster trust and effective knowledge sharing in the research community.