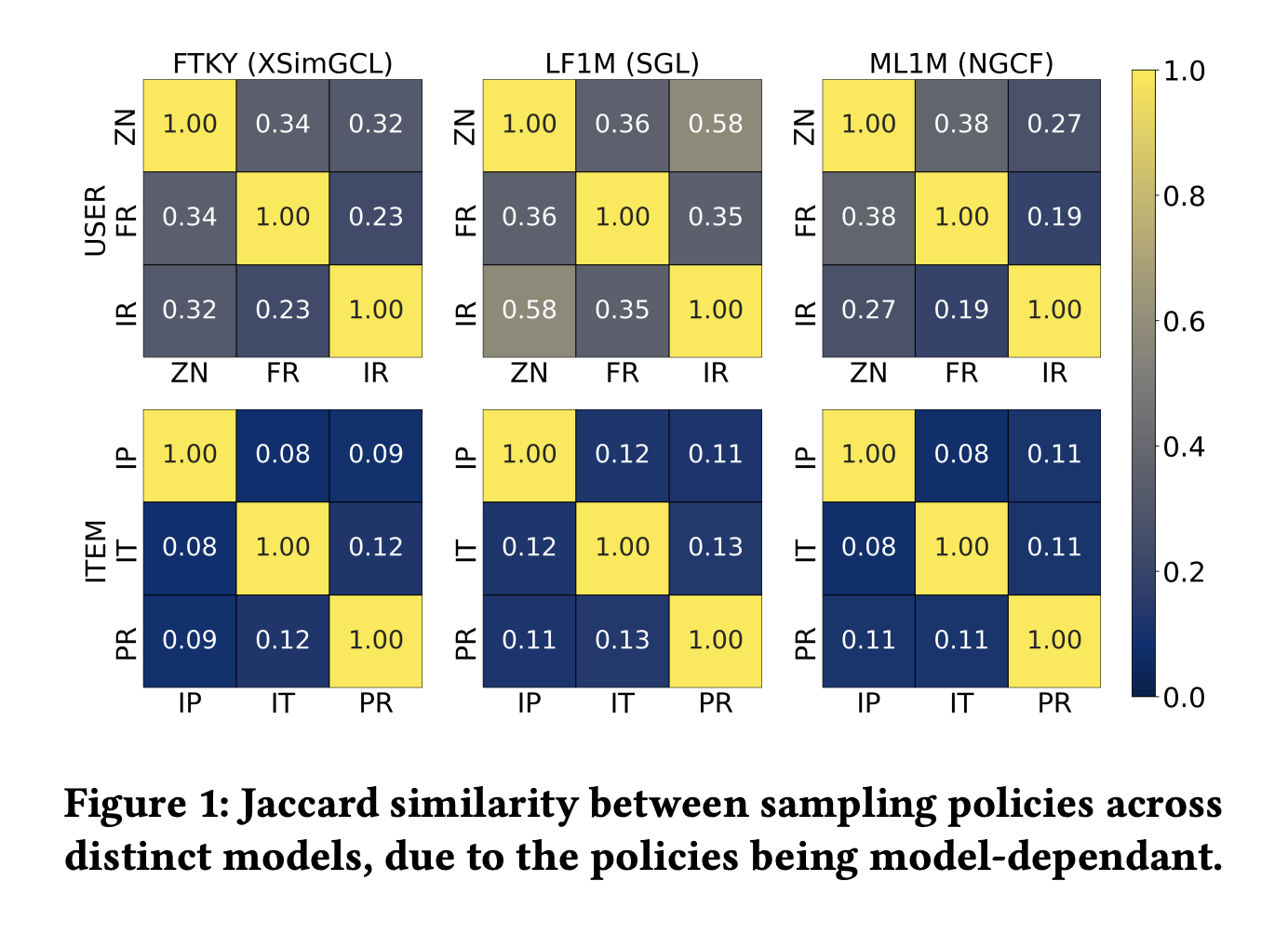
Practical Solutions for Fairness in Recommender Systems
Addressing Unfairness in Recommendations
Recommender systems are powerful tools for personalized suggestions, but concerns about trustworthiness and fairness have arisen. To tackle unfairness, algorithms have been developed and categorized into pre-processing, in-processing, and post-processing approaches.
Enhanced Mitigation Techniques
A detailed approach has been proposed to address the limitations of previous fairness-aware graph collaborative filtering (GCF) methods. This includes theoretical formalization of sampling policies, augmented graph integration in graph neural networks (GNNs), and the introduction of FA4GCF (Fair Augmentation for Graph Collaborative Filtering) – a versatile, publicly available tool.
Expanded Scope of Evaluation
The proposed method significantly expands the scope of evaluation compared to previous studies by replacing datasets and extending the experimental evaluation to include datasets from diverse domains such as movies, fashion, and points of interest in cities. Consistent pre-processing steps are applied, and a temporal user-based splitting strategy is adopted.
Impact on Different Models and Datasets
The results reveal that fairness mitigation techniques have varying impacts across different models and datasets, with different sampling policies exhibiting varying effectiveness. The evaluation covered diverse datasets, models, and fairness metrics, providing a more detailed assessment of the algorithm’s effectiveness.
Establishing a Robust Framework
This paper provides valuable insights into the complexities of fairness mitigation in GCF and establishes a robust framework for future research in the recommender systems field.
AI Solutions for Business
Discover how AI can redefine your way of work and sales processes. Identify automation opportunities, define KPIs, select an AI solution, and implement gradually. For AI KPI management advice and continuous insights into leveraging AI, connect with us at hello@itinai.com and stay tuned on our Telegram t.me/itinainews or Twitter @itinaicom.