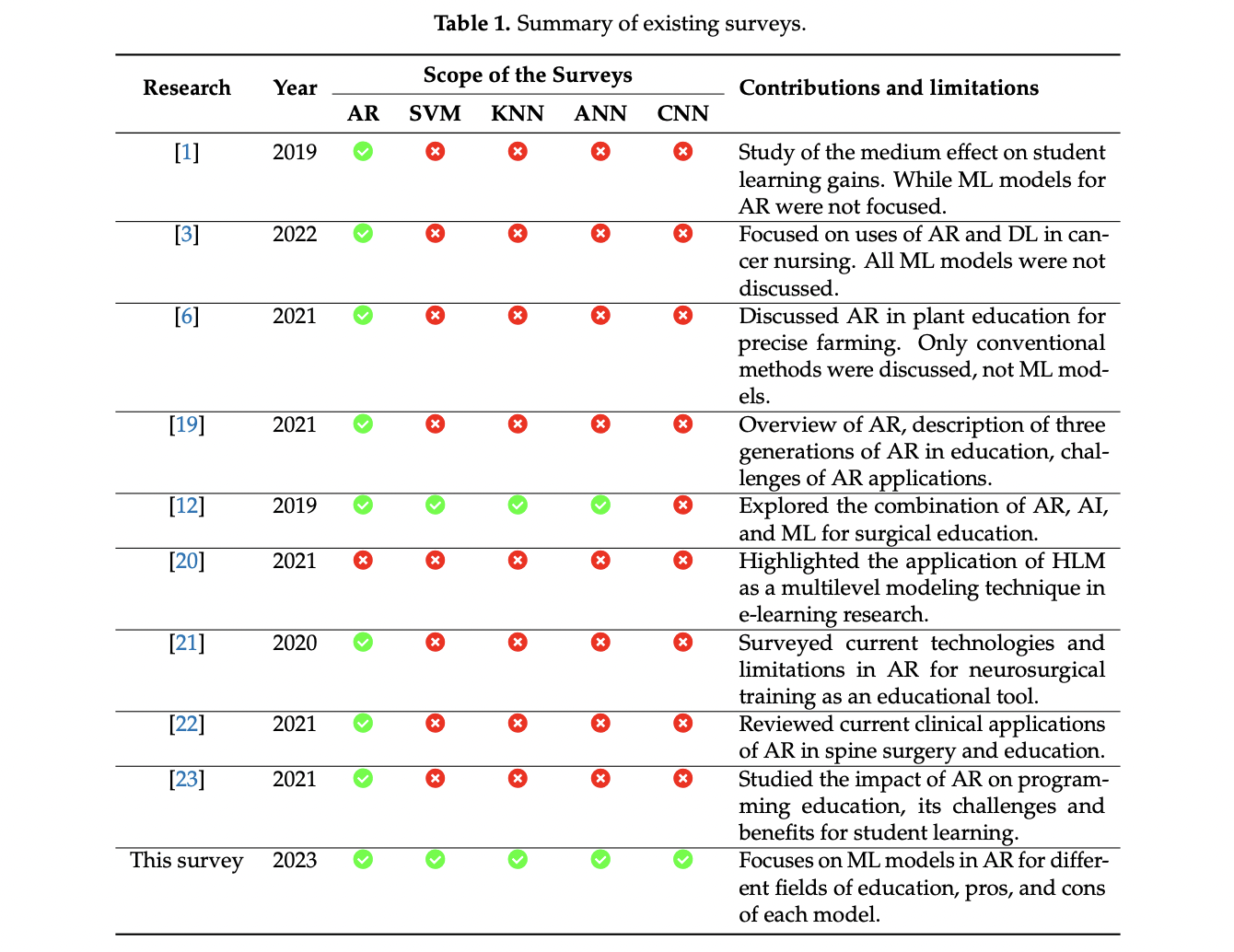
Machine Learning-Powered Augmented Reality in Education
Practical Solutions and Value
Machine learning (ML) is advancing augmented reality (AR) in education, enhancing object visualizations and interaction capabilities. ML models like support vector machines, CNNs, and ANNs are being integrated into AR for diverse educational fields, from kindergarten to university. This integration aims to address traditional educational issues, improve collaboration, and guide future research and development in educational technology.
Analysis of ML-Based AR in Education
Practical Solutions and Value
ML-based AR is enhancing medical education, particularly in surgical training and patient data analysis. The application of ML models like CNN, ANN, and SVM in AR for healthcare, agriculture, and e-learning is being explored. Identifying challenges in integrating ML and AR, this analysis emphasizes the need for a detailed examination of ML models in AR across educational fields to understand their benefits, limitations, and evolving trends.
Overview of Machine Learning Techniques
Practical Solutions and Value
ML automates the creation of analytical models using training data, vital in applications such as image and speech recognition, intelligent assistants, and autonomous vehicles. Categorized into four types, including Supervised Learning, Unsupervised Learning, Semi-Supervised Learning, and Reinforcement Learning, ML employs different algorithms for diverse real-world applications.
Introduction to Augmented Reality
Practical Solutions and Value
AR blends digital information with the physical world, offering immersive 3D experiences through devices like smartphones and tablets. It is used in various educational settings, benefiting diverse learner groups, and offers three main types of AR systems: Marker-Based AR, Marker-Less AR, and Location-Based AR. Integrating machine learning models with AR further enriches educational experiences.
ML Techniques for AR in Education
Practical Solutions and Value
Various ML techniques, including Support Vector Machines, K-Nearest Neighbors, ANNs, and CNNs, are enhancing educational experiences in AR applications. Integration of ML, such as SVM and CNN, in AR has shown promising results in enhancing educational experiences, motor skills assessment, and interactive learning.
SL and USL Models in AR
Practical Solutions and Value
Research has explored gesture recognition in AR for children’s education, the emergence of ARChem mobile app for chemistry students, and an interactive multi-meter tutorial using AR and DL. These innovations showcase the potential of ML and AR in enhancing technical education.
Conclusion
Practical Solutions and Value
This survey provides an overview of current applications of ML-powered AR in education, emphasizing the need for future studies to focus on subject-specific applications and address ethical considerations. Evaluating the impact of ML-powered AR on student engagement and learning outcomes is essential for its effective implementation.