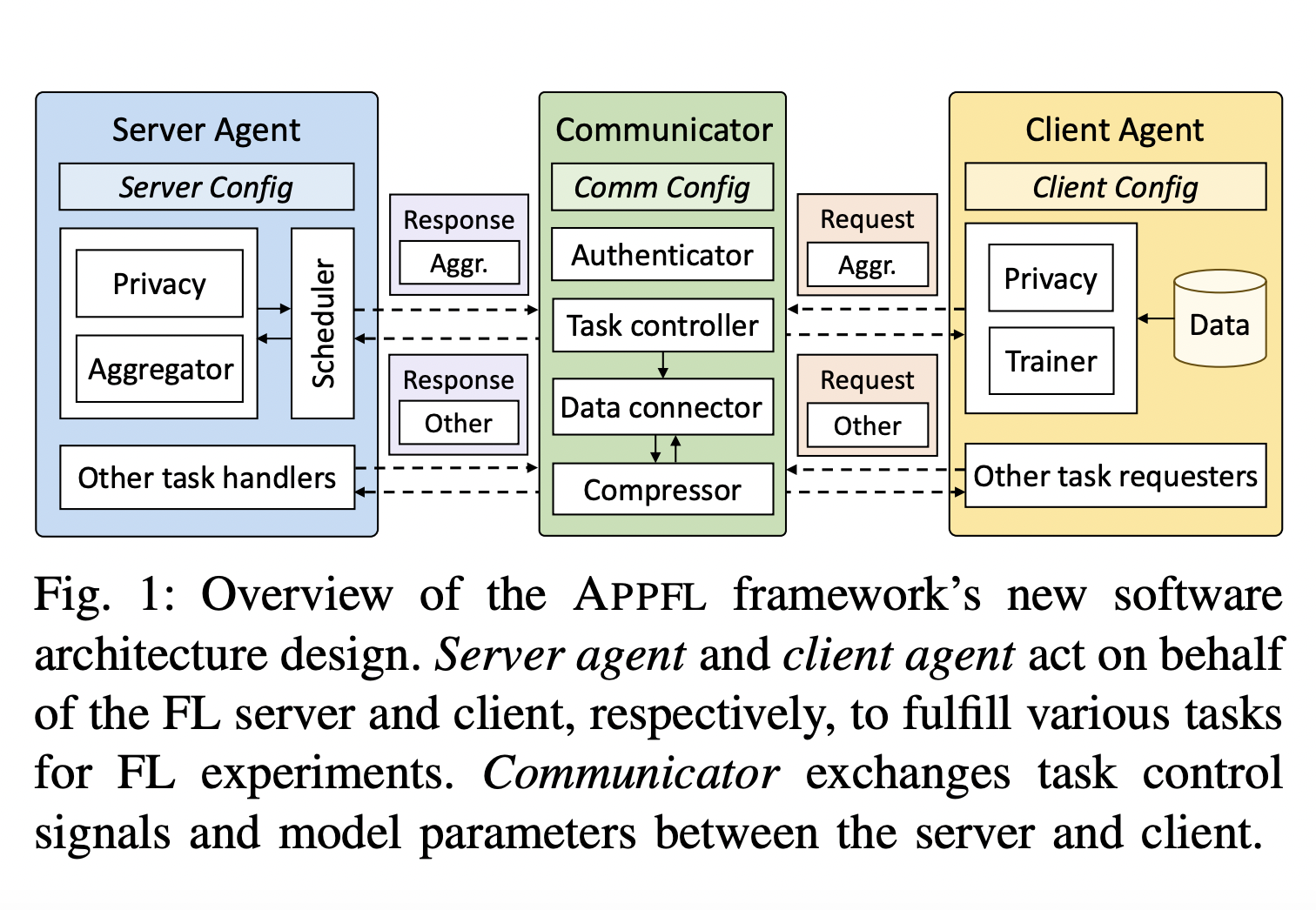
<>
Practical Solutions and Value of Advanced Privacy-Preserving Federated Learning (APPFL)
Overview:
Federated learning enables multiple data owners to collaboratively train models without sharing their data, crucial for privacy-sensitive sectors like healthcare and finance.
Challenges:
Challenges include data heterogeneity, computational variations, and security risks from model updates.
APPFL Framework:
Developed by researchers, APPFL offers solutions for technical and security challenges in federated learning.
Key Features:
- Supports synchronous and asynchronous aggregation
- Robust privacy-preserving mechanisms
- Efficient communication protocols and compression techniques
Performance:
APPFL reduces communication time by 40%, training time by 30%, and maintains high model accuracy in real-world scenarios.
Advantages:
- Improved efficiency and accuracy of federated learning models
- Adaptability across various deployment scenarios
- Enhanced privacy protection and model performance
Conclusion:
APPFL is a leading solution for decentralized machine learning, offering advancements in data privacy, computational efficiency, and model accuracy.
>