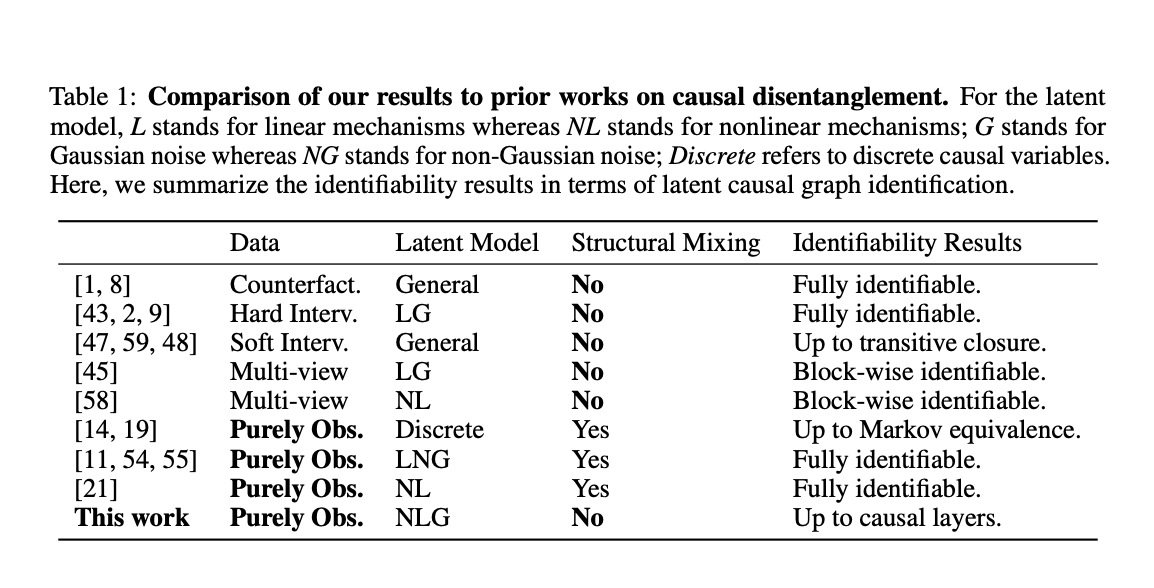
Causal Disentanglement in Machine Learning
What is Causal Disentanglement?
Causal disentanglement isolates hidden causal factors from complex data without needing direct manipulation. This is important in fields like computer vision, social sciences, and life sciences, allowing predictions of data behavior in hypothetical scenarios.
Why is it Valuable?
This method enhances the interpretability and generalizability of machine learning, which is crucial for making reliable predictions in real-world applications.
Key Challenges
The main obstacle is identifying latent causal factors from observational data, as traditional methods often depend on interventional data, which isn’t always possible due to ethical or logistical reasons.
Innovative Solutions
Researchers at the Broad Institute of MIT and Harvard have developed a new approach to causal disentanglement using only observational data. Their method does not rely on interventional access and uses nonlinear models to effectively identify causal relationships.
How It Works
This innovative approach leverages natural distributional asymmetries in the data, allowing for the detection of causal relationships. It combines score matching with quadratic programming to efficiently infer causal structures from observed data.
Results and Effectiveness
In testing, the algorithm successfully handled various causal configurations, demonstrating high accuracy in isolating causal factors. These results validate its reliability, even in noisy conditions, showcasing its potential in practical applications.
Practical Implications
This research opens doors for causal discovery in many fields, especially where direct interventions are challenging. It provides a flexible and efficient way to make causal inferences from purely observational data.
How Can Your Business Benefit?
If you’re looking to adapt your company through AI, consider these steps:
- Identify Automation Opportunities: Find customer interaction points that could benefit from AI.
- Define KPIs: Ensure your AI efforts can be measured against business outcomes.
- Select an AI Solution: Pick tools that meet your specific needs and allow for customization.
- Implement Gradually: Start small with pilot programs, gather data, and carefully expand.
Stay Connected
For AI KPI management advice, reach out to us at hello@itinai.com. For continuous insights into AI, follow us on Telegram or on @itinaicom.
Join Us Live
Don’t miss our upcoming LinkedIn event with Encord CEO Eric Landau and Head of Product Engineering Justin Sharps as they discuss transforming the data development process for innovative AI models.
Learn More
Discover advanced solutions to redefine your sales processes and enhance customer engagement at itinai.com.