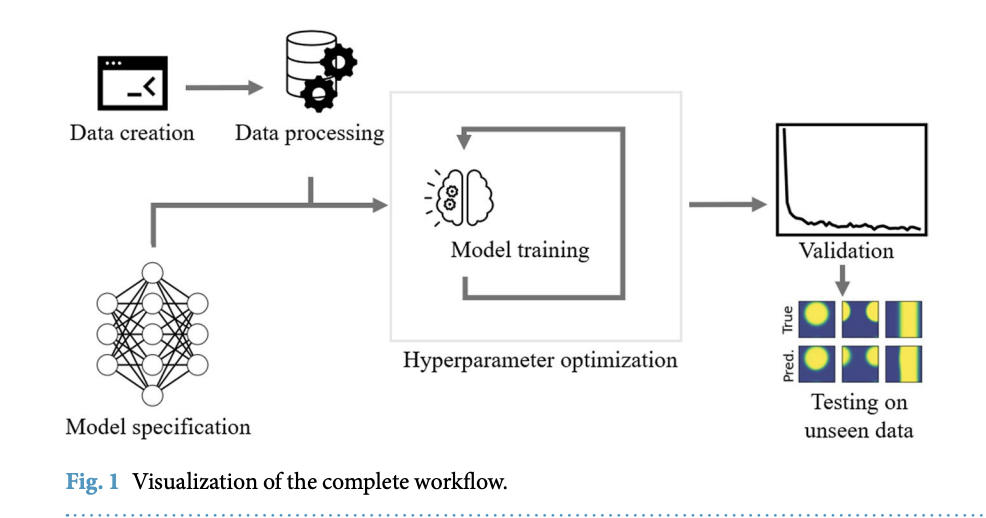
Phase-Field Models and Their Importance
Phase-field models are essential for simulating material behavior by connecting atomic-level details to larger-scale effects. They help in understanding microstructural changes and phase transformations, which are important in various processes like grain growth and crack propagation. These models are particularly significant in the field of battery materials research, where they analyze how materials behave during charging and discharging cycles.
Challenges and Solutions
However, these simulations can be very computationally demanding, making it hard to explore different designs and long-term behaviors effectively. By integrating machine learning (ML) with phase-field modeling, we can overcome these challenges. ML models, when trained on quality data, can quickly predict simulation outcomes, leading to faster analyses. This combination enhances material discovery and optimization in energy storage technologies.
New Dataset and Methodology
Researchers from DTU, Sloveniaβs National Institute of Chemistry, and the University of Ljubljana have created a publicly available dataset for testing ML algorithms in phase-field simulations. They used a model based on the Cahn-Hilliard equation for lithium iron phosphate (LFP) battery electrodes to generate this valuable dataset.
Dataset Features
The dataset includes 1,100 simulation trajectories that capture microstructure changes during lithiation. It has been validated using a U-Net-based ML model, which can predict entire simulation paths without needing intermediary steps. The model showed high accuracy across various conditions.
Implementation and Results
The phase-field model is implemented in efficient C code and uses the Cahn-Hilliard equation to simulate microstructural evolution. This methodology involves using a finite volume method (FVM) for effective numerical solutions. Simulations are conducted on high-performance computing (HPC) systems, allowing for comprehensive analysis through stored outputs like concentration fields and animations.
Training and Validation
The dataset was validated using U-Net and SegFormer architectures, both trained on powerful GPUs. The U-Net model effectively manages different features, while the SegFormer serves as a complementary benchmark. Training utilized techniques to avoid overfitting and focused on minimizing prediction errors.
Conclusion and Future Directions
The study presents a well-documented dataset aimed at enhancing ML applications in phase-field simulations. The validated U-Net model proves effective across various design sizes, indicating the dataset’s potential for advancing domain-independent models.
Get Involved!
Check out the Paper for more insights. Join us on Twitter, Telegram, and LinkedIn to stay connected. If you appreciate our work, consider subscribing to our newsletter and joining our 55k+ ML SubReddit.
Upcoming Event
Don’t miss the FREE AI VIRTUAL CONFERENCE – SmallCon, featuring industry leaders like Meta and Salesforce on December 11th. Discover how to effectively build with small models.
Leverage AI for Your Business
To enhance your company with AI, consider the following steps:
- Identify Automation Opportunities: Find customer interaction points where AI can add value.
- Define KPIs: Ensure your AI initiatives yield measurable results.
- Select an AI Solution: Choose tools that match your requirements and allow customization.
- Implement Gradually: Start with a pilot project, collect data, and expand AI use wisely.
For advice on AI KPI management, connect with us at hello@itinai.com. Stay updated with valuable AI insights on our Telegram and Twitter.
Transform Your Sales and Customer Engagement
Discover how AI can revolutionize your sales processes and customer interactions by visiting itinai.com.