The text can be summarized as follows:
The article discusses the use of LoRA (Low-Rank Adaptation) for fine-tuning language models. The summary highlights the practical strategies for achieving good performance and parameter efficiency using LoRA. It also addresses the impact of hyperparameters and design decisions on performance, GPU memory utilization, and training speed. The article includes empirical insights on tuning specific model components and provides practical guidance for applying LoRA in model training. Finally, it outlines the authors’ approach for selecting hyperparameters and summarizes key findings from the experiments.
Let me know if you need more information on any specific part of the text.
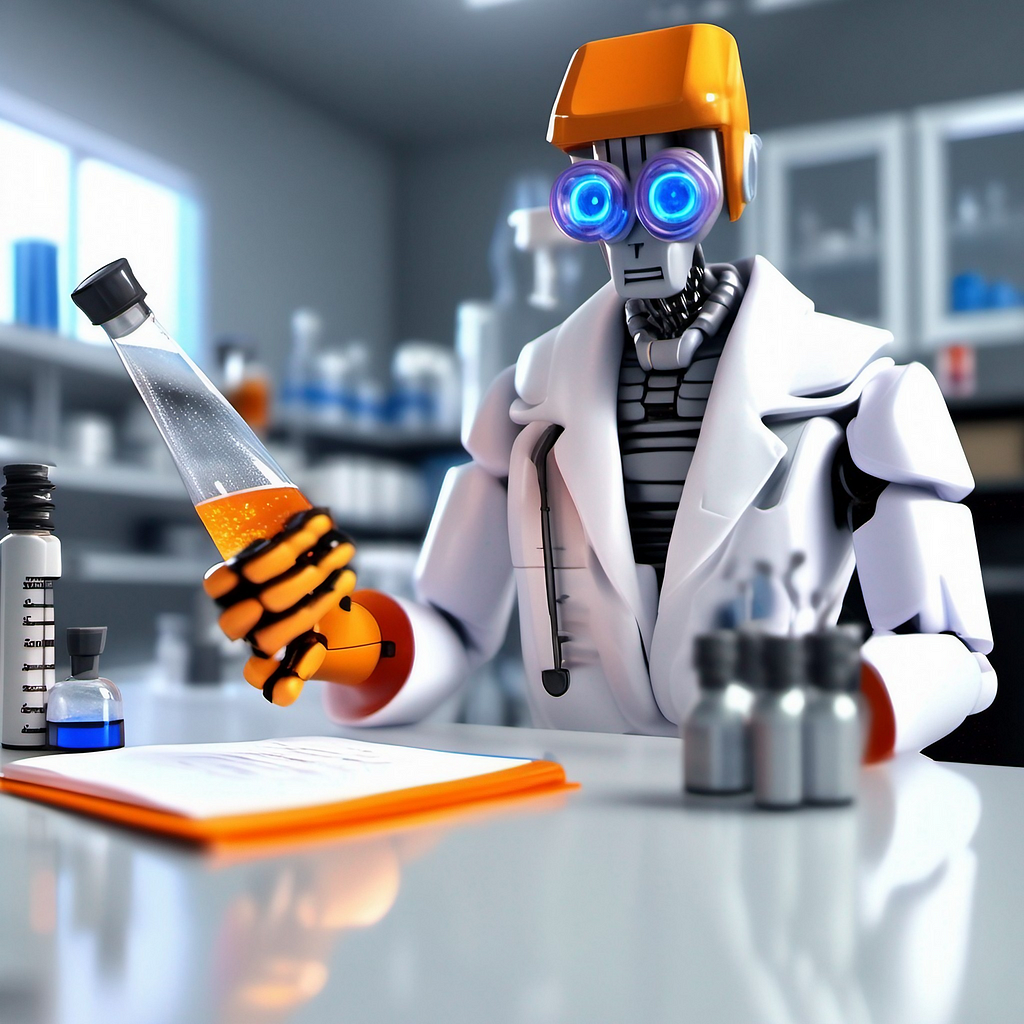
“`html
A Winding Road to Parameter Efficiency
If you want to evolve your company with AI, stay competitive, use for your advantage A Winding Road to Parameter Efficiency.
Discover how AI can redefine your way of work.
- Identify Automation Opportunities: Locate key customer interaction points that can benefit from AI.
- Define KPIs: Ensure your AI endeavors have measurable impacts on business outcomes.
- Select an AI Solution: Choose tools that align with your needs and provide customization.
- Implement Gradually: Start with a pilot, gather data, and expand AI usage judiciously.
For AI KPI management advice, connect with us at hello@itinai.com. And for continuous insights into leveraging AI, stay tuned on our Telegram t.me/itinainews or Twitter @itinaicom.
Spotlight on a Practical AI Solution:
Consider the AI Sales Bot from itinai.com/aisalesbot designed to automate customer engagement 24/7 and manage interactions across all customer journey stages.
Discover how AI can redefine your sales processes and customer engagement. Explore solutions at itinai.com.
“`