The article explores a framework called “The Contextual Scaffolds Framework” for effective prompt engineering. It discusses the importance of context in language interpretation and proposes two categories of context scaffolds: expectational context scaffold and operational context scaffold. The framework aims to align user expectations with model capabilities and provides a mental model for prompt crafting. It offers a visual representation and practical examples to illustrate its application. The article also briefly mentions the optimization of context scaffolds and the possibility of finetuning models using this framework.
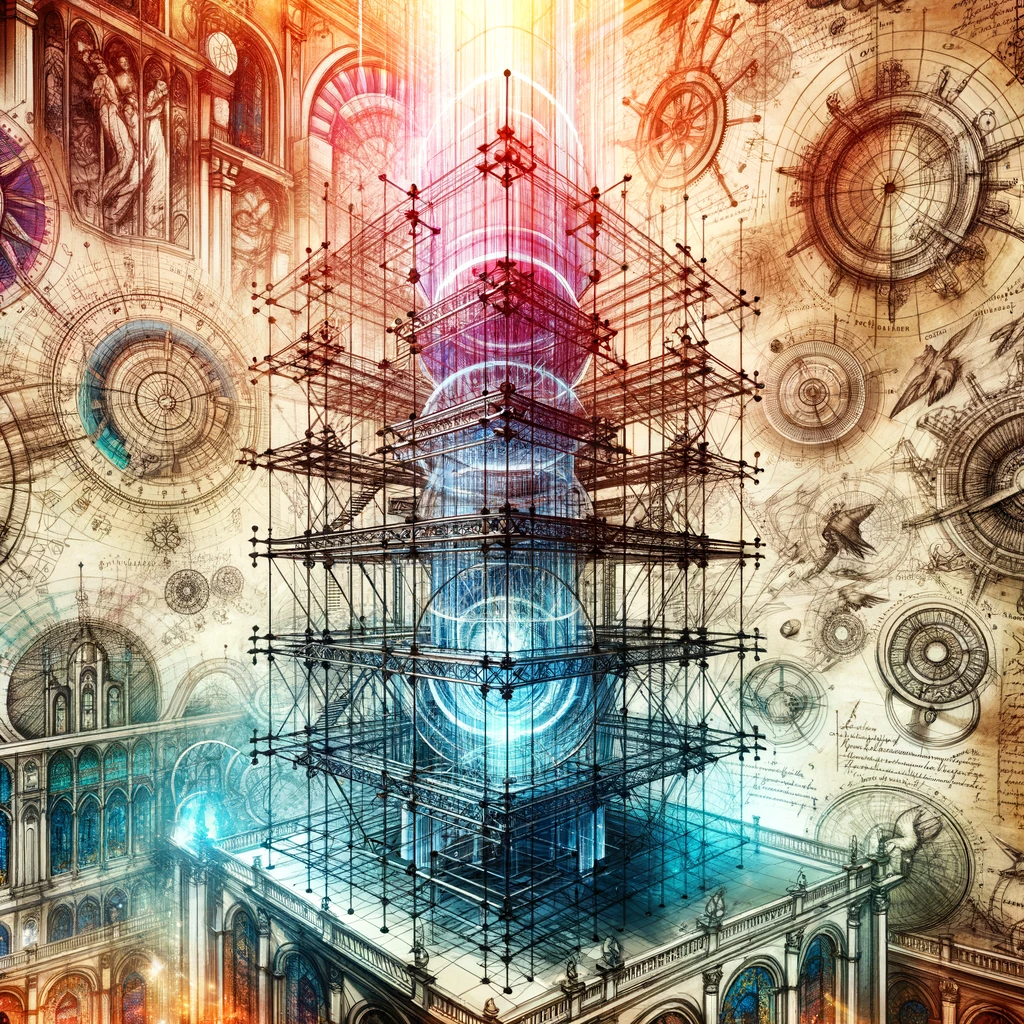
A Universal Roadmap for Prompt Engineering: The Contextual Scaffolds Framework (CSF)
Introduction
In this article, we explore a new approach for prompt engineering called the Contextual Scaffolds Framework (CSF). We delve into the nuances of language and propose a universal framework that can be used to craft effective prompts for AI models like ChatGPT-4. This framework takes into account the context of the prompt and provides a practical roadmap for prompt crafting in various scenarios.
Understanding Pragmatics
Pragmatics is a branch of linguistics that examines how context shapes meaning interpretation. Two important concepts in pragmatics are implicature and presupposition. Implicature refers to when a speaker implies something without explicitly stating it, expecting the listener to infer the intended meaning. Presupposition refers to assumptions or shared information between the speaker and listener. These concepts highlight that words have meanings beyond their literal definitions, and understanding the context is key to interpreting their intended meaning.
Large Language Models (LLMs) and “Meaning Auras”
Large Language Models (LLMs) like ChatGPT-4 systemize the production of “meaning auras” in conversations with humans. While these models primarily make word-by-word predictions, they may implicitly model the interplay of meaning auras. This understanding of pragmatics can be useful in prompt engineering and serves as a building block for the CSF.
The Contextual Scaffolds Framework (CSF)
Effective prompt crafting for models like ChatGPT-4 relies on considering both the context of the prompt and the desired model output. The CSF breaks down the context into two categories: the Expectational Context Scaffold and the Operational Context Scaffold. The Expectational Context Scaffold encompasses the user’s goals, intentions, and specific situation details. The Operational Context Scaffold defines how the AI model should operate to fulfill those expectations. By categorizing the context into these scaffolds, the CSF provides a practical and easy-to-use mental model for prompt crafting.
Optimization of Context Scaffolds for Prompt Engineering
When using an LLM, we want the model to produce output that meets or exceeds our expectations. The CSF allows us to optimize the context scaffolds by pinpointing one or more optimal operational contexts that fulfill the expectational context. By representing the context as vectors of attributes and maximizing the utility function, we can find the best operational contexts for a given expectational context.
Conclusion
The CSF provides a universal roadmap for prompt engineering, allowing us to align user expectations with AI model capabilities. By considering the context and optimizing the context scaffolds, we can craft prompts that generate the desired output. Implementing AI solutions based on the CSF can redefine your company’s way of work and give you a competitive edge. To explore AI solutions and learn more about leveraging AI for your business, connect with us at hello@itinai.com.