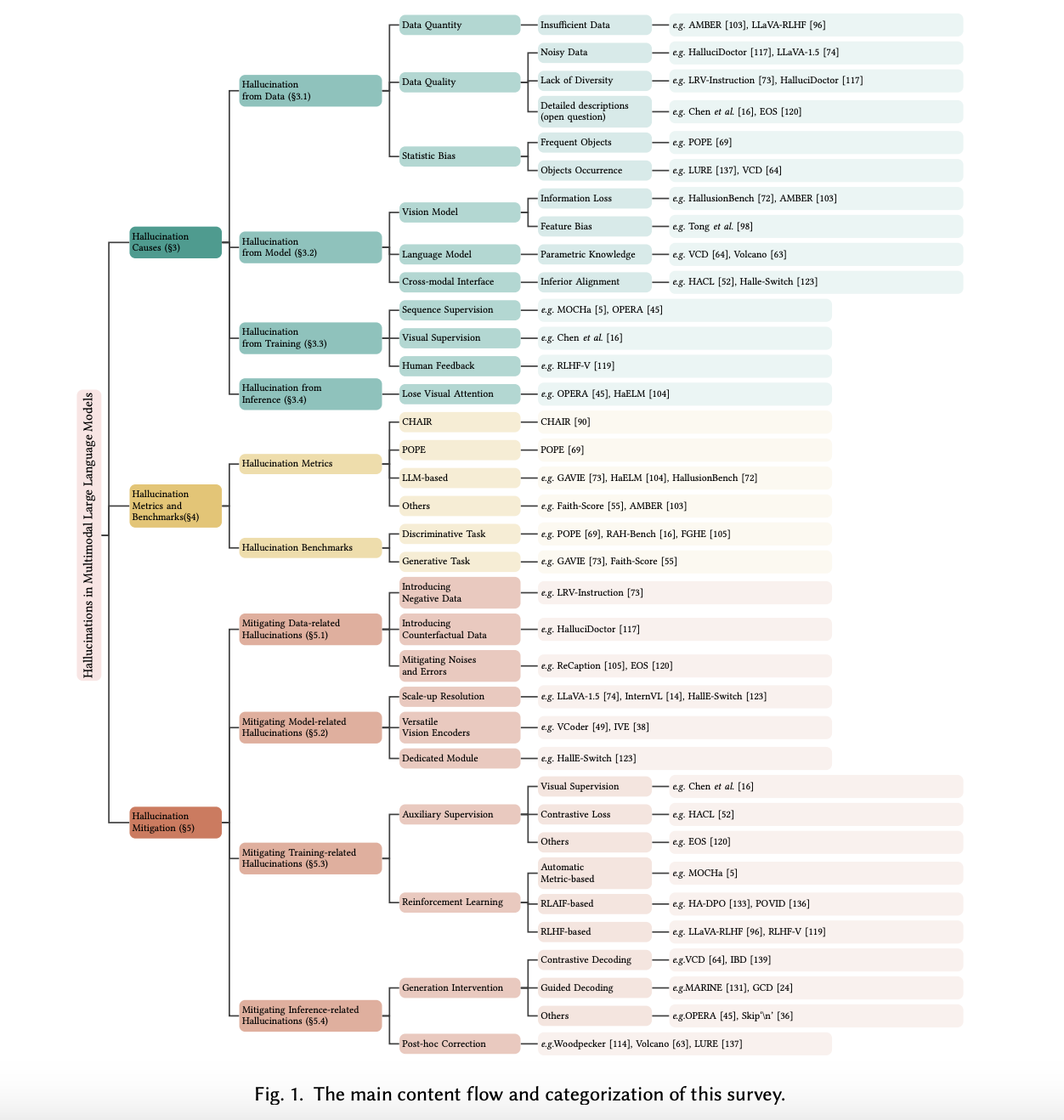
Mitigating Hallucination in Multimodal Large Language Models
Multimodal large language models (MLLMs) blend language processing and computer vision to understand and respond to both text and imagery. They excel at tasks like describing photographs and answering questions about video content, offering practical solutions for real-world challenges.
The Challenge of Hallucination
MLLMs can sometimes generate responses that are plausible but factually incorrect, undermining trust in AI applications. This is especially critical in areas like medical image analysis and surveillance systems.
Proposed Solutions
Researchers have developed new alignment techniques and critically evaluated data quality to enhance the models’ accuracy and reduce hallucination incidents. These approaches have led to significant improvements in benchmark tests, showing a 30% reduction in hallucination incidents and a 25% improvement in answering visual questions.
Advancing AI Capabilities
This research not only addresses the technical aspects of MLLMs but also promises enhanced applicability across various sectors, ensuring AI can accurately interpret and interact with the visual world.
AI Solutions for Your Business
Are you looking to evolve your company with AI? Discover how AI can redefine your way of work by mitigating hallucination in MLLMs. Identify automation opportunities, define KPIs, select suitable AI solutions, and implement them gradually. For AI KPI management advice and continuous insights into leveraging AI, connect with us at hello@itinai.com or stay tuned on Telegram or Twitter.
Practical AI Solution: AI Sales Bot
Consider using the AI Sales Bot from itinai.com/aisalesbot to automate customer engagement 24/7 and manage interactions across the entire customer journey, redefining your sales processes and customer engagement.