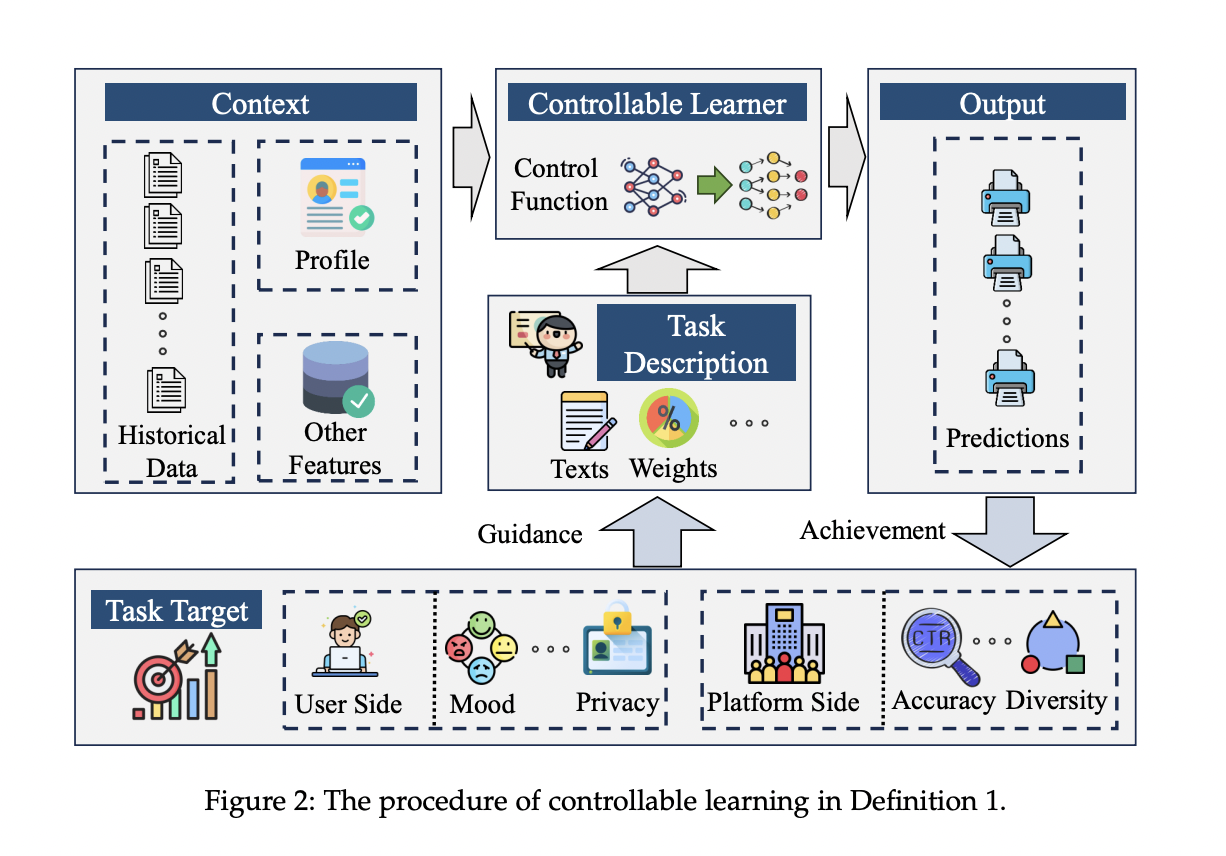
Controllable Learning: Methods, Applications, and Challenges in Information Retrieval
Definition and Importance of Controllable Learning
Controllable Learning (CL) ensures learning models meet predefined targets and adapt to changing requirements without retraining, enhancing reliability and effectiveness.
Taxonomy of Controllable Learning
The CL taxonomy categorizes who controls the learning process, what aspects are controllable, how control is implemented, and where power is applied.
User-Centric Control
User-centric control empowers users to shape their recommendation experience actively by modifying user profiles, interactions, and preferences.
Platform-Mediated Control
Platform-mediated control involves algorithmic adjustments and policy-based constraints imposed by the platform to enhance the recommendation process.
Implementation Techniques in Controllable Learning
Techniques include Rule-Based Techniques, Pareto Optimization, and Hypernetwork, which enhance adaptability and performance across various tasks and domains.
Applications in Information Retrieval
Controllable learning in IR ensures that learning models can dynamically adjust to different task descriptions, providing personalized and relevant search results without extensive retraining.
Conclusion
Controllable learning plays a critical role in ensuring trustworthy and adaptable machine learning systems, providing a comprehensive overview of methods, applications, and challenges.