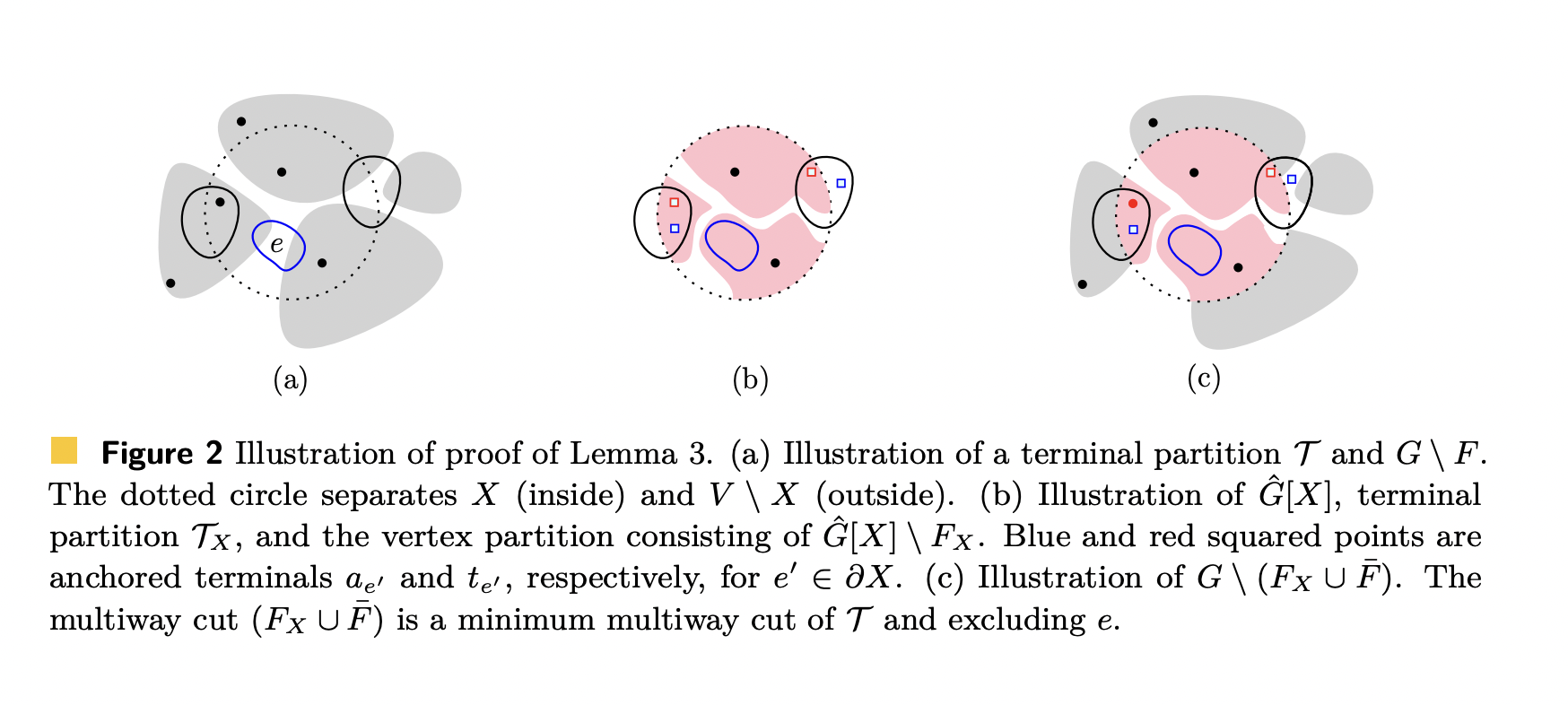
Practical Solutions and Value of Multicut-Mimicking Networks for Hypergraphs
Graph Sparsification and Its Relevance
Graph sparsification is crucial in reducing graph size without losing key properties. Hypergraphs offer more accurate modeling than normal graphs, leading to new algorithms addressing unique complexities.
Challenges in Graph Sparsification
Research tackles problems like mimicking network sizes and multicut-mimicking networks. New approaches aim to preserve cut sizes efficiently, especially for hypergraphs.
Proposed Method for Hypergraphs
Researchers introduce a method for minimal multicut-mimicking network in hypergraphs, using expander decomposition. This approach effectively handles challenges of hypergraph structures, enabling smaller and efficient networks.
Advancements and Future Focus
The study shows significant progress in hypergraph sparsification, introducing parameter c for network minimization. Future work aims to reduce complexity further, exploring near-linear time frameworks for network computation.
Engaging with AI Solutions
To leverage AI effectively, identify automation opportunities, define measurable KPIs, select suitable AI tools, and implement gradually. For AI KPI management advice and insights into AI utilization, connect with us at Itinai.
For more details on the research, visit the original post on MarkTechPost.