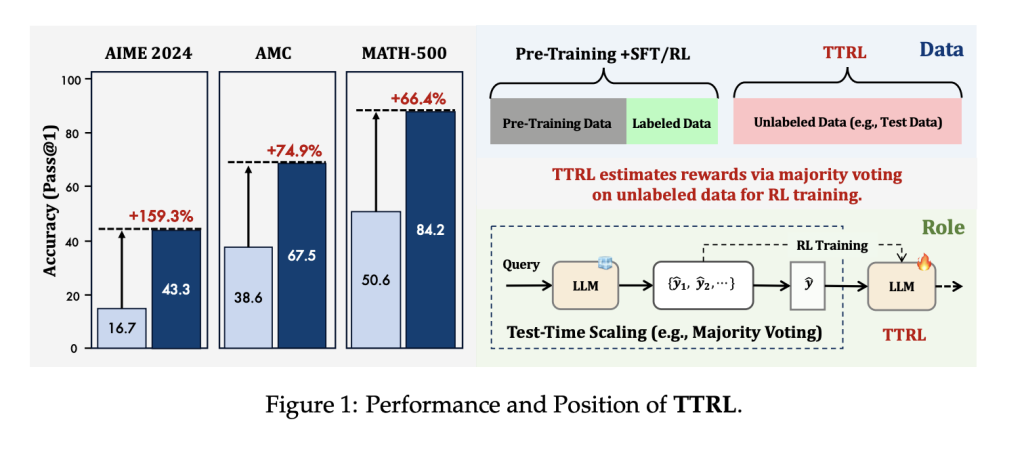
Innovative Approaches in AI: Test-Time Reinforcement Learning
Introduction
Recent advancements in artificial intelligence, particularly in large language models (LLMs), have highlighted the need for models that can learn without relying on labeled data. Researchers from Tsinghua University and Shanghai AI Lab have introduced a groundbreaking approach known as Test-Time Reinforcement Learning (TTRL), which allows LLMs to adapt and improve using only unlabeled data.
Understanding the Need for TTRL
Despite the progress made in enhancing reasoning capabilities through reinforcement learning (RL), most LLMs still depend heavily on supervised data. Traditional methods, such as Reinforcement Learning from Human Feedback (RLHF), require extensive human input and labeled datasets. As LLMs are increasingly utilized in dynamic environments—ranging from education to scientific research—they must generalize beyond their initial training data.
Challenges in Current Models
Existing models often struggle with performance gaps when faced with new reasoning tasks or distribution shifts. Techniques like Test-Time Scaling (TTS) and Test-Time Training (TTT) have been proposed to address these issues, but the lack of reliable reward signals during inference remains a significant challenge.
Introducing Test-Time Reinforcement Learning (TTRL)
TTRL is a novel framework that enables LLMs to learn during inference using only unlabeled test data. This method leverages the intrinsic capabilities of pre-trained language models to generate pseudo-rewards through majority voting on multiple outputs.
How TTRL Works
- Label Estimation via Majority Voting: For each input prompt, the model generates multiple outputs. The most frequent response is considered the estimated label.
- Reward Assignment and Policy Optimization: A binary reward is assigned based on whether each generated response aligns with the estimated label. The model is then updated using gradient-based RL algorithms to enhance agreement with these pseudo-labels.
This two-stage approach is straightforward and compatible with standard RL methods, providing sufficient learning signals even without ground-truth labels.
Empirical Findings and Case Studies
TTRL was tested on three mathematical reasoning benchmarks: AIME 2024, AMC, and MATH-500. The results demonstrated significant improvements:
- The Qwen2.5-Math-7B model improved its performance on AIME 2024 from 16.7% to 43.3%, marking a 159.3% increase without any labeled data.
- On average, this model achieved an 84.1% relative gain across all benchmarks.
- Even the smaller Qwen2.5-Math-1.5B model saw an increase from 33.0% to 80.0% on MATH-500.
These findings indicate that TTRL can enhance model performance even in the absence of supervised training signals, suggesting a self-reinforcing learning mechanism that extracts valuable insights from consensus signals.
Broader Implications of TTRL
The implications of TTRL extend beyond mathematical reasoning. The principles of self-estimated supervision and test-time adaptation can be applied across various domains, making it a scalable solution for LLMs facing diverse tasks.
Conclusion
TTRL represents a significant advancement in the application of reinforcement learning to LLMs, enabling continuous adaptation without the need for costly human annotations. This approach not only scales with model size but also demonstrates robustness across different tasks. As LLMs encounter increasingly complex challenges, frameworks like TTRL offer a promising pathway for self-adaptive, label-free learning.