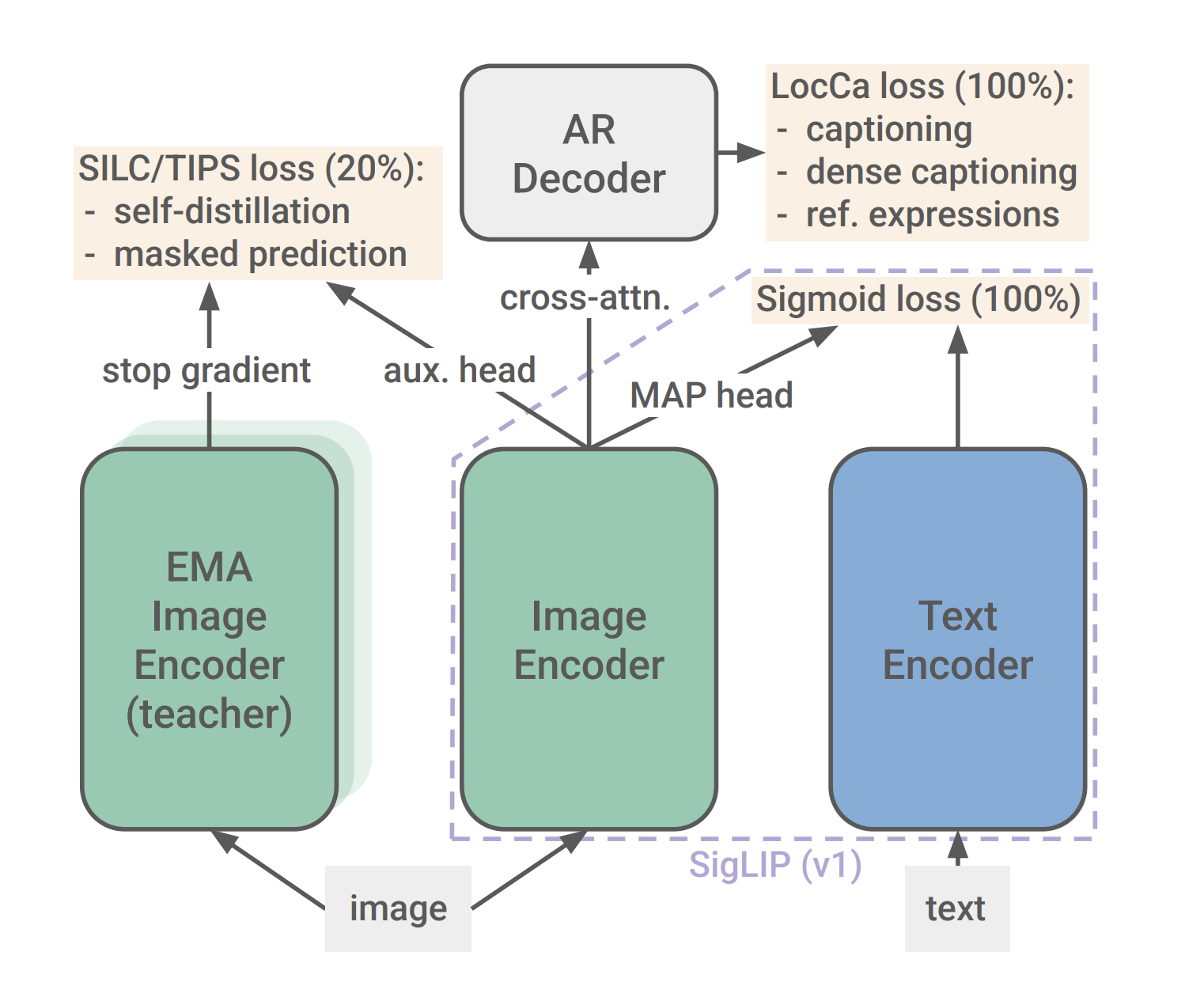
Transforming Business with Advanced AI Solutions
Introduction to Modern Vision-Language Models
Modern vision-language models have significantly changed how visual data is processed. However, they can struggle with detailed localization and dense feature extraction. This is particularly relevant for applications that require precise localization, like document analysis and object segmentation.
Challenges in Current Models
Many traditional models excel in high-level semantic understanding but may lack in detailed spatial reasoning. Additionally, models that primarily use contrastive loss often underperform when fine spatial cues are needed. Addressing these challenges is crucial for developing more effective and socially responsible AI systems.
Introducing SigLIP 2
Google DeepMind Research has introduced SigLIP 2, a new family of multilingual vision-language encoders designed to enhance semantic understanding, localization, and dense feature extraction. This model combines captioning-based pretraining and self-supervised learning approaches to improve performance.
Technical Benefits of SigLIP 2
SigLIP 2 is built on Vision Transformers, allowing users to easily integrate it into existing systems. It uses sigmoid loss to balance the learning of both global and local features, and incorporates a decoder-based loss for tasks like image captioning and region-specific localization.
The model also features a NaFlex variant that supports native aspect ratios, processing images of various resolutions while maintaining their spatial integrity. This is particularly useful in applications such as document understanding and OCR.
Enhanced Performance and Evaluation
Experimental results show that SigLIP 2 outperforms earlier models in zero-shot classification and multilingual image-text retrieval tasks. It demonstrates improved performance in dense prediction tasks, such as semantic segmentation and depth estimation, often reporting higher scores than previous models.
Additionally, the model shows reduced biases in representation, thanks to effective de-biasing techniques used during training. This ensures fairer associations and a more ethical approach to AI.
Conclusion
SigLIP 2 represents a significant advancement in vision-language models, effectively addressing challenges in localization and multilingual support while ensuring ethical considerations are met. Its robust performance across various tasks makes it a valuable addition to the AI research community and a practical solution for businesses looking to enhance their operations.
Next Steps for Businesses
- Explore how AI technology can transform your workflows.
- Identify processes that can be automated to add value in customer interactions.
- Establish key performance indicators (KPIs) to measure the impact of AI investments.
- Select customizable tools that align with your business objectives.
- Start with small AI projects, evaluate their effectiveness, and gradually scale up.
Contact Us
If you need guidance on integrating AI into your business, feel free to contact us at hello@itinai.ru or reach out via Telegram, X, or LinkedIn.
“`