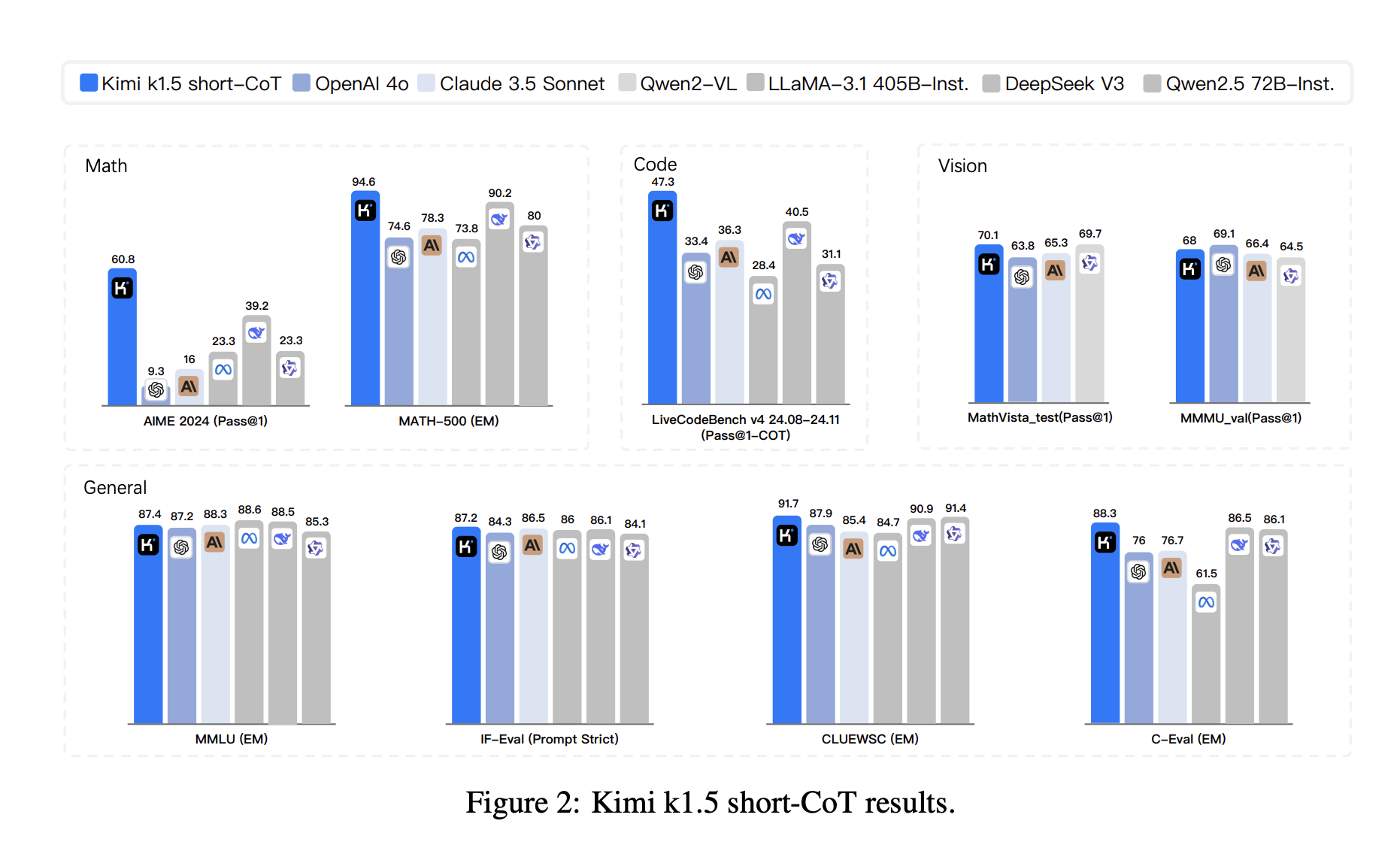
Reinforcement Learning (RL) in AI
Reinforcement Learning (RL) has revolutionized AI by enabling models to improve through interaction and feedback. When applied to large language models (LLMs), RL enhances their ability to tackle complex tasks like math problem-solving, coding, and data interpretation. Traditional models often rely on fixed datasets, which limits their effectiveness in dynamic environments.
Challenges in LLM Development
A key challenge is scaling LLMs while ensuring they are computationally efficient. Conventional training methods struggle with tasks that require deep reasoning. Current RL implementations for LLMs often fall short due to issues in prompt design, policy optimization, and data management. This gap highlights the need for a new approach that aligns model training with specific tasks, while also being efficient with token usage.
Innovative Solutions
Previous methods to enhance LLMs included supervised fine-tuning and techniques like chain-of-thought (CoT) prompting, which helps models break down complex problems. However, these methods can be resource-intensive and limited by context size. The absence of scalable RL frameworks has hindered advancements, indicating a need for a fresh approach.
Kimi k1.5: A Breakthrough Model
Researchers from the Kimi Team have developed Kimi k1.5, a next-generation multimodal LLM that combines RL with extended context capabilities. This model features:
- Long-context scaling: Supports a context window of 128,000 tokens, allowing for effective processing of larger problems.
- Streamlined RL framework: Avoids complex methods, focusing on efficient training and adaptability.
Two Model Variants
Kimi k1.5 comes in two versions:
- Long-CoT Model: Excels in extended reasoning tasks, achieving impressive scores like 96.2% on MATH500.
- Short-CoT Model: Optimized for efficiency, maintaining high performance while reducing token usage.
Key Innovations and Benefits
The training process for Kimi k1.5 integrates supervised fine-tuning, long-chain reasoning, and RL, enhancing problem-solving capabilities. Notable innovations include:
- Partial rollouts: Reuses previous computations to boost efficiency.
- Diverse data sources: Enhances the model’s ability to reason across text and images.
- Advanced sampling strategies: Focus training on areas needing improvement.
Performance Highlights
Kimi k1.5 shows remarkable improvements in token efficiency and performance:
- Achieved 96.2% accuracy on MATH500 and a 94th percentile ranking on Codeforces.
- Outperformed other models like GPT-4o and Claude Sonnet 3.5 in various benchmarks.
Conclusion
Kimi k1.5 addresses the limitations of traditional training methods, setting new standards for performance in reasoning and multimodal tasks. Its dual models showcase the versatility needed for both complex and efficient problem-solving.
Get Involved
Explore the Paper and GitHub Page for more insights. Follow us on Twitter, join our Telegram Channel, and connect with our LinkedIn Group. Join our vibrant ML SubReddit community of over 65k members.
Transform Your Business with Kimi k1.5
Stay competitive by leveraging Kimi k1.5 to redefine your operations:
- Identify Automation Opportunities: Find key interactions that AI can enhance.
- Define KPIs: Ensure measurable impacts on your business.
- Select an AI Solution: Choose tools that fit your needs.
- Implement Gradually: Start with a pilot project and expand wisely.
For AI KPI management advice, reach out to us at hello@itinai.com. Stay updated on AI insights via our Telegram or Twitter.