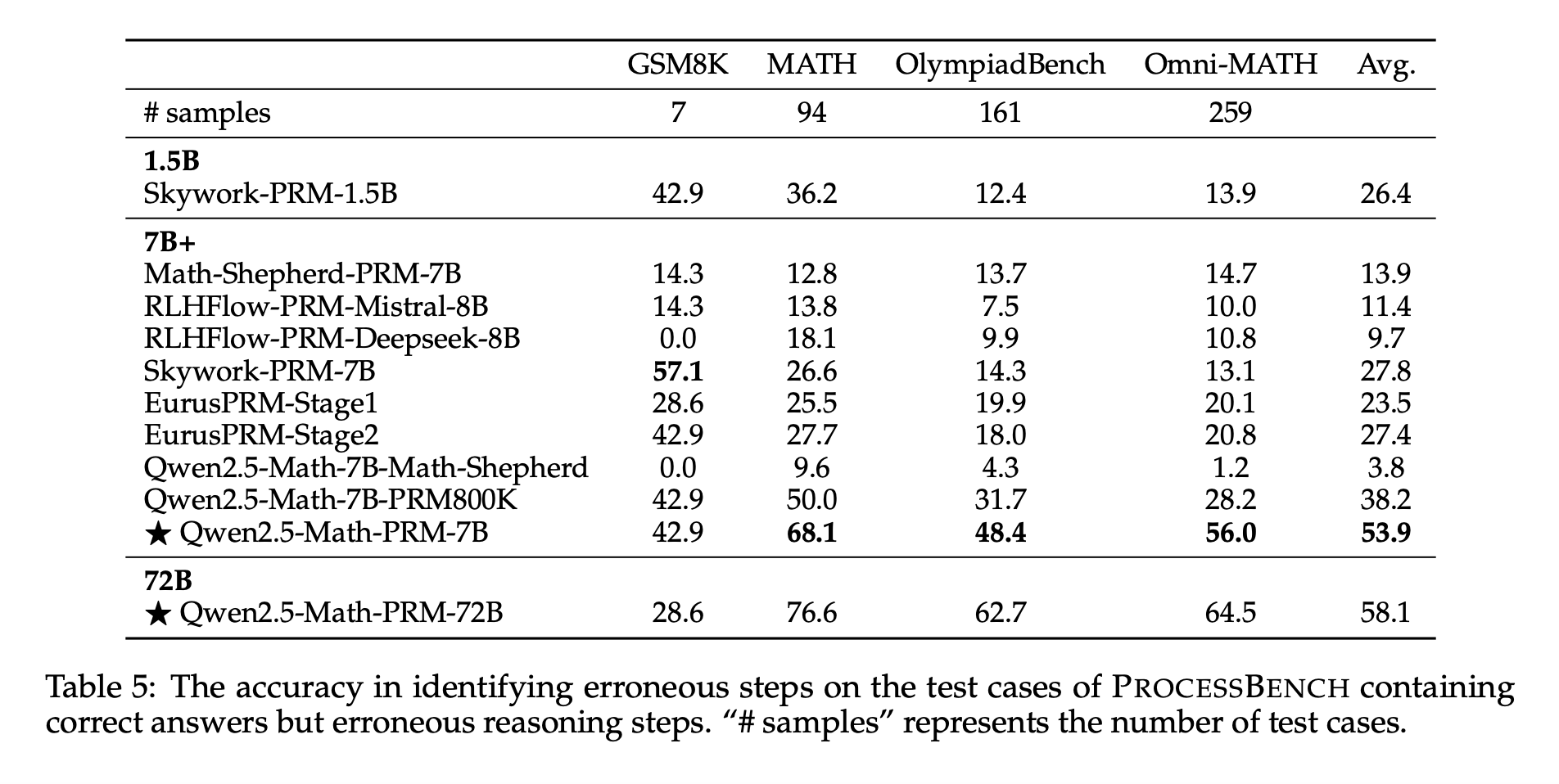
Understanding the Challenges in Mathematical Reasoning for AI
Mathematical reasoning has been a tough hurdle for Large Language Models (LLMs). Mistakes in reasoning steps can lead to inaccurate final results, which is especially crucial in fields like education and science. Traditional evaluation methods, such as the Best-of-N (BoN) strategy, often miss the complexities of reasoning. This has prompted the creation of Process Reward Models (PRMs) to offer better supervision by assessing the correctness of each reasoning step. However, developing effective PRMs is challenging due to issues in data annotation and evaluation methods.
Recent Innovations by Alibaba Qwen Team
The Alibaba Qwen Team has introduced two new PRMs, with 7B and 72B parameters, as part of their Qwen2.5-Math-PRM series. These models enhance existing PRM frameworks and utilize innovative techniques to improve reasoning accuracy and generalization.
Key Features of the Qwen2.5-Math-PRM Models
The Qwen team’s approach combines Monte Carlo (MC) estimation with a unique “LLM-as-a-judge” method. This hybrid technique improves the quality of step-by-step annotations, making it easier to spot and correct errors in mathematical reasoning.
Technical Innovations and Benefits
- Consensus Filtering: This method only keeps data where both MC estimation and LLM-as-a-judge agree on the correctness of steps, reducing noise in training.
- Hard Labeling: Verified deterministic labels help the model differentiate between valid and invalid reasoning steps.
- Efficient Data Utilization: By combining MC estimation with LLM-as-a-judge, the models ensure high-quality data, enabling effective PRMs even with smaller datasets.
Impressive Results
The Qwen2.5-Math-PRM models have shown excellent results on benchmarks like PROCESSBENCH. For instance, the Qwen2.5-Math-PRM-72B model achieved an F1 score of 78.3%, outperforming many open-source models and even proprietary ones like GPT-4-0806. The consensus filtering method significantly improved training quality, cutting down data noise by about 60%.
Shift in Evaluation Approach
The Qwen2.5-Math-PRM series emphasizes evaluating each step rather than just focusing on final outcomes. This adjustment addresses limitations found in earlier models, providing a more accurate reasoning process.
Conclusion
The Qwen2.5-Math-PRM models mark a significant advancement in mathematical reasoning for LLMs. By tackling challenges in PRM development, the Alibaba Qwen Team offers a practical framework for enhancing reasoning accuracy and reliability. These models not only surpass existing alternatives but also pave the way for future research in AI reasoning.
Stay Connected
Explore the paper and models on Hugging Face. Follow us on Twitter, join our Telegram Channel, and connect with us on LinkedIn. Don’t forget to join our 65k+ ML SubReddit!
Enhance Your Business with AI
To remain competitive, consider how AI can transform your operations:
- Identify Automation Opportunities: Find key customer interactions that can benefit from AI.
- Define KPIs: Ensure your AI projects have measurable impacts.
- Select an AI Solution: Choose tools that fit your needs and allow customization.
- Implement Gradually: Start with a pilot, collect data, and expand AI usage thoughtfully.
For AI KPI management advice, contact us at hello@itinai.com. For ongoing insights into leveraging AI, follow us on Telegram or Twitter.