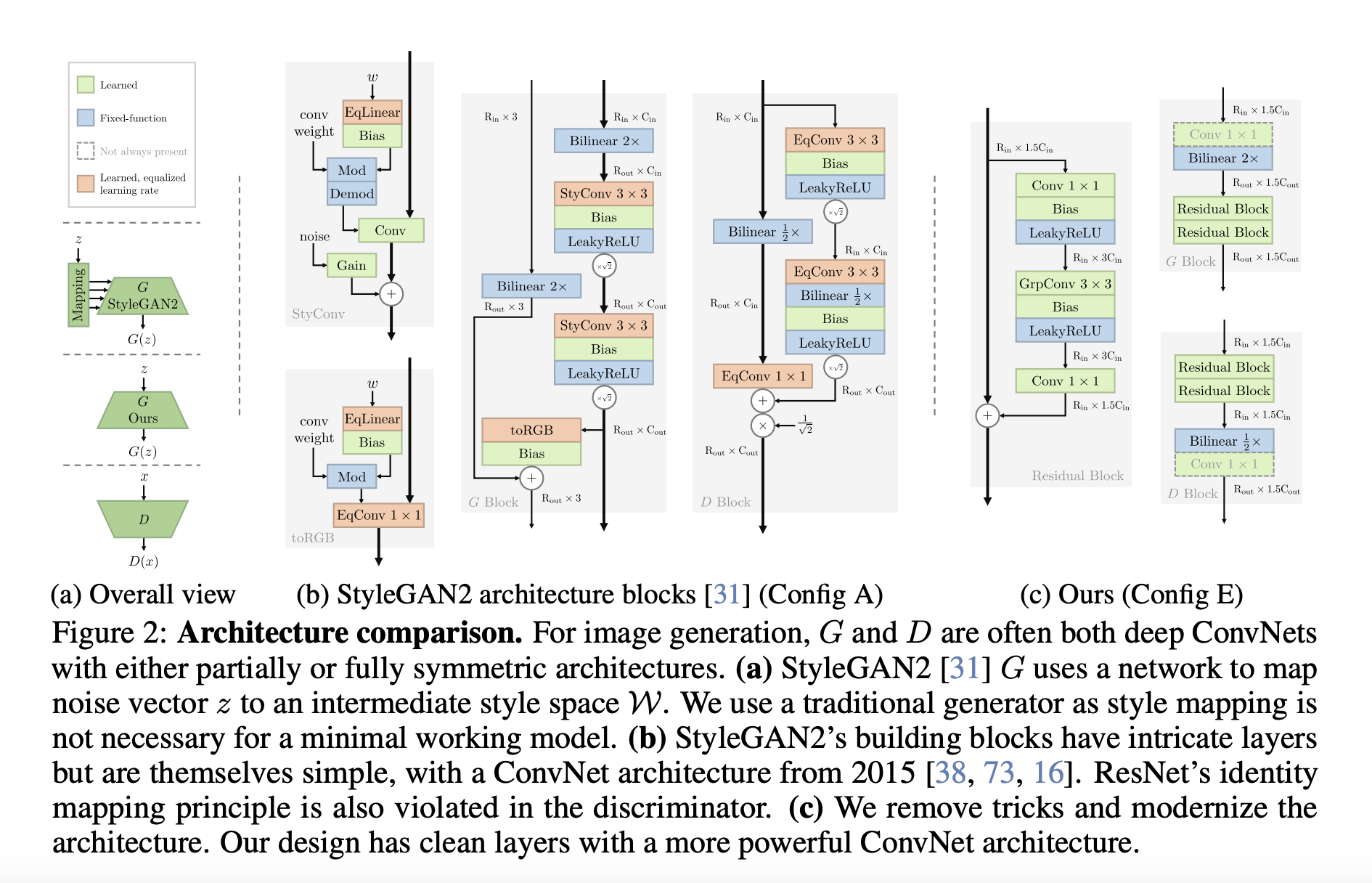
Understanding R3GAN: A Simplified and Stable GAN Model
Challenges with Traditional GANs
GANs (Generative Adversarial Networks) often face training difficulties due to complex architectures and optimization challenges. They can generate high-quality images quickly, but their original training methods can lead to instability and issues like mode collapse. Although some models, like StyleGAN, use various techniques to improve performance, they still lack a strong theoretical foundation and can be less effective than newer models.
Introducing R3GAN
Researchers from Brown and Cornell Universities have developed R3GAN, a modern GAN that simplifies training without relying on numerous tricks. By using a new loss function that stabilizes training and improves convergence, R3GAN outperforms older models like StyleGAN2 while maintaining fewer complexities. This model incorporates advanced design elements to enhance performance across various datasets.
Key Features of R3GAN
– **Balanced Stability and Diversity**: R3GAN addresses traditional GAN issues, such as mode collapse, by evaluating fake samples against real ones, ensuring better coverage of data types.
– **Enhanced Training Stability**: The model uses gradient penalties to ensure stable training and convergence, leading to improved results in experiments.
– **Minimalist Design**: R3GAN removes unnecessary components from StyleGAN2, focusing on essential features to create a powerful yet efficient architecture.
Performance Highlights
– On the FFHQ-256 dataset, R3GAN achieved an FID score of 7.05, surpassing StyleGAN2.
– It demonstrated perfect mode recovery on StackedMNIST, with minimal KL divergence.
– R3GAN consistently outperformed previous GANs on CIFAR-10, FFHQ-64, and ImageNet, achieving lower FID scores with faster inference times.
Conclusion
R3GAN represents a significant advancement in GAN technology, providing a streamlined and effective model for image generation. While it may not be optimized for specific tasks like image editing, it offers a solid foundation for future research. However, it is essential to consider its limitations, including scalability and ethical concerns regarding generative models.
Get Involved
– Check out the full research paper and GitHub page for more details.
– Follow us on Twitter, join our Telegram Channel, and become part of our LinkedIn Group.
– Join our growing ML community on Reddit with over 65k members.
Boost Your Business with AI
Explore how R3GAN can enhance your company’s AI capabilities. Key steps include:
– **Identify Automation Opportunities**: Find areas in customer interactions that can benefit from AI.
– **Define KPIs**: Ensure measurable impacts from your AI initiatives.
– **Select the Right AI Solution**: Choose tools that fit your needs and allow for customization.
– **Implement Gradually**: Start small, gather data, and expand wisely.
For AI management advice, contact us at hello@itinai.com. Stay updated on AI insights through our Telegram and Twitter channels. Discover how AI can transform your sales processes and customer engagement at itinai.com.