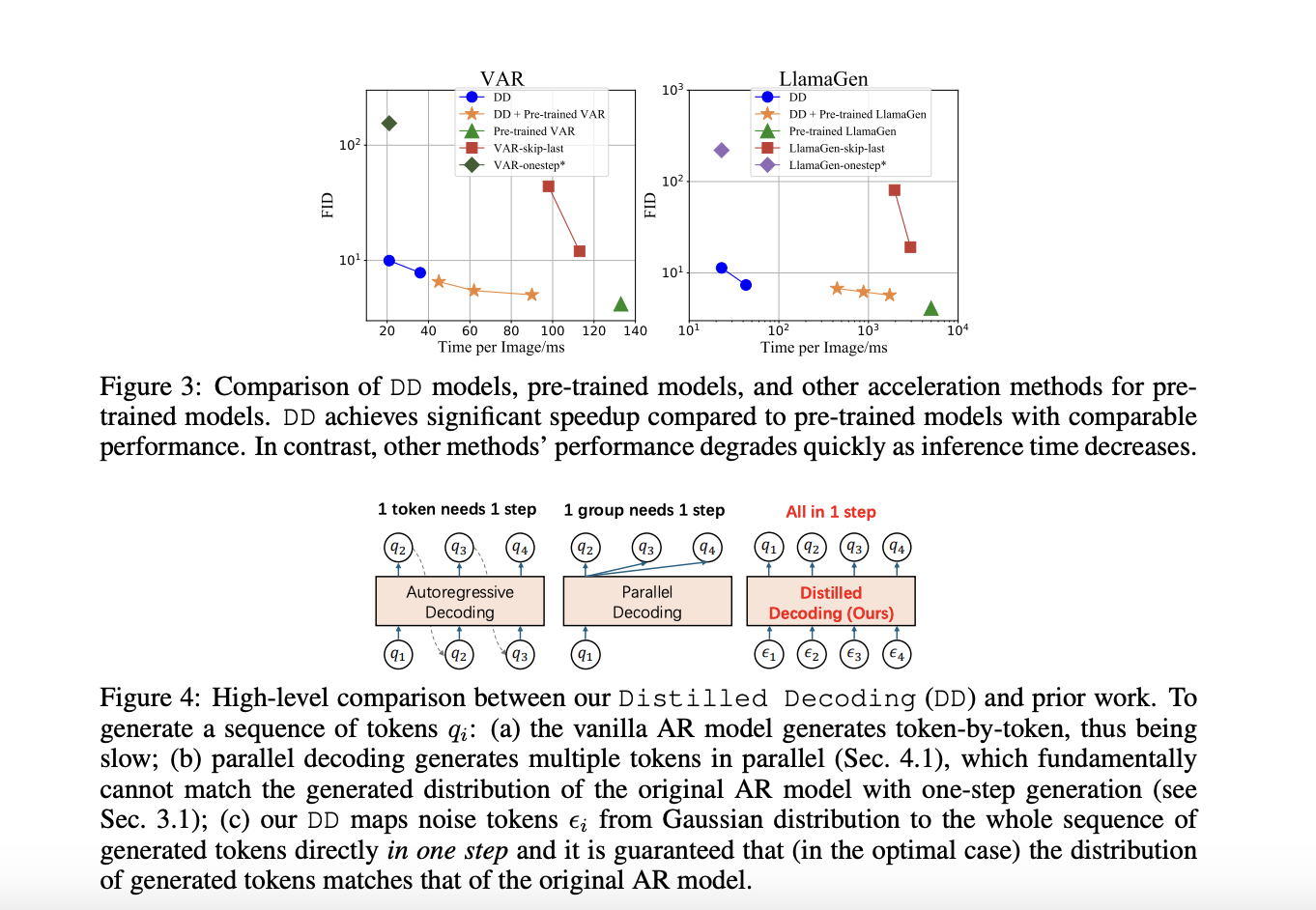
Transforming Image Generation with Distilled Decoding
Key Innovations in Autoregressive (AR) Models
Autoregressive models are revolutionizing image generation by creating high-quality visuals in a step-by-step process. They generate each part of an image based on previously created parts, leading to impressive realism and coherence. These models are widely used in various fields such as computer vision, gaming, and content creation.
The Challenge of Speed
However, a major drawback of AR models is their speed. The sequential generation means each new token has to wait for the previous one to finish, causing delays. For instance, generating a 256×256 image can take around five seconds with traditional AR models. This slow speed limits their use in applications where quick results are essential.
Efforts to Improve Speed
Researchers are exploring ways to speed up AR models, including generating multiple tokens at once and using masking strategies. While these methods can reduce time, they often compromise image quality.
Introducing Distilled Decoding (DD)
Researchers from Tsinghua University and Microsoft have developed a groundbreaking solution called Distilled Decoding (DD). This innovative approach allows for generating images in just one or two steps instead of hundreds, while still maintaining high-quality output. On ImageNet-256, DD achieved a remarkable 6.3x speed increase for VAR models and an astonishing 217.8x for LlamaGen.
How Distilled Decoding Works
DD utilizes a process called flow matching, which connects random noise to the final image in a deterministic way. This method creates a lightweight network that can quickly produce high-quality images without needing original model training data, making it suitable for real-world applications.
Key Benefits of Distilled Decoding
- Speed: Reduces generation time significantly, achieving up to 217.8x faster results.
- Quality: Maintains image quality, with manageable increases in FID scores.
- Flexibility: Offers one-step, two-step, or multi-step generation options based on user needs.
- No Original Data Required: Can be deployed without needing access to original AR model training data.
- Wide Applicability: Potential for use in various AI applications beyond image generation.
Conclusion
With Distilled Decoding, researchers have tackled the speed-quality trade-off in AR models, enabling swift and effective image generation. This advancement paves the way for real-time applications and further innovations in generative modeling.
Get in Touch
If you’re looking to leverage AI to enhance your business, consider adopting Distilled Decoding methods. For more insights and support, connect with us via email or follow us on Telegram and @Twitter.
For more details, explore the Paper and GitHub Page. And don’t forget to join our community on LinkedIn and our 60k+ ML SubReddit.
Discover how AI can reshape your processes at itinai.com.