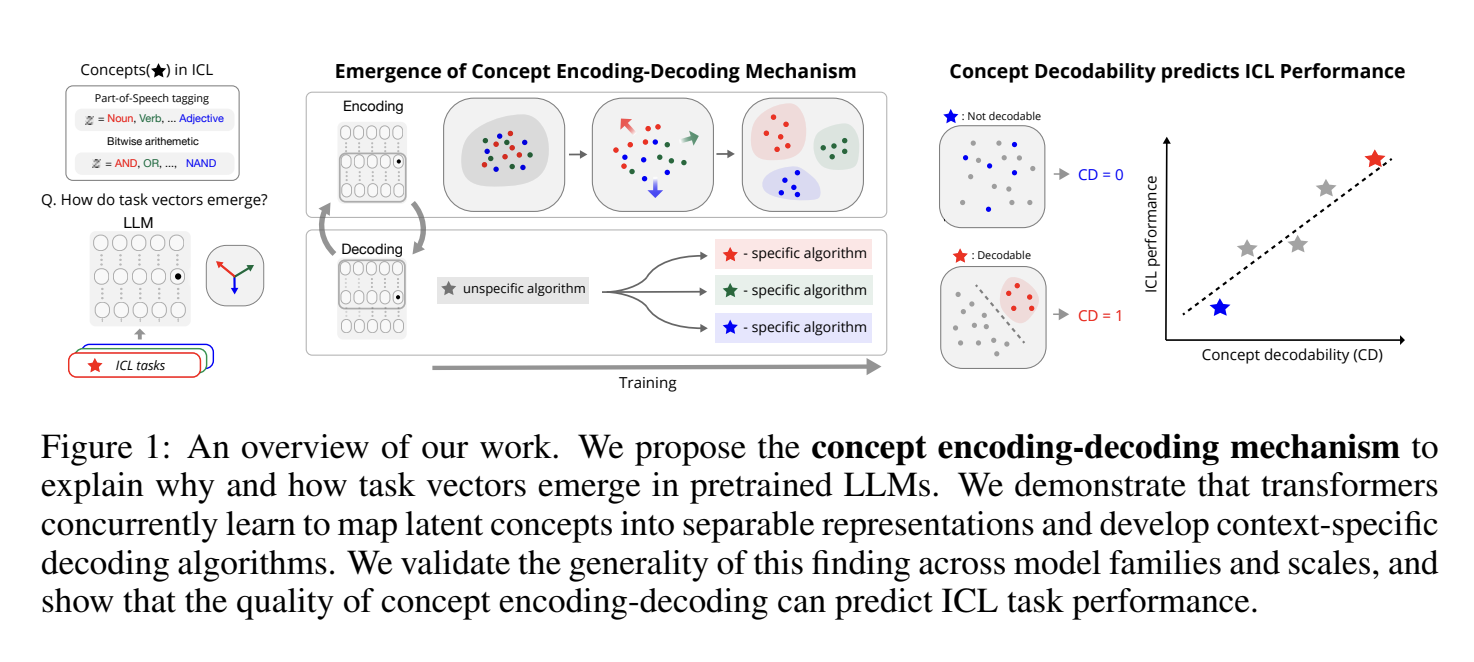
Understanding Large Language Models (LLMs)
Large Language Models (LLMs) show remarkable similarities to how humans think and learn. They can adapt to new situations and understand complex ideas, much like we do with concepts in physics and mathematics. These models can learn from examples without needing changes in their core settings, indicating they create internal representations similar to our mental models.
Key Insights from Research
Recent studies have explored how LLMs represent concepts and perform tasks. Researchers have proposed various frameworks to understand how these models learn from context. One major approach uses a Bayesian framework, which suggests a method for estimating probabilities and applying algorithms based on learned concepts.
Concept Encoding-Decoding Mechanism
Researchers from MIT and Improbable AI introduced a concept encoding-decoding mechanism. This explains how LLMs develop internal representations as they learn. For example, a small transformer trained on linear regression tasks showed that it can separate different concepts into distinct areas of understanding. This mechanism helps models create specific algorithms based on the learned concepts.
Practical Applications and Value
Understanding how LLMs work can help businesses implement AI effectively:
- Identify Automation Opportunities: Find areas where AI can improve customer interactions.
- Define KPIs: Set measurable goals for AI projects to track their impact.
- Select an AI Solution: Choose tools that fit your needs and allow for customization.
- Implement Gradually: Start with pilot projects, gather data, and expand usage wisely.
Stay Connected
For more insights into leveraging AI, follow us on Twitter, join our Telegram Channel, and connect on LinkedIn. If you have questions about AI KPI management, email us at hello@itinai.com.
Discover More
Explore how AI can transform your sales processes and improve customer engagement. Visit itinai.com for more solutions.