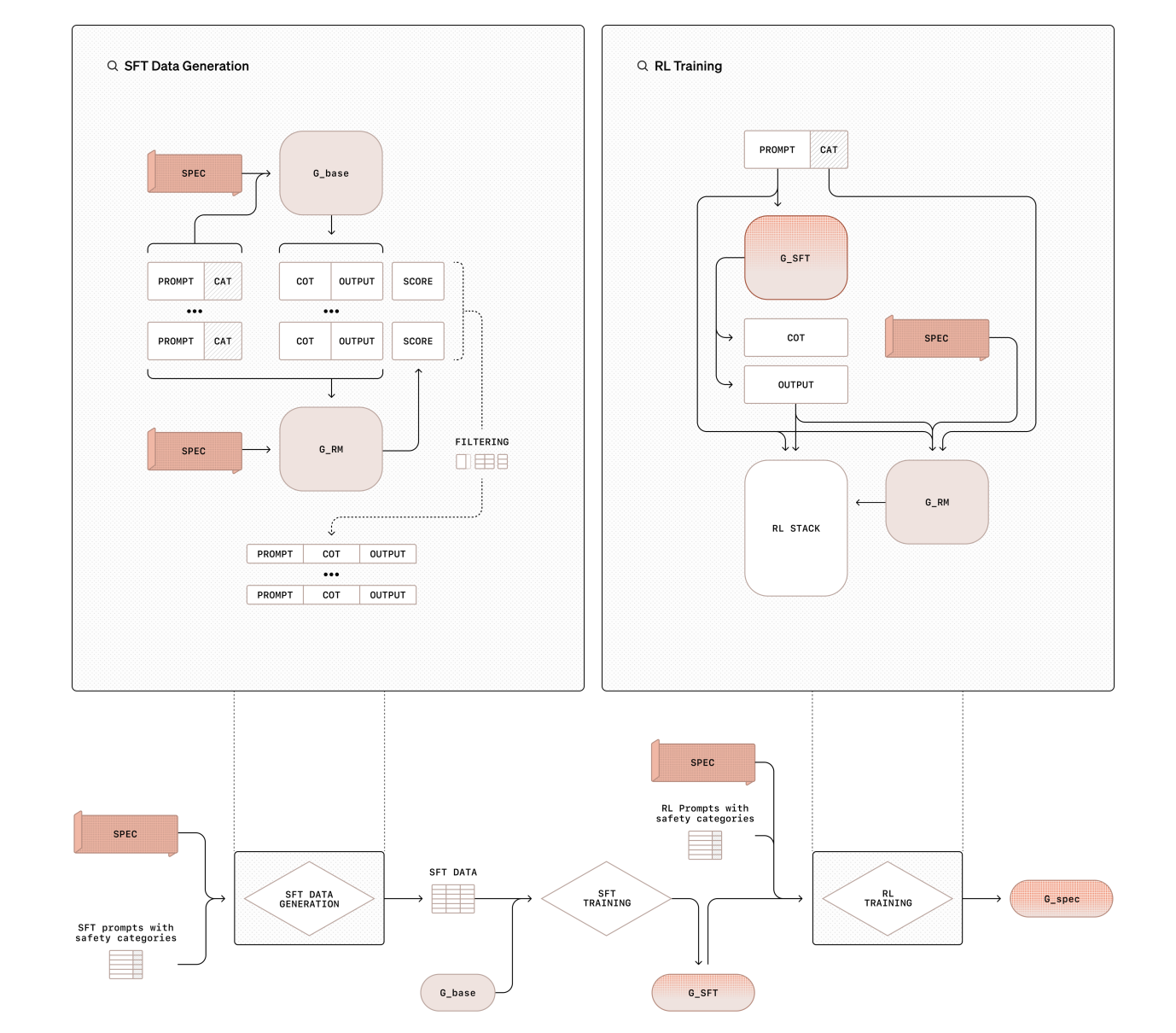
Understanding Deliberative Alignment in AI
Challenge in AI Safety
The use of large-scale language models (LLMs) in critical areas raises a key issue: ensuring they follow ethical and safety guidelines. Current methods like supervised fine-tuning (SFT) and reinforcement learning from human feedback (RLHF) have limitations. These models can still create harmful content, deny valid requests, or struggle with new situations. This is often because they learn from data indirectly rather than directly understanding safety standards.
Introducing Deliberative Alignment
OpenAI researchers have developed **Deliberative Alignment**, a new method that teaches models safety rules directly. This approach helps models think about these rules before giving responses. By focusing on safety during the reasoning process, Deliberative Alignment improves the models’ ability to handle complex situations. Instead of relying on human-annotated data, it uses model-generated data and chain-of-thought (CoT) reasoning for better safety outcomes. This method has shown improved resistance to security threats and fewer refusals of valid requests.
How Deliberative Alignment Works
Deliberative Alignment uses a two-step training process:
1. **Supervised Fine-Tuning (SFT)**: Models learn to refer to and think through safety guidelines using data generated from base models. This builds a solid understanding of safety principles.
2. **Reinforcement Learning (RL)**: The model’s reasoning is fine-tuned using a reward system that evaluates its performance against safety standards. This process does not depend on human-created data, making it more efficient.
By using synthetic data and CoT reasoning, this method prepares models to tackle ethical dilemmas more accurately and effectively.
Results and Benefits
Deliberative Alignment has significantly improved the performance of OpenAI’s models. For example, the o1 model scored 0.88 on the StrongREJECT benchmark, outperforming others like GPT-4o. It also achieved a 93% accuracy rate on benign prompts, reducing unnecessary refusals. The method enhanced adherence to guidelines for sensitive topics as well. Studies confirm that both SFT and RL stages are crucial for these improvements. The approach also adapts well to varied scenarios, including multilingual inputs.
Conclusion
Deliberative Alignment marks a major step forward in aligning language models with safety principles. By teaching models to reason about safety rules, it provides a clear and scalable solution to complex ethical challenges. The success of the o1 series models demonstrates the potential of this method to enhance safety and reliability in AI systems. As AI capabilities grow, approaches like Deliberative Alignment will be vital in keeping these systems aligned with human values.
Get Involved
Check out the research paper for more details. Follow us on Twitter, join our Telegram Channel, and connect with our LinkedIn Group. Don’t forget to join our 60k+ ML SubReddit community.
Transform Your Business with AI
To stay competitive and leverage AI effectively, consider the following steps:
– **Identify Automation Opportunities**: Find key customer interactions that can benefit from AI.
– **Define KPIs**: Ensure your AI initiatives have measurable impacts on your business.
– **Select an AI Solution**: Choose tools that meet your needs and allow for customization.
– **Implement Gradually**: Start small, gather data, and expand your AI usage wisely.
For AI KPI management advice, contact us at hello@itinai.com. For ongoing insights into AI applications, stay connected through our Telegram channel or Twitter. Discover how AI can enhance your sales processes and customer engagement at itinai.com.