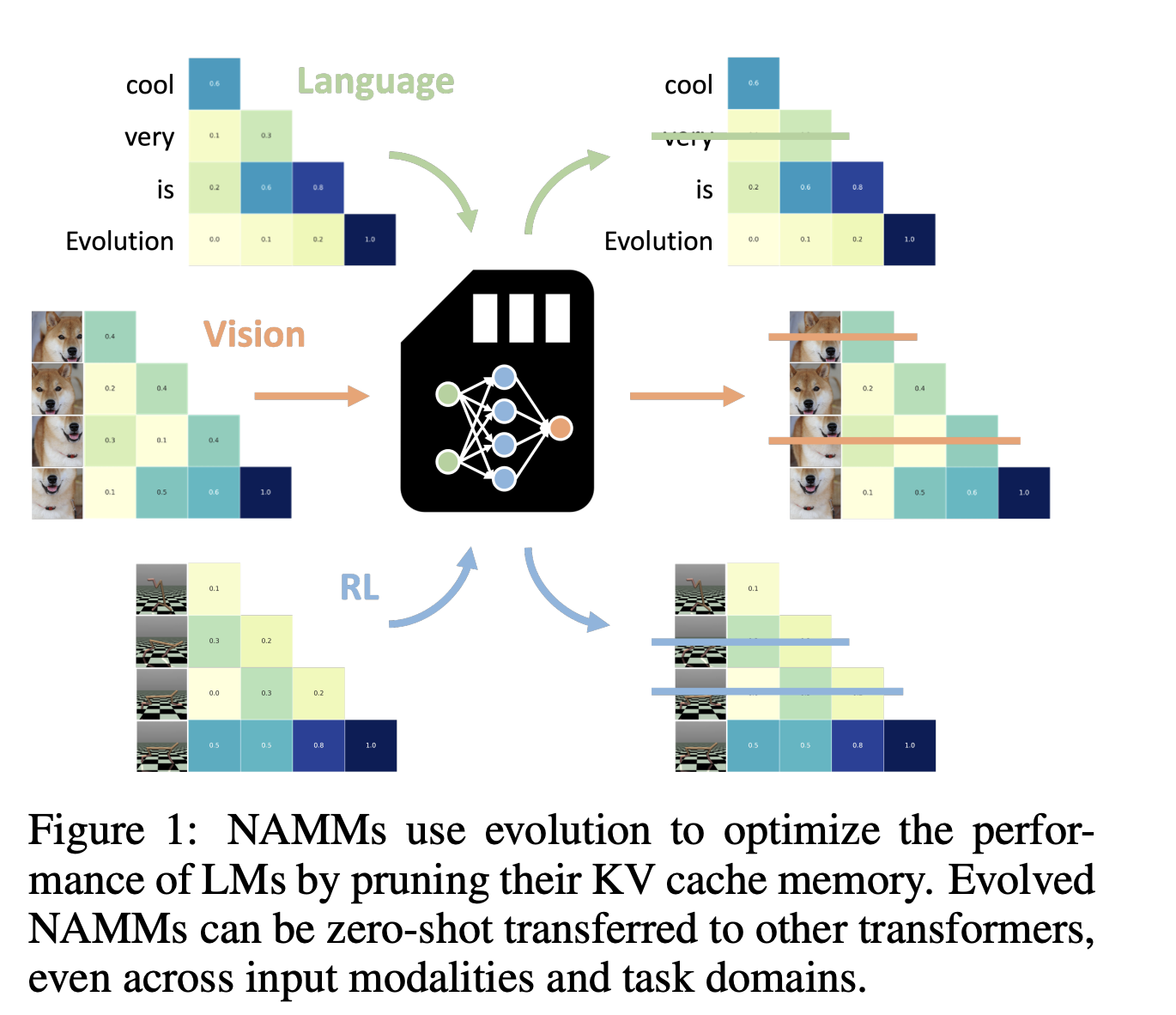
Transformers: The Backbone of Deep Learning
Transformers are essential for deep learning tasks like understanding language, analyzing images, and reinforcement learning. They use self-attention to understand complex relationships in data. However, as tasks grow larger, managing longer contexts efficiently is vital for performance and cost-effectiveness.
Challenges with Long Contexts
One major issue is balancing performance and resource use. Transformers utilize a Key-Value (KV) cache to remember past inputs, but this cache can grow significantly with long tasks, leading to high memory usage. Current methods to reduce cache size often compromise performance by removing important tokens without proper optimization.
Introducing Neural Attention Memory Models (NAMMs)
A research team from Sakana AI in Japan has developed NAMMs, a new approach to enhance memory management in transformers. Unlike traditional methods, NAMMs learn the importance of tokens through evolutionary optimization, making them more efficient and effective.
How NAMMs Work
NAMMs extract features from transformers’ attention matrices using a spectrogram technique. This helps in understanding token importance over time. By applying a lightweight neural network, NAMMs assign scores to tokens, keeping only the most relevant ones in the KV cache while freeing up memory.
Innovative Backward Attention Mechanisms
NAMMs introduce backward attention, allowing for efficient token comparison. This feature helps to retain essential information while discarding redundant data, optimizing memory use across different layers of the transformer.
Proven Performance Improvements
NAMMs have shown impressive results in various benchmarks. For example, on the LongBench benchmark, they improved performance by 11% while reducing the KV cache size to just 25% of the original. In the InfiniteBench test, NAMMs significantly enhanced performance and reduced memory usage, demonstrating their effectiveness for long-context tasks.
Versatility Across Different Tasks
NAMMs have also proven versatile, successfully transferring to various tasks beyond natural language processing, including computer vision and reinforcement learning. They improved performance in long video understanding and decision-making scenarios, showcasing their adaptability and efficiency.
Conclusion: A Step Forward in Memory Management
NAMMs offer a groundbreaking solution for handling long-context processing in transformers. By intelligently managing memory, they boost performance while reducing computational costs. Their broad applicability across different domains indicates significant potential for advancing transformer-based models.
Explore More
For further insights, check out the paper and details of this research. Follow us on Twitter, join our Telegram Channel, and connect with our LinkedIn Group. Also, join our 60k+ ML SubReddit.
Embrace AI for Your Business
If you’re looking to leverage AI for your company, consider these steps:
- Identify Automation Opportunities: Find customer interaction points that can benefit from AI.
- Define KPIs: Ensure your AI initiatives have measurable impacts on business outcomes.
- Select an AI Solution: Choose tools that align with your needs and allow customization.
- Implement Gradually: Start with a pilot project, gather data, and expand AI usage smartly.
For advice on AI KPI management, connect with us at hello@itinai.com. For continuous insights, follow us on Telegram or Twitter.
Redefine Your Sales Processes with AI
Discover how AI can transform your sales and customer engagement strategies at itinai.com.