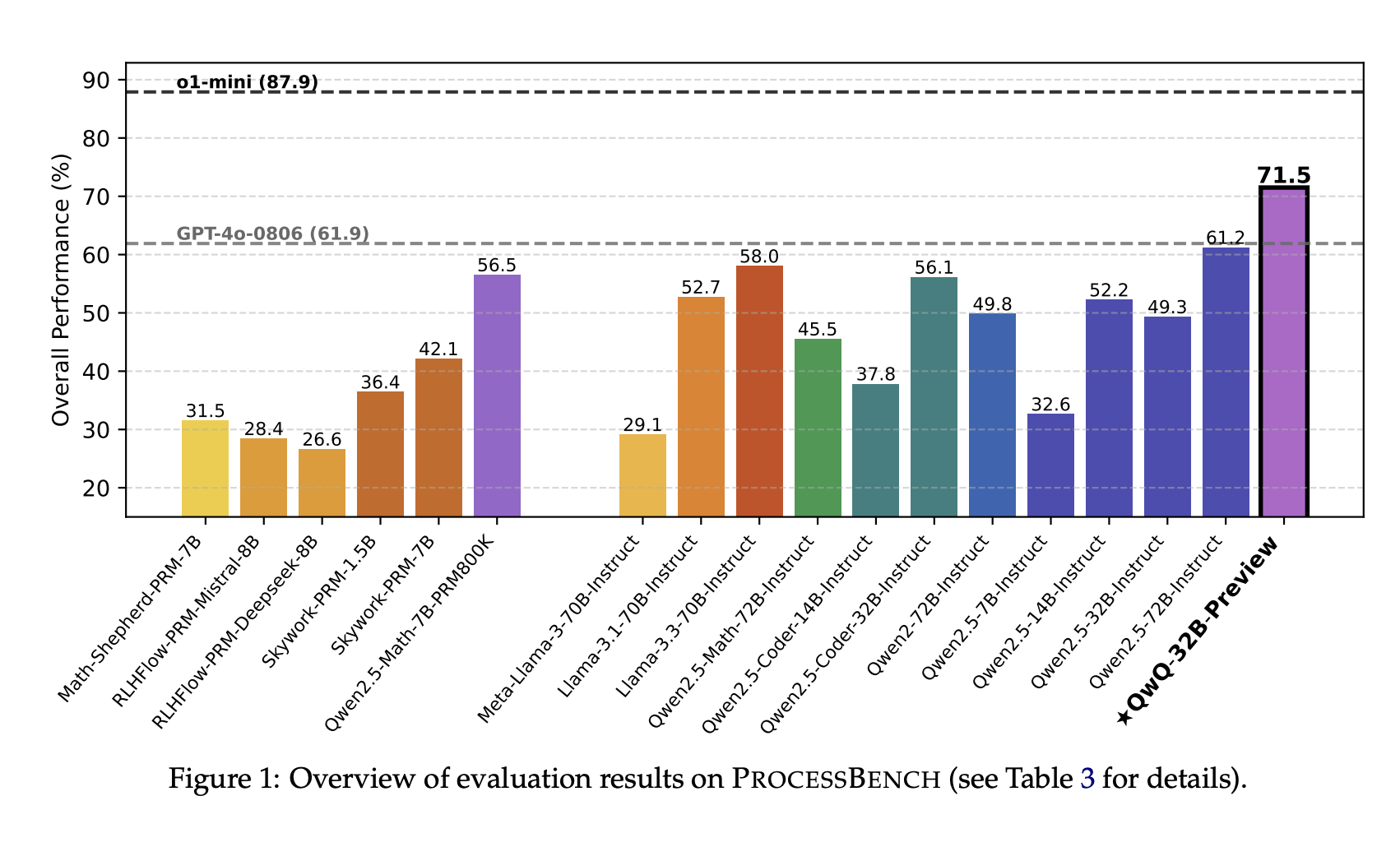
Recent Advances in Language Models
Recent studies show that language models have made significant progress in complex reasoning tasks like mathematics and programming. However, they still face challenges with particularly tough problems. The field of scalable oversight is emerging to create effective supervision methods for AI systems that can match or exceed human performance.
Identifying Errors in Reasoning
Researchers believe language models could automatically spot errors in their reasoning. Yet, current evaluation benchmarks have serious limitations. Some problems are too easy for advanced models, while others only provide simple correct/incorrect answers without detailed feedback. This gap emphasizes the need for better evaluation frameworks to thoroughly assess the reasoning abilities of advanced language models.
New Benchmark Datasets
Several benchmark datasets have been created to evaluate the reasoning processes of language models, each offering unique insights into error detection and solution critique:
- CriticBench: Evaluates models on their ability to critique solutions and correct mistakes across various reasoning areas.
- MathCheck: Uses the GSM8K dataset to create solutions with intentional errors, challenging models to find incorrect reasoning steps.
- PRM800K: Based on MATH problems, it provides detailed annotations for reasoning step correctness, generating interest in process reward models.
Introducing PROCESSBENCH
The Qwen Team and Alibaba Inc. have developed PROCESSBENCH, a benchmark to measure language models’ ability to identify errors in mathematical reasoning. It stands out due to:
- Problem Difficulty: Focuses on high-level competition and Olympiad math problems.
- Solution Diversity: Utilizes various open-source models to generate different solving approaches.
- Comprehensive Evaluation: Includes 3,400 test cases, each carefully annotated by experts for quality and reliability.
Evaluation Protocol
PROCESSBENCH uses a straightforward evaluation method, asking models to find the first error in a solution. This makes it adaptable for various model types, including process reward and critic models, providing a solid framework for assessing error detection capabilities.
Development Process
The creation of PROCESSBENCH involved careful curation of problems, solution generation, and expert annotation. Problems were sourced from established datasets, ensuring a wide range of difficulties. Solutions were generated using multiple models to enhance diversity, and a reformatting method was applied to standardize solution steps for better evaluation.
Key Insights from Evaluation
Evaluation results from PROCESSBENCH revealed important insights:
- As problem complexity increased, all models showed a decline in performance, indicating generalization challenges.
- Existing process reward models performed worse than top prompt-driven critic models, especially on simpler problems.
- Current methodologies for PRMs often struggle with the complexities of mathematical reasoning.
Conclusion and Future Directions
PROCESSBENCH is a groundbreaking benchmark for assessing language models in identifying mathematical reasoning errors. It highlights the need for improved methodologies to enhance AI’s reasoning processes. The research also points to the growing capabilities of open-source models, which are nearing the performance of proprietary models in critical reasoning tasks.
Get Involved
Explore the Paper, GitHub Page, and Data on Hugging Face. Follow us on Twitter, join our Telegram Channel, and connect with our LinkedIn Group. Join our 60k+ ML SubReddit community!
Transform Your Business with AI
Stay competitive by leveraging AI solutions like PROCESSBENCH:
- Identify Automation Opportunities: Find key customer interaction points that can benefit from AI.
- Define KPIs: Ensure your AI initiatives have measurable impacts on business outcomes.
- Select an AI Solution: Choose tools that fit your needs and allow for customization.
- Implement Gradually: Start with a pilot project, gather data, and expand AI usage wisely.
For AI KPI management advice, contact us at hello@itinai.com. For ongoing insights into AI, follow us on Telegram or Twitter.
Discover how AI can enhance your sales processes and customer engagement at itinai.com.