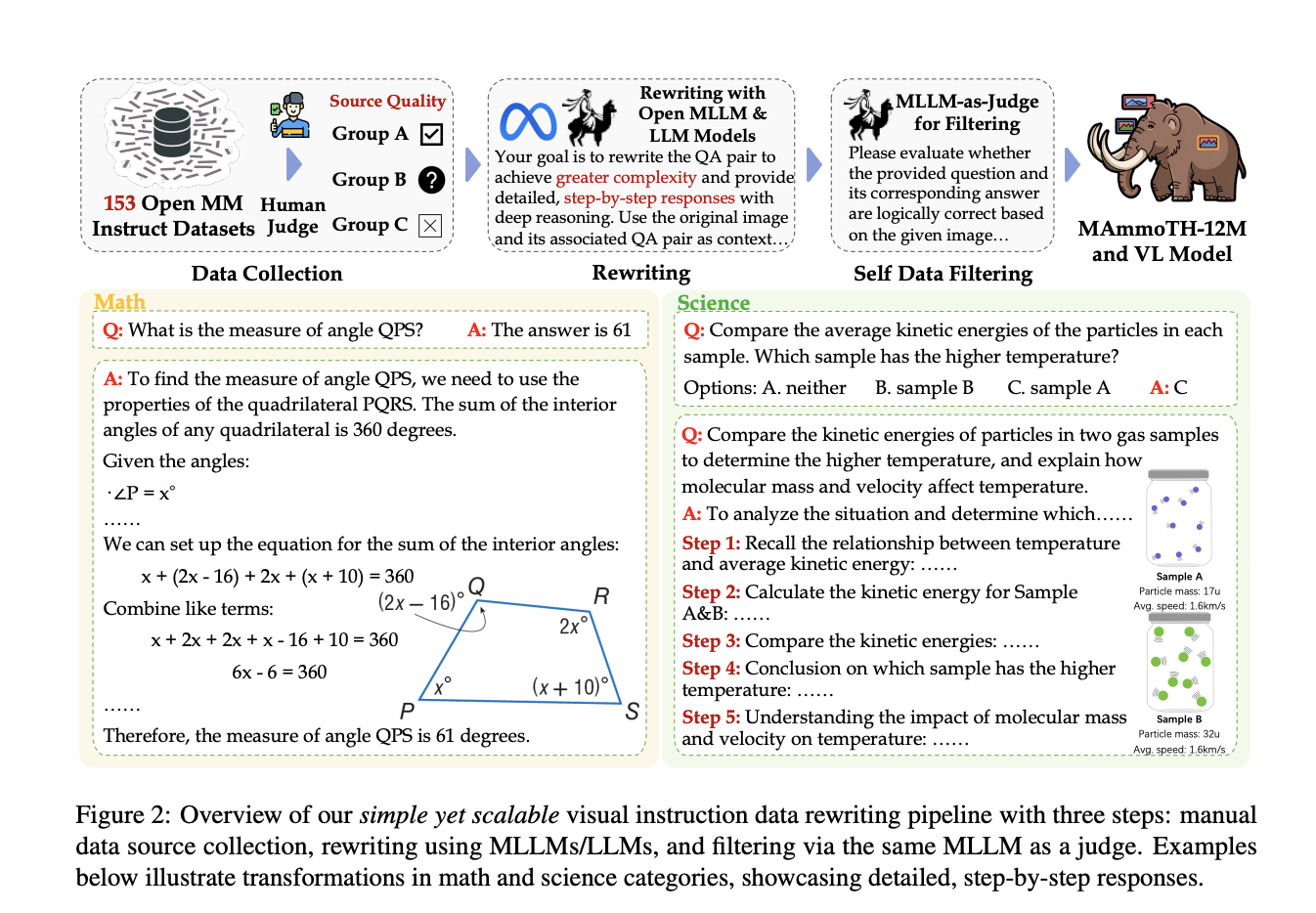
Open-Source MLLMs: Enhancing Reasoning with Practical Solutions
Open-source Multimodal Large Language Models (MLLMs) show great potential for tackling various tasks by combining visual encoders and language models. However, there is room for improvement in their reasoning abilities, primarily due to the reliance on instruction-tuning datasets that are often simplistic and academic in nature. A method called Chain of Thought (CoT) reasoning can enhance these models but requires creating detailed datasets that demonstrate step-by-step reasoning.
Challenges in Dataset Creation
Creating comprehensive datasets is both costly and challenging, especially when relying on expensive proprietary tools. To overcome this, recent efforts aim to build multimodal datasets using only open-source resources. This includes strategies like data augmentation and strict quality filtering.
Innovative Solutions in Dataset Construction
Researchers from universities like Carnegie Mellon and Nanyang Technological University developed a scalable method to create a multimodal instruction-tuning dataset. This dataset includes 12 million entries focused on complex reasoning tasks such as math problem-solving and optical character recognition (OCR).
Three-Step Dataset Creation Process
The dataset is generated through a three-step process:
- Task Categorization: Collecting diverse open-source data.
- Task Augmentation: Rewriting tasks with detailed rationales using open models.
- Quality Filtering: Ensuring data accuracy and removing errors.
Improving Performance
The newly created MAmmoTH-VL-Instruct dataset has shown state-of-the-art performance improvements across various benchmarks. The model displayed significant enhancements in reasoning tasks as well as non-reasoning tasks.
Proven Quality and Effectiveness
Quality evaluation using the InternVL2-Llama3-76B model indicated that the augmented dataset was superior in terms of relevance and information content. Advanced filtering techniques also enhanced training outcomes, especially for visually complex tasks.
Conclusion: Democratizing AI Development
This study outlines an effective approach to boost MLLMs by creating diverse, high-quality training datasets that reflect complex real-world scenarios. The MAmmoTH-VL-Instruct dataset is central to achieving superior performance across various challenges, reducing dependency on expensive proprietary systems.
For businesses looking to evolve with AI, consider these steps:
- Identify Automation Opportunities: Find key customer interaction points that could benefit from AI.
- Define KPIs: Ensure measurable impacts from your AI initiatives.
- Select an AI Solution: Pick tools that meet your requirements and allow customization.
- Implement Gradually: Start with pilot projects, gather data, and expand wisely.
For more insights on leveraging AI, connect with us via hello@itinai.com and follow us on Twitter, Telegram, and LinkedIn.
Check out the research paper for more details. All credit goes to the researchers behind this project.