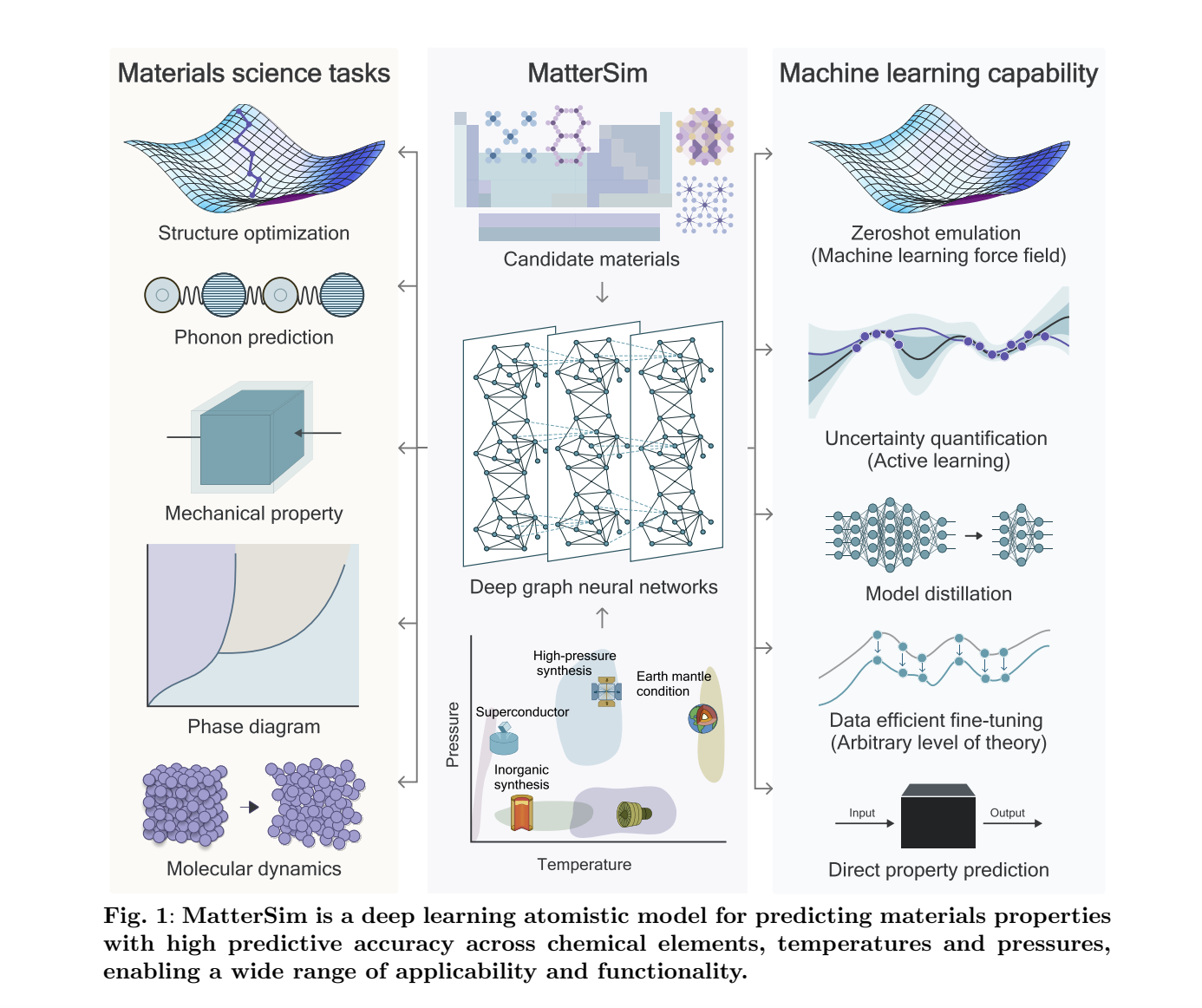
Microsoft’s MatterSim Models: A Game Changer in Materials Science
Overview of MatterSim Models
Microsoft has introduced **MatterSimV1-1M** and **MatterSimV1-5M** on GitHub. These advanced models use deep learning to simulate materials with high accuracy, making them invaluable for researchers in materials science. They can predict material properties under a wide range of conditions, such as extreme temperatures (up to 5000 K) and pressures (up to 1000 GPa).
Key Benefits
– **Fast and Accurate Predictions**: MatterSim models significantly speed up material property predictions, offering accuracy levels comparable to traditional methods but at a fraction of the time and cost.
– **Reduced Experimental Costs**: By providing an in silico alternative, these models minimize the need for expensive and time-consuming experimental methods.
– **Wide Application Range**: They can be used for various applications, including materials design, thermodynamics, and molecular dynamics simulations.
Advanced Capabilities
MatterSim models accurately forecast properties like Gibbs free energy, mechanical behavior, and phase transitions, achieving a remarkable ten-fold improvement in precision. They utilize cutting-edge deep graph neural networks and active learning to enhance their predictive capabilities continuously.
Practical Applications
Here are some of the key applications of MatterSim models:
– **Materials Design**: Discover and refine materials according to specific requirements.
– **Thermodynamic Analysis**: Compute crucial properties like Gibbs free energies and phase diagrams for stability assessment.
– **Mechanical Insights**: Predict mechanical properties critical for engineering applications.
– **Phonon and Molecular Dynamics**: Simulate lattice vibrations and molecular interactions under varying conditions.
Customization and Scalability
These models can be tailored using specific data, reducing data needs by up to **97%**. For instance, fine-tuning for simulating water only requires a small fraction of data compared to traditional methods.
Unmatched Performance
MatterSim models outperform existing universal force fields in accuracy. With a robust training background, they maintain over **90%** success rates even under extreme conditions, proving their reliability in real-world applications.
Conclusion
MatterSimV1-1M and MatterSimV1-5M combine the power of machine learning with precise simulations to accelerate research in materials science. These models are accessible on GitHub, enabling researchers to drive innovation in material discovery and design.
Get Involved
Explore the models on GitHub and stay connected with us on Twitter, Telegram, and LinkedIn for the latest updates. Join our community of over 60k on ML SubReddit and subscribe to our newsletter to stay informed.
Leverage AI for Business Growth
To evolve your company with AI:
– **Identify Automation Opportunities**: Find areas in customer interactions that could benefit from AI.
– **Set Measurable KPIs**: Ensure your AI solutions impact business outcomes.
– **Choose the Right Tools**: Select AI solutions that meet your needs and allow for customization.
– **Implement Gradually**: Start with small projects, collect data, and expand thoughtfully.
For advice on AI KPI management, contact us at hello@itinai.com and follow us for ongoing insights at t.me/itinainews or @itinaicom. Discover how AI can enhance your sales and customer engagement processes at itinai.com.