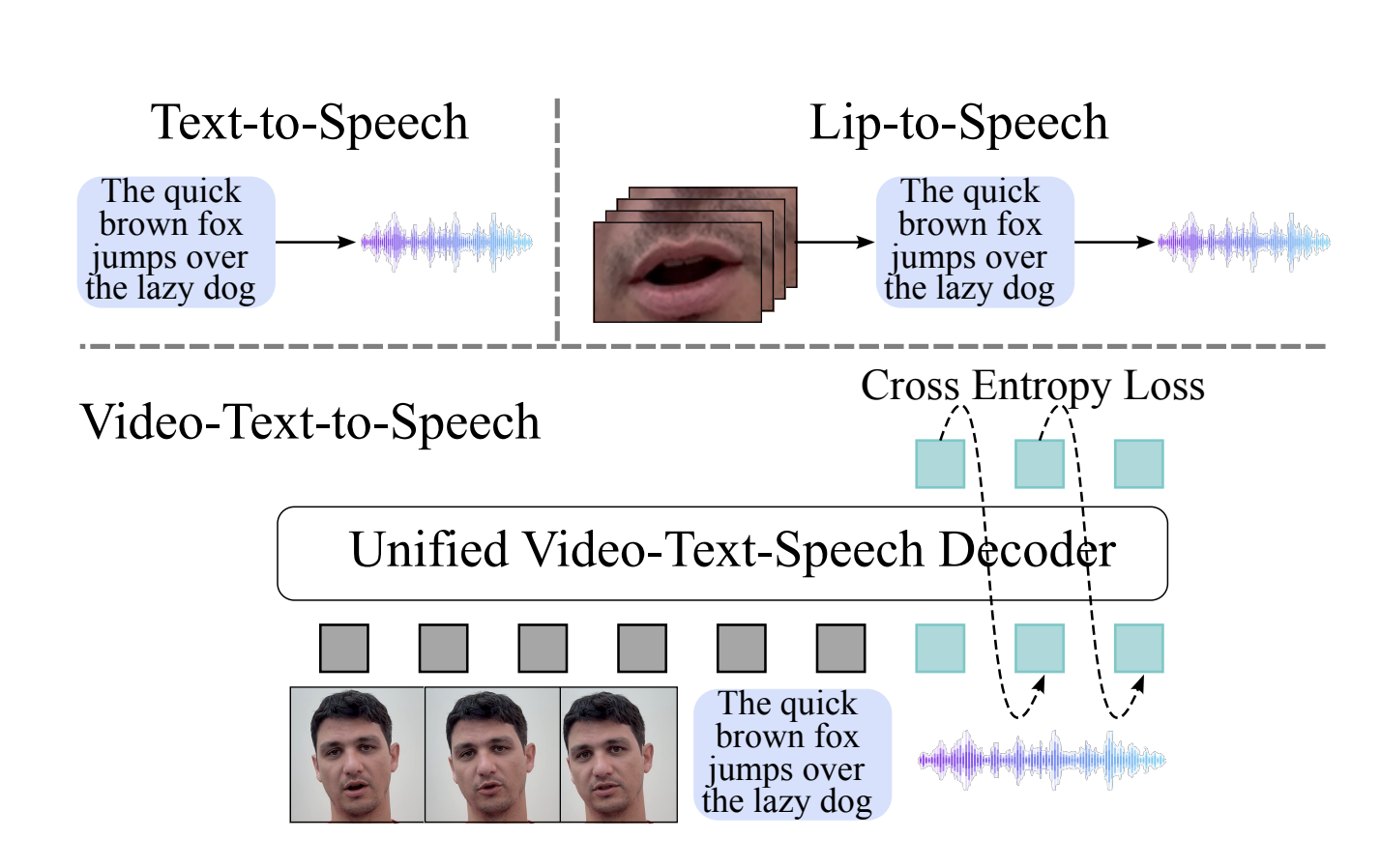
Transforming Speech Synthesis with Visatronic
Speech synthesis is evolving to create more natural audio outputs by combining text, video, and audio data. This approach enhances human-like communication. Recent advancements in machine learning, especially with transformer models, have led to exciting applications like cross-lingual dubbing and personalized voice synthesis.
Challenges in Current Methods
One major challenge is aligning speech with visual and textual cues. Traditional methods, such as lip-based speech generation and text-to-speech (TTS) models, often struggle with synchronization and naturalness, especially in multilingual or complex visual contexts. This limits their effectiveness in real-world applications that require high fidelity and understanding.
Limitations of Existing Tools
Current tools often rely on single-modality inputs or complex systems for combining different types of data. For instance, lip-detection models crop videos, while text systems focus only on language features. These methods frequently fail to capture the broader dynamics needed for natural speech synthesis.
Introducing Visatronic
Researchers from Apple and the University of Guelph have developed Visatronic, a new multimodal transformer model. This model processes video, text, and speech data together, eliminating the need for lip-detection pre-processing. This streamlined approach generates speech that aligns well with both textual and visual inputs.
How Visatronic Works
Visatronic uses a unique method to handle different types of data. It encodes video into discrete tokens and converts speech into mel-spectrograms. Text is tokenized at the character level, enhancing its understanding. All these inputs are integrated into a single transformer model that allows for interaction through self-attention mechanisms. The model also synchronizes data streams of different resolutions, ensuring coherence across inputs.
Performance and Efficiency
Visatronic has shown impressive results on challenging datasets. For example, it achieved a Word Error Rate (WER) of 12.2% on the VoxCeleb2 dataset, outperforming previous models. It also scored 4.5% WER on the LRS3 dataset without extra training. In subjective evaluations, Visatronic was rated higher for intelligibility, naturalness, and synchronization compared to traditional TTS systems.
Benefits of Video Integration
Incorporating video not only enhances content generation but also reduces training time. Visatronic models performed comparably or better after two million training steps, while text-only models required three million. This efficiency demonstrates the value of combining modalities for improved precision and alignment.
Conclusion
Visatronic is a significant advancement in multimodal speech synthesis, tackling the challenges of naturalness and synchronization. Its unified architecture integrates video, text, and audio data, offering superior performance across various conditions. This innovation sets a new benchmark for applications like video dubbing and accessible communication technologies.
For more insights, check out the Paper. Follow us on Twitter, join our Telegram Channel, and connect with our LinkedIn Group. If you appreciate our work, subscribe to our newsletter and join our 55k+ ML SubReddit.
Explore AI Solutions for Your Business
Stay competitive by leveraging Visatronic for your company. Here’s how AI can transform your operations:
- Identify Automation Opportunities: Find key customer interaction points that can benefit from AI.
- Define KPIs: Ensure your AI efforts have measurable impacts on business outcomes.
- Select an AI Solution: Choose tools that fit your needs and allow for customization.
- Implement Gradually: Start with a pilot project, gather data, and expand AI usage wisely.
For AI KPI management advice, contact us at hello@itinai.com. For ongoing insights into leveraging AI, follow us on Telegram or Twitter.