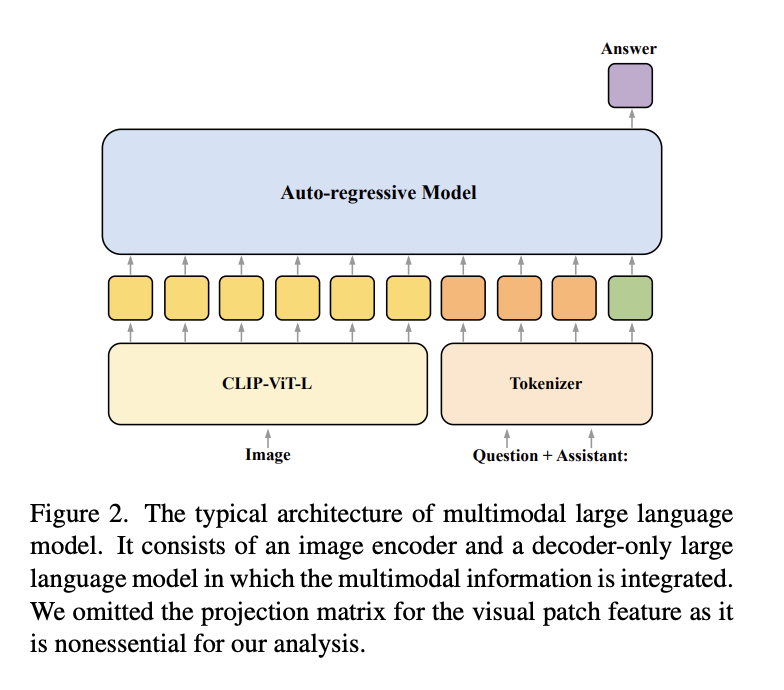
Understanding Multimodal Large Language Models (MLLMs)
MLLMs combine advanced language models with visual understanding to perform tasks that involve both text and images. They generate responses based on visual and text inputs, but we still need to understand how they function internally. This lack of understanding affects their clarity and limits the development of better models.
Research Insights
Previous studies have explored how MLLMs work by examining their internal processes and how they relate to their outputs. Key focuses included:
- Information storage within the model
- Identification of unwanted content
- Recognition and alteration of visual information
- Application of safety mechanisms
- Reduction of unnecessary visual tokens
Despite these efforts, current models still face challenges in accurately combining visual and linguistic information.
Proposed Solutions
Researchers from the University of Amsterdam and the Technical University of Munich have suggested a method to analyze how MLLMs integrate visual and linguistic information. They focused on:
- Auto-regressive models with an image encoder and a language decoder
- Interactions during visual question answering (VQA)
By using a technique called attention knockout, they tested how blocking connections between visual and linguistic inputs influenced predictions in MLLMs like LLaVA-1.5-7b and LLaVA-v1.6-Vicuna-7b.
Findings and Impact
The study utilized data from the GQA dataset and focused on different question types. Key findings include:
- The question information significantly impacts predictions, while image information has an indirect role.
- Information integration occurs in two stages, with important changes in the early and later model layers.
These insights improve the transparency of MLLMs and suggest new directions for research, ultimately leading to better model designs.
Take Action with AI
To enhance your company with AI:
- Identify Automation Opportunities: Find customer interaction points to improve with AI.
- Define KPIs: Ensure AI projects have measurable impacts.
- Select an AI Solution: Choose tools that fit your needs and allow for customization.
- Implement Gradually: Start small, collect data, and expand carefully.
For AI KPI management advice, contact us at hello@itinai.com. Stay updated on AI insights through our Telegram or follow us on @itinaicom.
Discover how AI can transform your sales and customer engagement strategies at itinai.com.