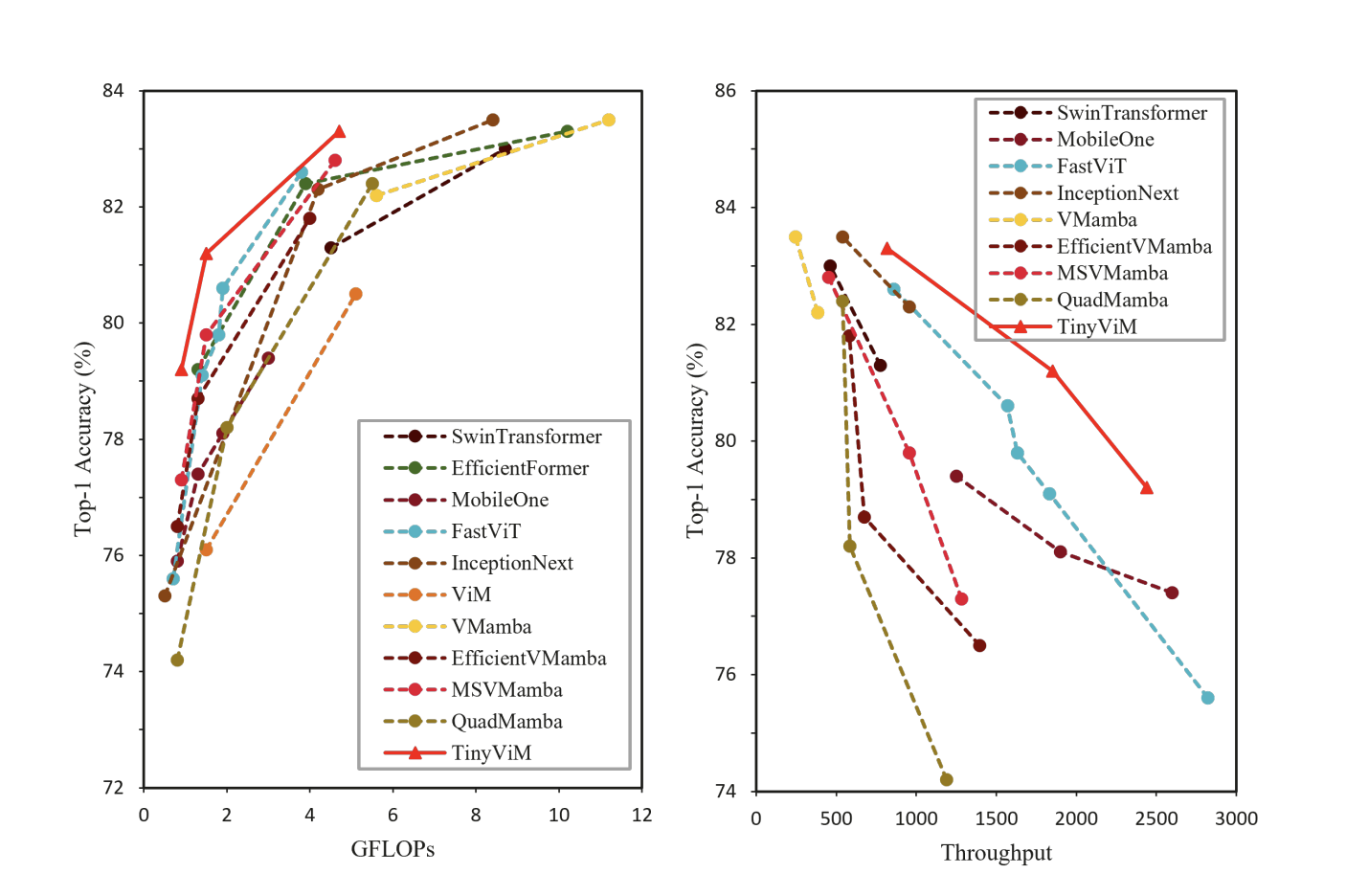
Understanding Computer Vision
Computer vision allows machines to understand and analyze visual data. This technology is crucial for various fields, including self-driving cars, medical diagnostics, and industrial automation. Researchers are working to improve how computers process complex images, using advanced techniques like neural networks to manage detailed visual tasks efficiently.
Challenges in Lightweight Models
A major challenge in creating lightweight computer vision models is capturing important details in environments with limited resources. Current technologies, such as Convolutional Neural Networks (CNNs) and Transformers, have their drawbacks. While CNNs are good at local detail extraction, they struggle with global context. On the other hand, Transformers can handle global features but are often too complex and costly to use effectively.
Innovative Solutions
To tackle these issues, new approaches have emerged. For example, MobileNet introduced efficient separable convolutions, and hybrid models like EfficientFormer combine CNNs with Transformers for better global attention. However, many of these models still miss out on capturing high-frequency details necessary for precise visual tasks.
Introducing TinyViM
Researchers from Huawei Noah’s Ark Lab have developed TinyViM, a hybrid model that combines Convolution and Mamba blocks. This model is designed to improve efficiency and feature representation by separating low and high-frequency components. The innovative Laplace mixer allows TinyViM to effectively process these features, enhancing its overall performance.
Efficiency and Performance
TinyViM uses a frequency ramp inception strategy to optimize resource allocation throughout its architecture. This means it focuses on local details early on and shifts to global context in deeper layers, ensuring the best feature representation at every stage. Additionally, its mobile-friendly design makes it suitable for real-time applications.
Proven Results
TinyViM has shown impressive results across various benchmarks. In image classification, it achieved a top-1 accuracy of 79.2% on the ImageNet-1K dataset, outperforming competitors. In object detection and segmentation tasks, TinyViM demonstrated significant improvements, showcasing its advanced feature extraction capabilities.
Lightweight and Scalable
The lightweight nature of TinyViM allows it to maintain high throughput without sacrificing accuracy. Its models, such as TinyViM-B, achieved an accuracy of 81.2% on ImageNet-1K, surpassing several other models. This scalability makes TinyViM versatile across different tasks.
Conclusion
TinyViM represents a significant advancement in lightweight vision models, effectively addressing previous limitations. By integrating innovative techniques, it achieves a balance between high-frequency detail and low-frequency context, making it a valuable tool for real-time applications.
Stay Connected
Check out the Paper for more details. Follow us on Twitter, join our Telegram Channel, and connect with our LinkedIn Group. If you’re interested in our work, subscribe to our newsletter and join our 55k+ ML SubReddit.
Explore AI Solutions
To enhance your business with AI, consider the following steps:
- Identify Automation Opportunities: Find areas in customer interactions that can benefit from AI.
- Define KPIs: Make sure your AI efforts have measurable impacts.
- Select an AI Solution: Choose tools that fit your needs and allow for customization.
- Implement Gradually: Start small, gather data, and expand your AI usage wisely.
For AI KPI management advice, contact us at hello@itinai.com. For ongoing insights, follow us on Telegram or Twitter.
Transform Your Sales and Customer Engagement
Discover how AI can reshape your sales processes and enhance customer engagement. Explore solutions at itinai.com.