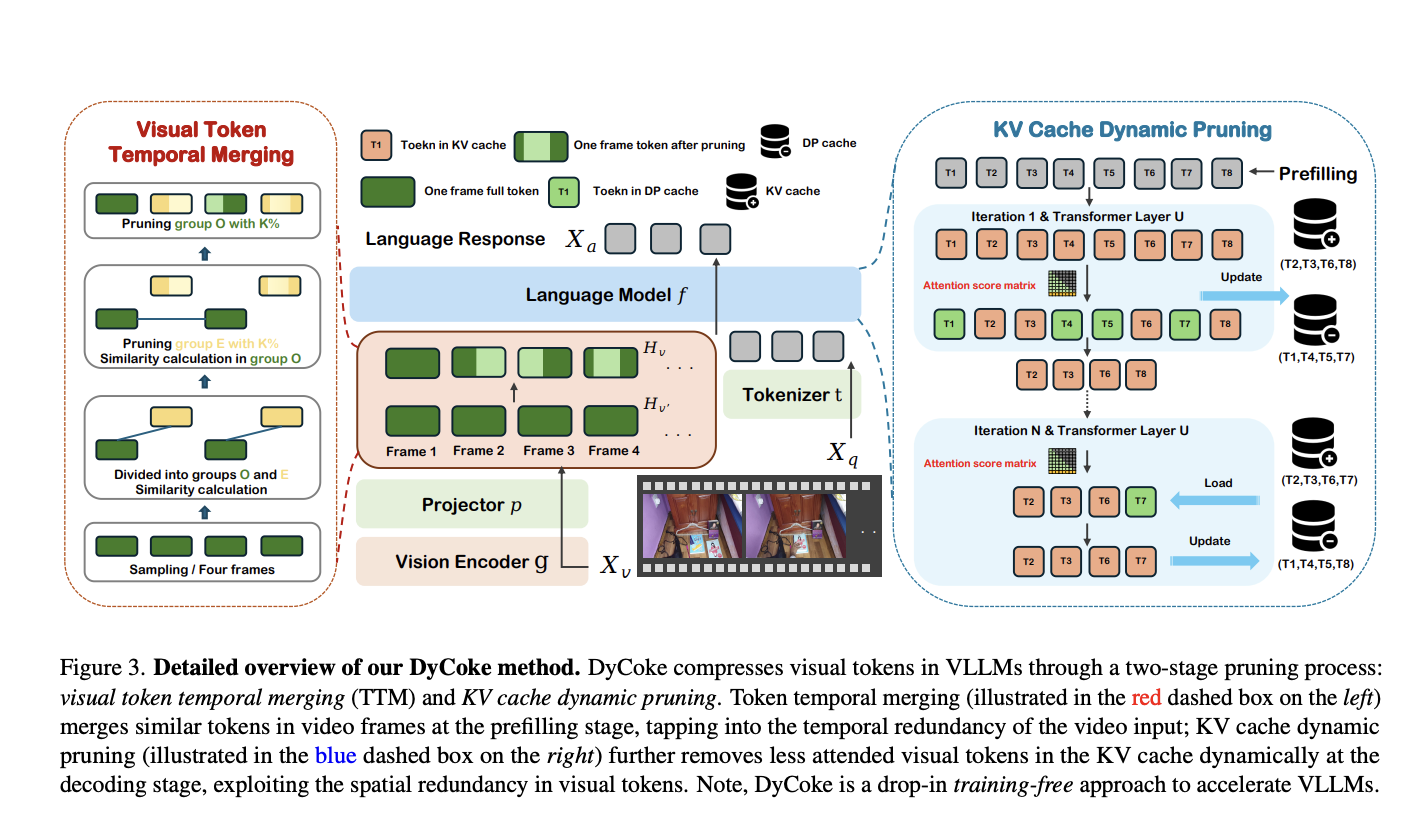
Transformative Video Language Models (VLLMs)
Video large language models (VLLMs) are game-changers for analyzing video content. They combine visual and textual information to understand complex video scenarios. Their uses include:
- Answering questions about videos
- Summarizing video content
- Describing videos in detail
These models can handle large amounts of data and produce detailed results, making them essential for tasks that require deep understanding of visual elements.
Challenges with VLLMs
A major challenge is the high computational cost involved in processing extensive video data. Videos often have many redundant frames, which can lead to:
- High memory usage
- Slower processing speeds
Improving efficiency without losing the ability to perform complex reasoning is critical.
Current Solutions
Existing methods have tried to reduce computational demands using techniques like token pruning and developing lighter models. However, these often:
- Remove important tokens needed for accuracy
- Limit the model’s reasoning capabilities
Introducing DyCoke
Researchers from various universities have created DyCoke, a new method that dynamically compresses tokens in VLLMs. Key features include:
- Training-free approach: It doesn’t require extra training or fine-tuning.
- Dynamic pruning: Adjusts which tokens to keep based on their importance.
How DyCoke Works
DyCoke uses a two-stage process for token compression:
- Temporal token merging: Combines redundant tokens from adjacent video frames.
- Dynamic pruning: Evaluates tokens during processing to retain only the most important ones.
This ensures efficient processing while keeping critical information intact.
Results and Benefits
DyCoke has shown impressive results:
- Up to 1.5× speed increase in processing time
- Memory usage reduced by 1.4×
- Maintained high accuracy even with fewer tokens
It’s effective for long video sequences and outperformed other methods in various tasks.
Accessibility and Impact
DyCoke simplifies video reasoning tasks and balances performance with resource use. It is easy to implement and doesn’t require extensive training. This advancement allows VLLMs to perform efficiently in real-world applications with limited computing resources.
Stay Connected
For more information, check out the research paper and GitHub page. Follow us on Twitter, join our Telegram Channel, and connect on LinkedIn. If you appreciate our work, subscribe to our newsletter and join our community of 55k+ on ML SubReddit.
Take Action with AI
To keep your business competitive with AI:
- Identify Automation Opportunities: Find customer interaction points that can benefit from AI.
- Define KPIs: Ensure measurable impacts on business outcomes.
- Select an AI Solution: Choose tools that fit your needs.
- Implement Gradually: Start small, gather data, then expand.
For AI management advice, reach out at hello@itinai.com. Stay tuned for insights on Telegram or Twitter.