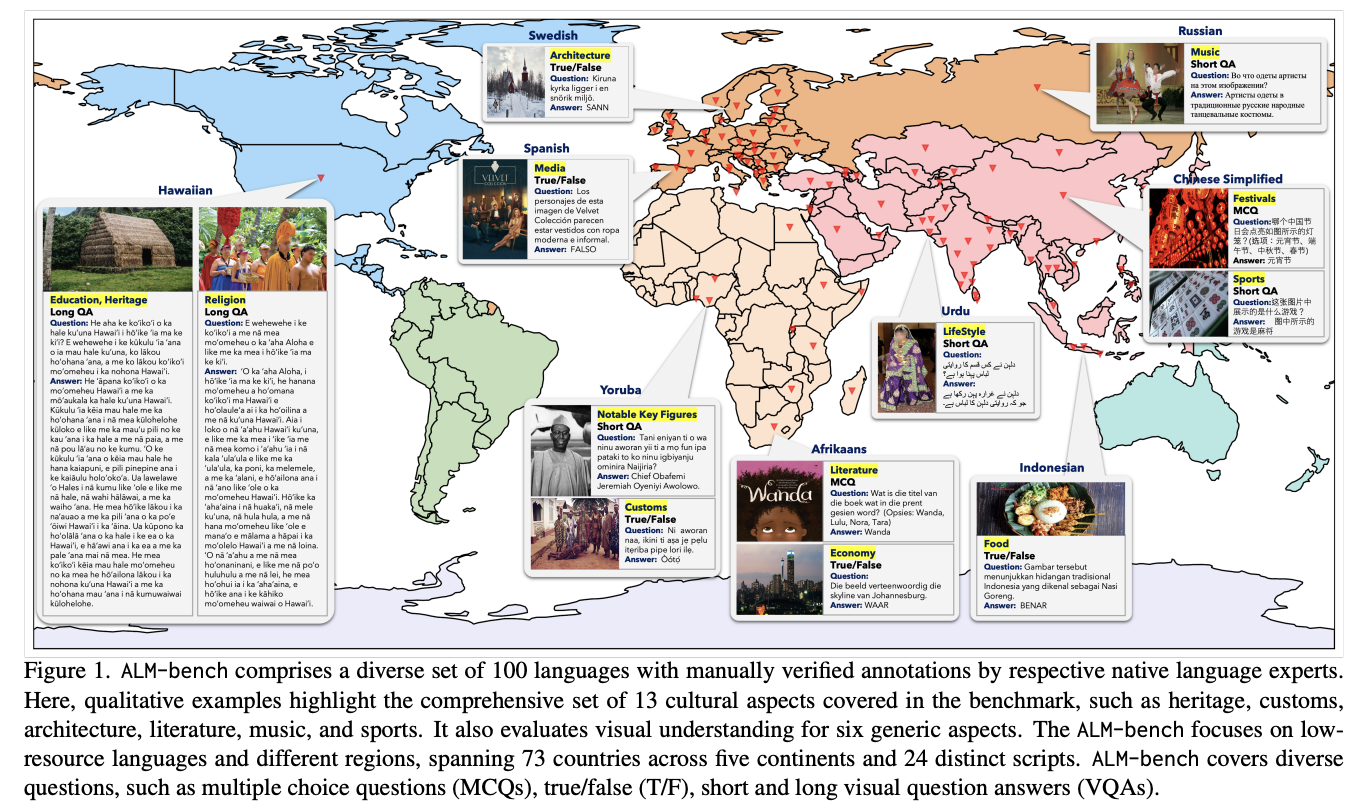
Understanding Multimodal Language Models (LMMs)
Multimodal language models (LMMs) combine language processing with visual data interpretation. They can be used for:
- Multilingual virtual assistants
- Cross-cultural information retrieval
- Content understanding
This technology improves access to digital tools, especially in diverse linguistic and visual environments.
Challenges with LMMs
Despite their potential, LMMs face significant challenges:
- Performance Gaps: They often struggle with low-resource languages like Amharic and Sinhala.
- Cultural Representation: Many models lack understanding of cultural nuances and specific traditions.
These issues limit their effectiveness for global users.
The Need for Better Evaluation
Current benchmarks for LMMs, such as CulturalVQA and Henna, are limited in scope. They focus mainly on high-resource languages and do not adequately assess cultural diversity.
Introducing ALM-bench
To tackle these challenges, researchers have developed the All Languages Matter Benchmark (ALM-bench). This benchmark:
- Evaluates LMMs across 100 languages from 73 countries
- Covers 24 scripts and 19 cultural domains
Robust Methodology
ALM-bench uses a rigorous approach with:
- Over 22,763 verified question-answer pairs
- Various question formats including multiple-choice and visual questions
This ensures a comprehensive evaluation of language models.
Insights from Evaluation
Evaluation results showed:
- Proprietary models like GPT-4o performed better than open-source models.
- Performance dropped significantly for low-resource languages.
- Best results were in education and heritage domains, but weaker in customs and notable figures.
Key Takeaways
- Cultural Inclusivity: ALM-bench sets a new standard for diverse language evaluation.
- Robust Evaluation: It tests models on complex linguistic and cultural contexts.
- Performance Gaps: Highlights the need for more inclusive model training.
- Model Limitations: Even top models struggle with cultural reasoning.
Conclusion
The ALM-bench research identifies limitations in current LMMs and provides a framework for improvement. By covering a wide range of languages and cultural contexts, it aims to enhance inclusivity and effectiveness in AI technology.
Get Involved
For more information, check out the Paper and Project. Follow us on Twitter, join our Telegram Channel, and connect on LinkedIn. If you enjoy our work, subscribe to our newsletter and join our 55k+ ML SubReddit.
Transform Your Business with AI
Stay competitive and leverage the All Languages Matter Benchmark (ALM-bench) to enhance your AI capabilities:
- Identify Automation Opportunities: Find key areas for AI integration.
- Define KPIs: Measure the impact of AI on business outcomes.
- Select an AI Solution: Choose tools that fit your needs.
- Implement Gradually: Start small, gather data, and expand.
For AI management advice, contact us at hello@itinai.com. Stay updated on AI insights via our Telegram or Twitter.