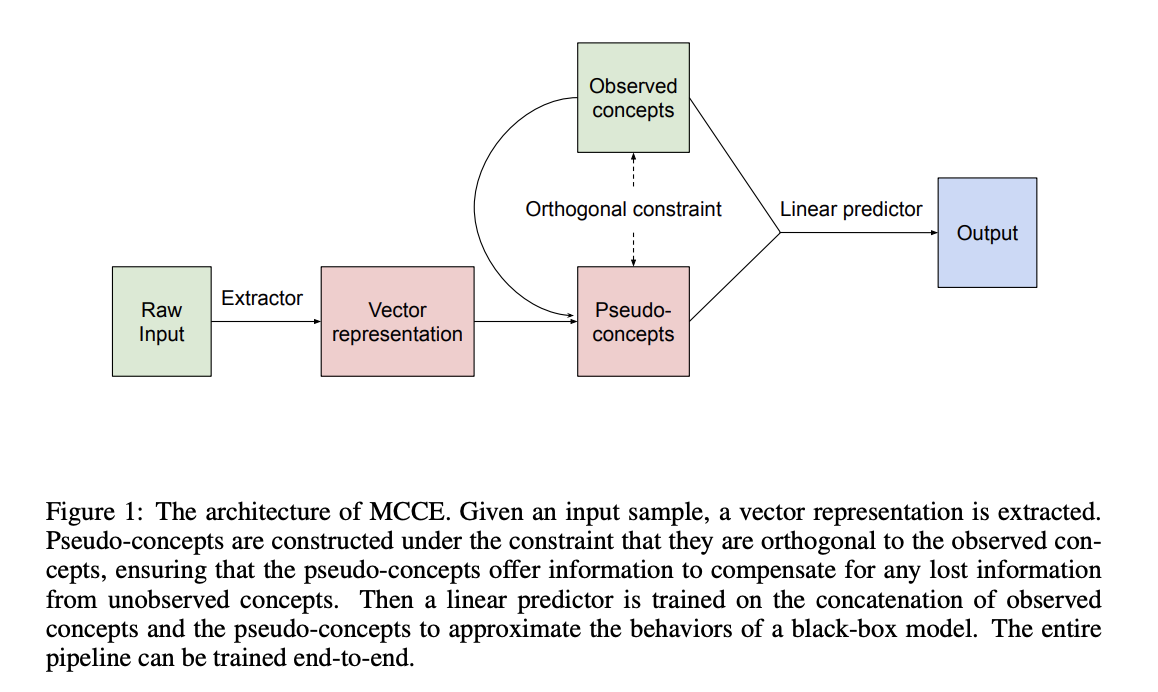
Understanding Machine Learning with Concept-Based Explanations
Machine learning can be explained more intuitively by using concept-based methods. These methods help us understand how models make decisions by connecting them to concepts we can easily grasp. Unlike traditional methods that focus on low-level features, concept-based approaches look at high-level features and extract meaningful information from them. This allows us to see how the model thinks.
Practical Solutions and Their Value
Concept-based methods assess their effectiveness by estimating causal effects. This involves changing specific concepts and observing how these changes affect the model’s predictions. While this method is gaining traction, it has limitations. Current methods assume that all concepts in the dataset are fully observed, which is often not the case.
New Research from the University of Wisconsin-Madison
Researchers have introduced a framework called the “Missingness-aware Causal Concept Explainer” (MCCE) to address the issue of unobserved concepts. This framework creates pseudo-concepts that help capture the effects of concepts that are not directly observed.
How MCCE Works
MCCE uses mathematical experiments to show how unobserved concepts can skew causal explanations. It models the relationship between concepts and the model’s output using a linear function. This approach allows MCCE to explain reasoning at both the individual sample level and the overall model level.
By using raw data, MCCE compensates for missing information. It creates pseudo-concept vectors from the encoded input data and trains a linear model using both observed and pseudo-concepts.
Experimental Validation
The researchers tested MCCE on the CEBaB dataset, known for its human-verified counterfactual text. They fine-tuned three large models: base BERT, base RoBERTa, and Llama-3. The results showed that MCCE outperformed existing methods, especially when some concepts were unobserved.
Benefits of MCCE
MCCE not only provided robust performance but also served as an interpretable predictor. It matched the performance of black-box models while offering clearer insights into the model’s reasoning process.
Conclusion
This research presents a valuable solution to the challenges of causal effects in explainability. The MCCE framework can enhance the accuracy and generalizability of AI models, especially when validated with more diverse datasets.
For more details, check out the Paper here. Follow us on Twitter, join our Telegram Channel, and connect with our LinkedIn Group. If you enjoy our insights, subscribe to our newsletter and join our 55k+ ML SubReddit.
Upcoming Event
Join us for the [FREE AI VIRTUAL CONFERENCE] SmallCon on Dec 11th. Learn from AI leaders like Meta, Mistral, and Salesforce about building effective AI solutions.
Enhancing Your Business with AI
To stay competitive, consider implementing the Missingness-aware Causal Concept Explainer in your AI strategies:
- Identify Automation Opportunities: Find areas in customer interactions that can benefit from AI.
- Define KPIs: Ensure your AI projects have measurable impacts.
- Select an AI Solution: Choose tools that meet your specific needs.
- Implement Gradually: Start with a pilot project, gather data, and expand carefully.
For AI KPI management advice, contact us at hello@itinai.com. For ongoing insights, follow us on Telegram or @itinaicom.
Discover how AI can transform your sales processes and customer engagement. Explore more at itinai.com.