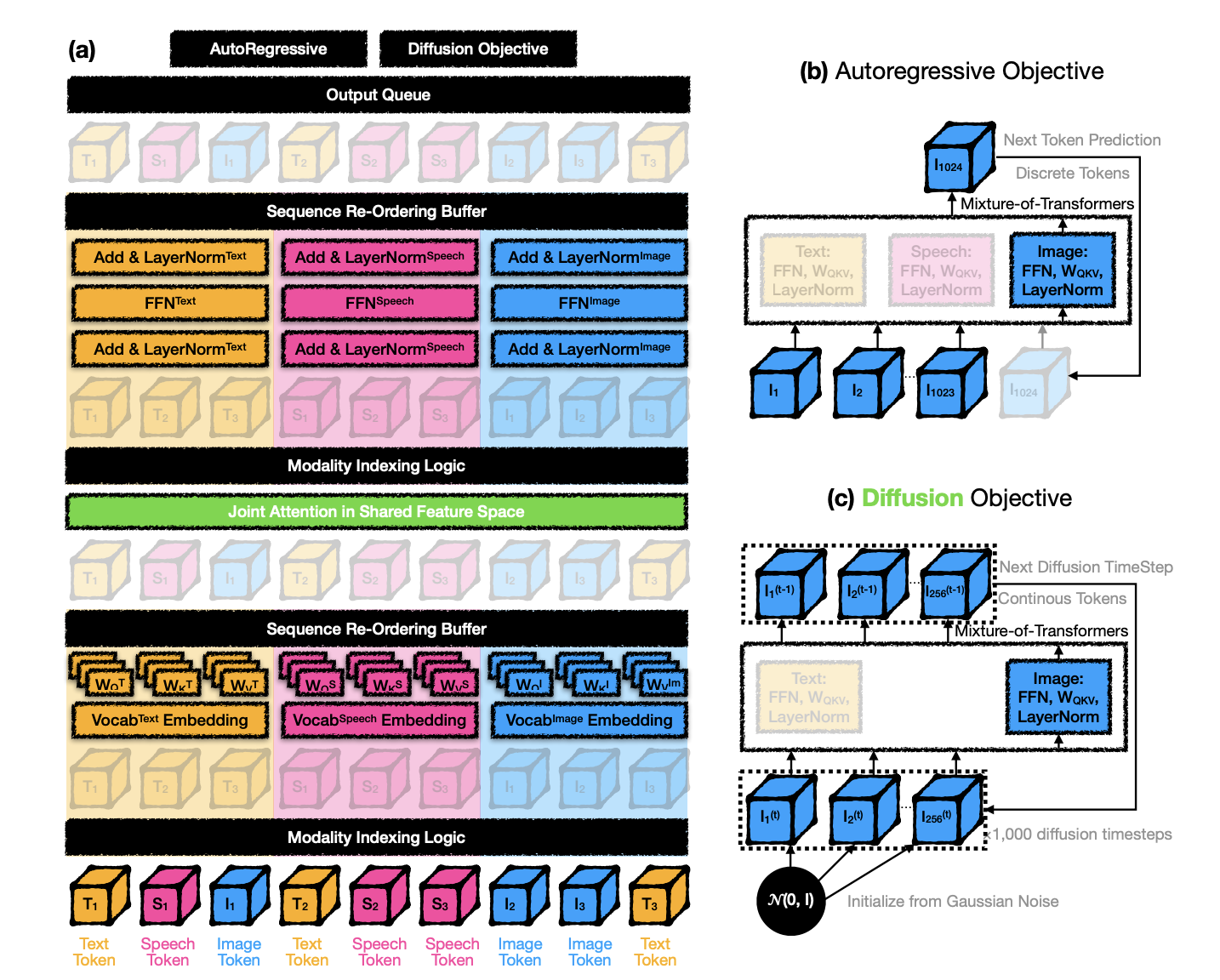
Advancements in AI: Multi-Modal Foundation Models
Recent developments in AI have led to models that can handle text, images, and speech all at once. These multi-modal models can change how we create content and translate information across different formats. However, they require a lot of computing power, making them hard to scale and use efficiently.
Challenges in Multi-Modal AI
Traditional language models are mainly designed for text. Adding images and audio increases the computational demands significantly. For example, models that work with text alone need much more data and processing power when they also handle images and speech. Researchers are looking for ways to build models that can manage these needs without needing extra resources.
Strategies for Efficiency
One effective method is using sparse architectures like Mixture-of-Experts (MoE). This approach activates only certain parts of the model when needed, which helps reduce the workload. However, MoE can face issues like instability and difficulty in managing different types of data effectively.
Introducing Mixture-of-Transformers (MoT)
Researchers from Meta and Stanford have developed a new architecture called Mixture-of-Transformers (MoT). This model is designed to be more efficient by using specific parameters for each type of data (text, image, speech). MoT allows for better optimization without needing extra components, leading to improved processing and accuracy.
Benefits of Mixture-of-Transformers
MoT separates the parameters for text, images, and speech, which enhances both training and performance. For instance, in tests, MoT achieved similar results to traditional models while using significantly less computational power. This efficiency can lead to major cost savings in large-scale AI applications.
Key Improvements with MoT
- Efficient Processing: MoT performs as well as dense models while using only 37.2% to 55.8% of the resources.
- Faster Training: MoT reduced training time for image tasks by 52.8% and text tasks by 24.4% without losing accuracy.
- Scalable Solutions: MoT effectively manages different types of data without needing extra processing layers.
- Real-Time Efficiency: MoT significantly cuts down training times, making it suitable for real-time applications.
Conclusion
Mixture-of-Transformers offers a groundbreaking approach to multi-modal AI by efficiently integrating various data types. Its sparse architecture reduces computational demands while maintaining strong performance across tasks. This innovation could reshape the AI landscape, making advanced multi-modal applications more accessible and resource-efficient.
For more insights, check out the research paper and follow us on Twitter, join our Telegram Channel, and LinkedIn Group. If you enjoy our work, subscribe to our newsletter and join our 55k+ ML SubReddit.
Upcoming Webinar
[FREE AI WEBINAR] Implementing Intelligent Document Processing with GenAI in Financial Services and Real Estate Transactions.
Transform Your Business with AI
Stay competitive by leveraging AI solutions:
- Identify Automation Opportunities: Find key areas in customer interactions that can benefit from AI.
- Define KPIs: Ensure your AI initiatives have measurable impacts on your business.
- Select an AI Solution: Choose tools that fit your needs and allow for customization.
- Implement Gradually: Start small, gather data, and expand your AI usage wisely.
For AI KPI management advice, contact us at hello@itinai.com. For ongoing insights into AI, follow us on Telegram at t.me/itinainews or Twitter @itinaicom.
Explore how AI can enhance your sales processes and customer engagement at itinai.com.