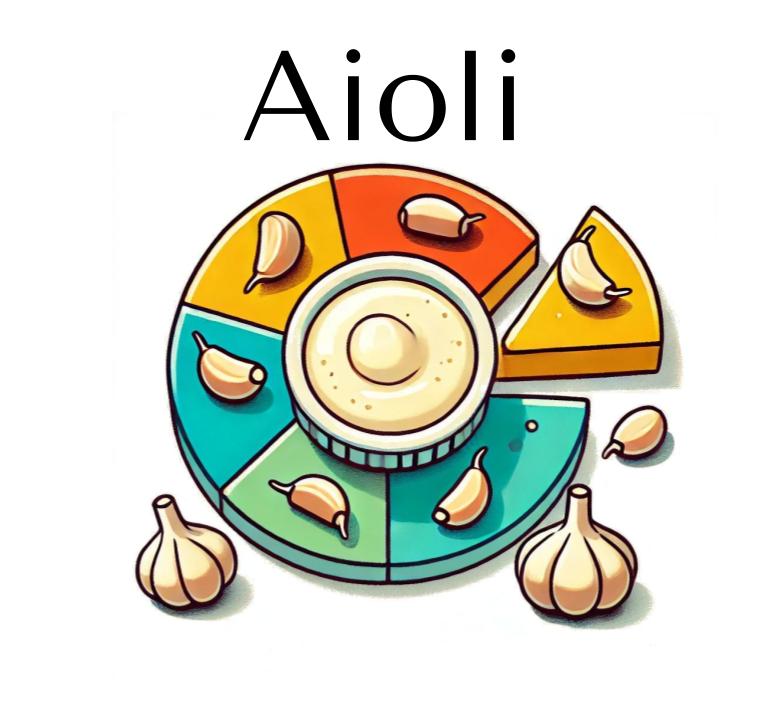
Challenges in Training Large Language Models
Training large language models like GPT-4 has a key challenge: finding the right mix of training data. These models can create various types of content, but their success depends on balancing data from different sources, such as legal documents, code, and scientific articles. Current methods for mixing this data are inconsistent and often fail to outperform basic sampling techniques, wasting resources and leading to subpar performance.
Introducing Aioli: A Better Solution for Data Mixing
To tackle these issues, researchers from Stanford, NYU, and Genentech have developed Aioli, a new online data mixing method using a framework called Linear Mixing Optimization (LMO). This approach improves how data mixtures are optimized during training. Unlike older methods that rely on static guesses, Aioli adjusts the data mix based on the model’s performance in real-time, eliminating the need for extra training runs.
How Aioli Works
Aioli treats data mixing as an optimization problem aimed at reducing the model’s average test loss. It uses an online adjustment mechanism, allowing the model to change mixture proportions dynamically at each training step. This means Aioli can adapt to the model’s needs as training progresses, leading to better results.
Proven Results
In tests across six datasets, Aioli outperformed traditional methods by improving model accuracy by an average of 0.28 in test perplexity. In more limited training scenarios, Aioli achieved up to 12.01 points of improvement, demonstrating its effectiveness.
Why Aioli Matters
Aioli is a major breakthrough for several reasons:
- Improved Understanding: It clarifies why previous methods struggled, allowing for better parameter estimation during training.
- Efficiency: Aioli saves computational resources and reduces the environmental impact of training large models.
- Faster Deployment: This efficiency means quicker updates for applications like conversational AI and search engines.
Conclusion
Aioli offers a promising solution to the challenges of data mixing in language model training. By using the LMO framework, it dynamically adjusts data mixtures in real-time, enhancing accuracy without extra computational costs. As the demand for effective language models grows, Aioli provides a significant advancement, enabling better learning from diverse data sources.
For more information, check out the Paper and GitHub. Follow us on Twitter, join our Telegram Channel, and connect with our LinkedIn Group. If you enjoy our work, subscribe to our newsletter and join our 55k+ ML SubReddit.
Upcoming Event
Join our live LinkedIn event, ‘One Platform, Multimodal Possibilities,’ featuring Encord CEO Eric Landau and Head of Product Engineering, Justin Sharps, discussing how to reinvent the data development process for building advanced multimodal AI models quickly.
Transform Your Business with AI
To stay competitive and leverage AI effectively:
- Identify Automation Opportunities: Find key areas for AI integration.
- Define KPIs: Ensure measurable impacts from your AI initiatives.
- Select an AI Solution: Choose tools that fit your needs.
- Implement Gradually: Start small, gather data, and expand wisely.
For AI KPI management advice, contact us at hello@itinai.com. For ongoing insights, follow us on Telegram or Twitter.
Discover how AI can enhance your sales processes and customer engagement at itinai.com.