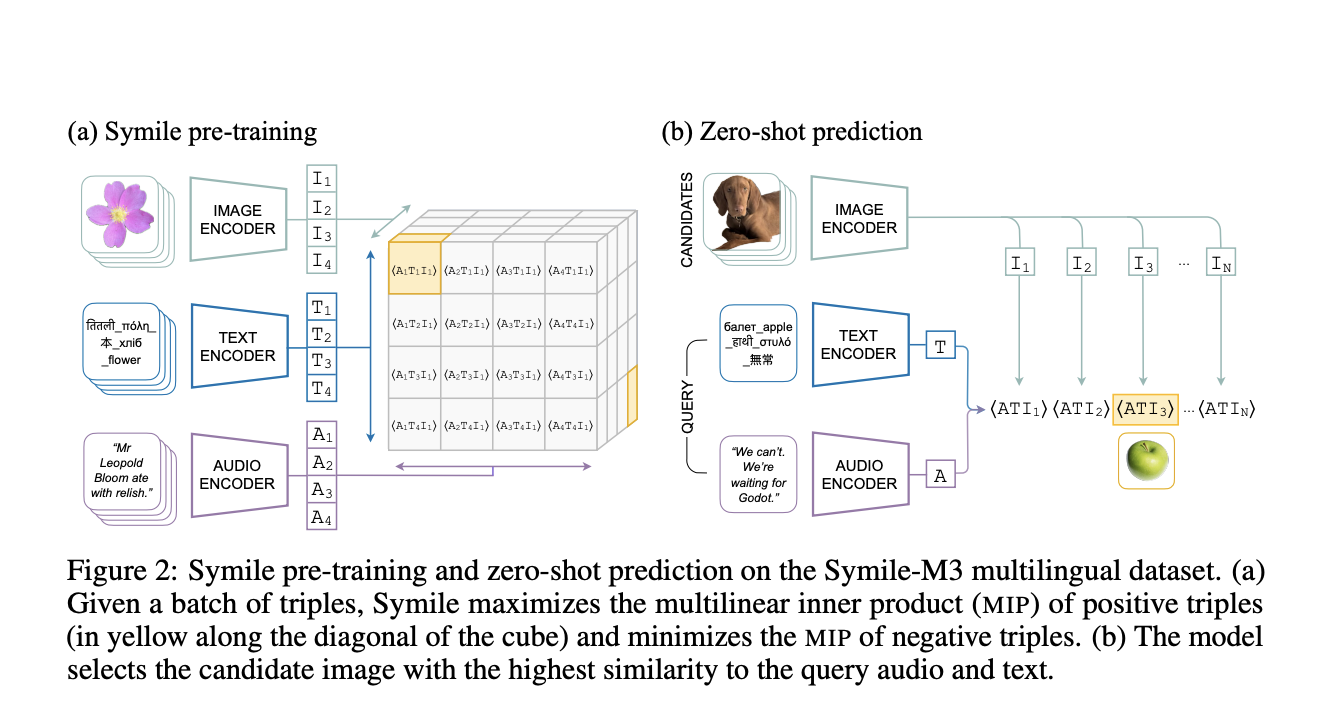
Understanding Contrastive Learning and Its Challenges
Contrastive learning is vital for creating representations from paired data, such as image-text combinations. It helps transfer knowledge to various tasks, especially in complex fields like robotics and healthcare.
Real-World Applications
In robotics, data is collected from various sensors, while healthcare combines medical images and genomic data. Both fields need models that can handle multiple data types simultaneously. Current contrastive learning models are mostly limited to two data types, which affects their representation quality.
The Limitations of Pairwise Models
Models like CLIP only analyze pairwise data, which means they miss deeper relationships among multiple data types. For example, if analyzing images, audio, and text, a pairwise model might overlook how all three interact.
Challenges Faced
Extending pairwise models to handle more data types requires complex structures and specific training steps, making them less versatile. This creates a need for models that can capture interactions among all data types in one go.
Introducing Symile: A New Solution
Researchers from New York University have developed Symile, a groundbreaking model that overcomes the limitations of traditional contrastive learning. Symile can manage multiple modalities without complicated adjustments.
Key Features of Symile
Symile uses a total correlation objective to unify various data types and estimates their dependencies. Its design allows for flexibility and adaptability, making it effective even when some data is missing.
How Symile Works
Symile employs a unique scoring function, the multilinear inner product (MIP), to assess the similarity among multiple data types. This allows it to capture richer information than standard pairwise models.
Impressive Performance
In tests, Symile outperformed traditional models significantly. For instance, it achieved 93.9% accuracy in predicting image content based on text and audio, while CLIP only reached 47.3%. Even with missing data, Symile maintained high accuracy, demonstrating its robustness.
The Value of Symile
Symile represents a major advancement in contrastive learning, particularly for healthcare and multilingual tasks. Its ability to process multiple data types simultaneously makes it a valuable tool for real-world applications.
Practical Steps for AI Integration
- Identify Automation Opportunities: Find areas where AI can enhance customer interactions.
- Define KPIs: Ensure your AI efforts have measurable impacts.
- Select AI Solutions: Choose tools that fit your needs.
- Implement Gradually: Start small, gather data, and expand wisely.
Stay Updated
For continuous insights into AI, connect with us through our newsletter, Twitter, and Telegram. Join our community to explore how AI can transform your business.
Check out the Paper and GitHub. Don’t forget to follow us on Twitter and join our Telegram Channel and LinkedIn Group.
Upcoming Event
Join our live LinkedIn event, ‘One Platform, Multimodal Possibilities,’ featuring experts discussing innovative approaches to building multimodal AI models.